filmov
tv
CVPR 2019 Oral - JSIS3D: Joint Semantic-Instance Segmentation of 3D Point Clouds
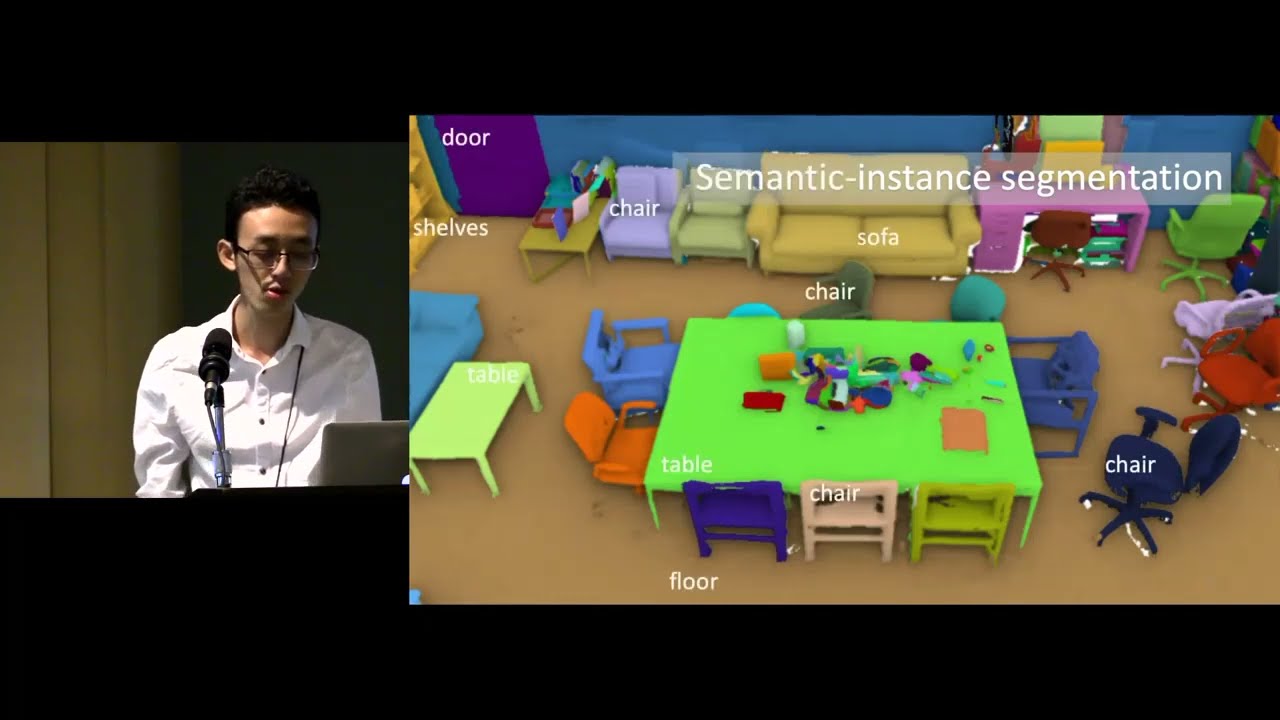
Показать описание
Deep learning techniques have become the to-go models for most vision-related tasks on 2D images. However, their power has not been fully realized on several tasks in 3D space, e.g., 3D scene understanding. In this work, we jointly address the problems of semantic and instance segmentation of 3D point clouds. Specifically, we develop a multi-task point-wise network that simultaneously performs two tasks: predicting the semantic classes of 3D points and embedding the points into high-dimensional vectors so that points of the same object instance are represented by similar embeddings. We then propose a multi-value conditional random field model to incorporate the semantic and instance labels and formulate the problem of semantic and instance segmentation as jointly optimizing labels in the field model. The proposed method is thoroughly evaluated and compared with existing methods on different indoor scene datasets including S3DIS and SceneNN. Experimental results showed the robustness of the proposed joint semantic-instance segmentation scheme over its single components. Our method also achieved state-of-the-art performance on semantic segmentation.