filmov
tv
The biggest beef in statistics explained
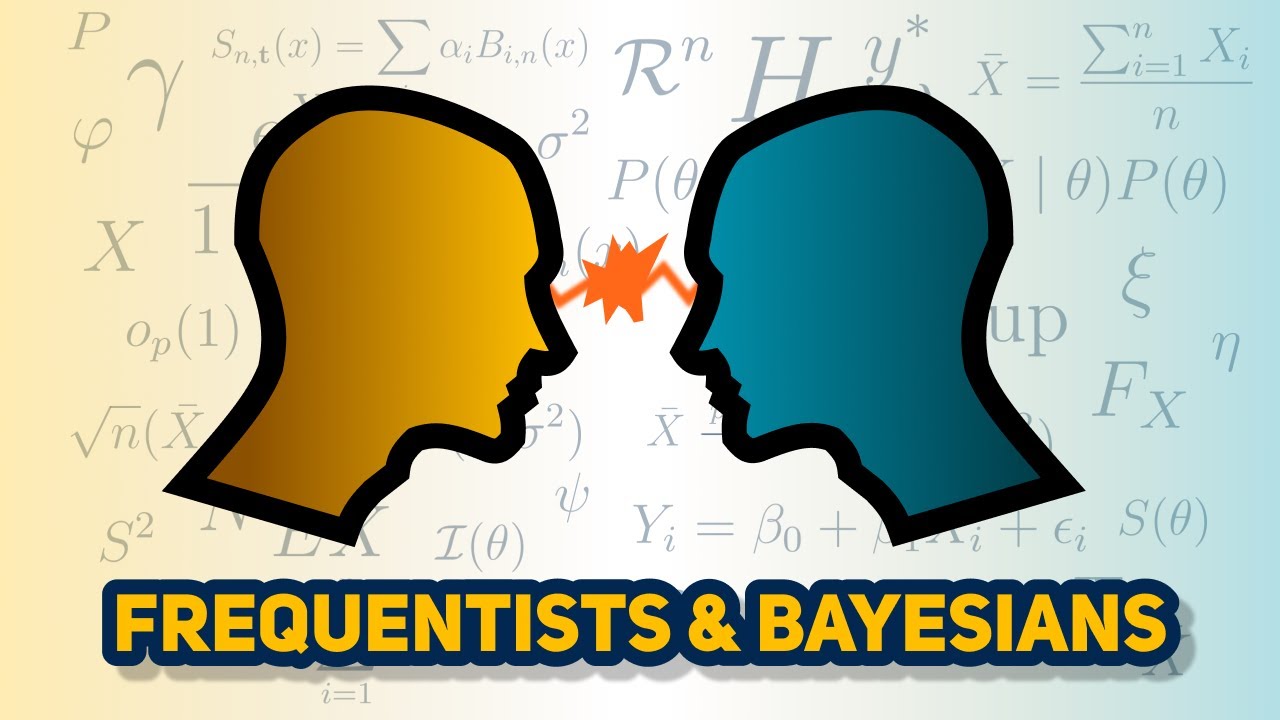
Показать описание
Non-clickbait title: Explaining the differences between frequentist and Bayesian statistics (with analyses)
🔗 Links mentioned in video:
Stay updated with the channel and some stuff I make!
This video was sponsored by Brilliant.
The biggest beef in statistics explained
Beef found in BFDI!
Top 5 Most Delicious Cuts of Beef #facts #food #ingredients
Top beef exporter countries ranking | Beef meat export
Beef Production by Countries(1960-2022)#shorts
Who are the biggest beef eaters of all?
How 4 companies control the beef industry
Why you should eat beef and fly planes less 🐮✈️ #facts #beef #plane #cow
'10 Protein-Rich Meat Dishes You Need to Try!' #facts #superfood #shorts
she’ll eat it off like beef jerky #shorts #facts
Top Nutrition Facts and Health Effects of Beef
India is the Largest Beef exporter in the World 🐄 #shorts
Diddy responds to Mase 😱#facts #truth #diddy #hiphop #beef
Top 10 Countries With The Most Beef Consumer #india #top10famous #instagram
Top 10 Cattle Beef Breeds | Highest Average Daily Gain the World from Weaning to Yearling Age
Grass Fed vs Grain Fed Beef
Where’s the Beef: Is Big Data Having a Real Impact on The Economy? (DataEDGE 2014)
Argentina: The World’s Biggest Beef Lovers! 🥩 #funfacts
How India became one of the world’s top beef exporters | India Now! | ABC News
The Biggest Mistakes You Make When Cooking With Ground Beef
Top 5 beef 🍖 producing countries in the world #shorts #beef
BEEF IS GOOD! #facts
3 Reasons Beef Is Not Bad For The Environment 🐄🚫🌱
Veggie Grill gets TAKEN OUT by big beef & dairy 😳 #fastfoodhouse #veganhumor #plantbased #comedy...
Комментарии