filmov
tv
Deep reinforcement learning for flow control on a cylinder at Re=2,000
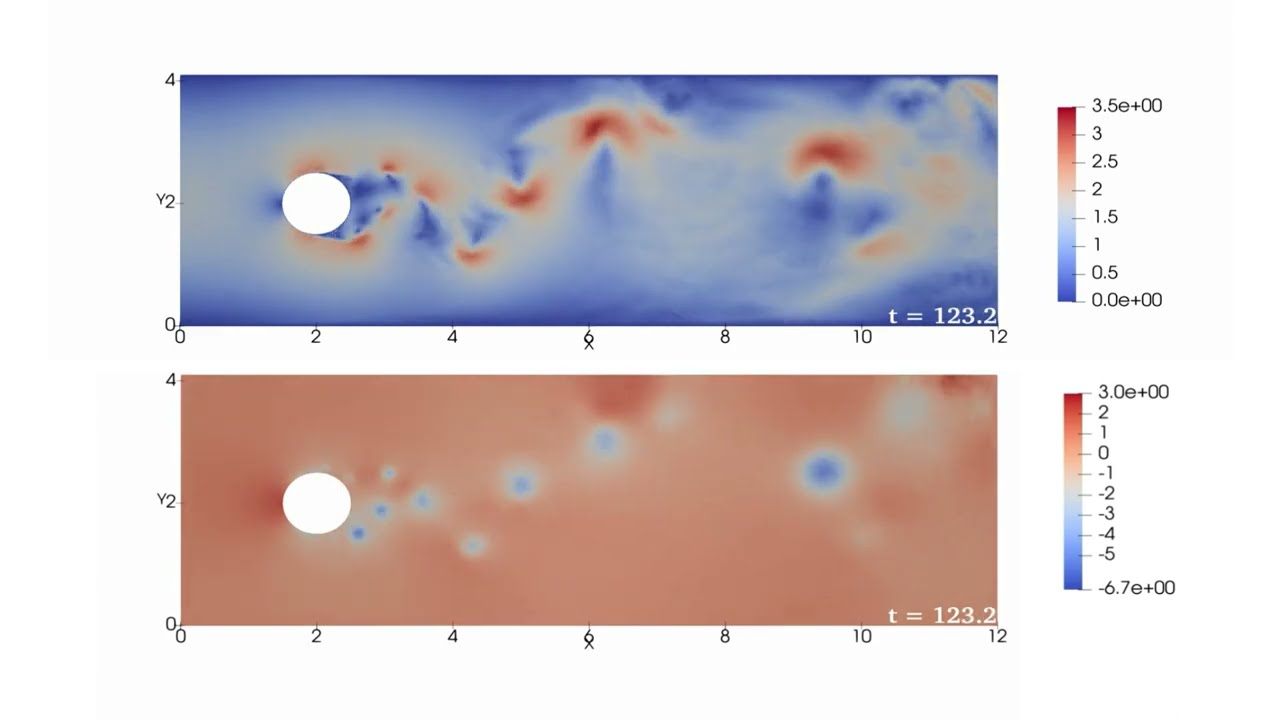
Показать описание
In this video, created by Pol Suárez, we summarize some of the results of our recent work:
We show the visualization of the (top) velocity and (bottom) pressure field of an actuated two-dimensional cylinder at Reynolds number Re=2,000. Deep neural networks trained through deep reinforcement learning (DRL), coupled with the numerical solver Alya, are used to perform active flow control (AFC). The control consists of two jets located at the highest and lowest points of the cylinder surface. The agent finds a strategy yielding 17% drag reduction. At this Reynolds number the control is very different from the ones obtained for Re=100 or 1,000. The jet actuation starts at t=50, and it can be observed how the jets clearly break the stabilized uncontrolled vortices into smaller and less-energetic ones thanks to high-frequency actuations. This control mechanism, obtained through the DRL agent, is similar to the drag-crisis phenomenon.
We show the visualization of the (top) velocity and (bottom) pressure field of an actuated two-dimensional cylinder at Reynolds number Re=2,000. Deep neural networks trained through deep reinforcement learning (DRL), coupled with the numerical solver Alya, are used to perform active flow control (AFC). The control consists of two jets located at the highest and lowest points of the cylinder surface. The agent finds a strategy yielding 17% drag reduction. At this Reynolds number the control is very different from the ones obtained for Re=100 or 1,000. The jet actuation starts at t=50, and it can be observed how the jets clearly break the stabilized uncontrolled vortices into smaller and less-energetic ones thanks to high-frequency actuations. This control mechanism, obtained through the DRL agent, is similar to the drag-crisis phenomenon.
Deep reinforcement learning for flow control on a cylinder at Re=2,000
Deep Reinforcement Learning for Fluid Dynamics and Control
Thinking While Moving: Deep Reinforcement Learning with Concurrent Control
Deep Reinforcement Learning for Goal oriented Dialogue Systems
Deep Reinforcement Learning for Multi-Agent Interaction - Stefano Albrecht
Active Flow Control via Deep Reinforcement Learning Implemented in OpenFOAM
Ricardo Vinuesa - Modelling and controlling turbulent flows through deep learning
NACA Airfoil optimization with Deep Reinforcement Learning
Deep Learning Cars
Physics-constrained deep reinforcement learning for flow field denoising
Deep reinforcement learning for flow control in aeronautics
Using AI to Navigate Flow (Peter Gunnarson Presentation APS DFD 2021)
Maxim Lapan | Deep Reinforcement Learning: theory, intuition, code
Alexandre Bayen (Berkeley) Deep Reinforcement Learning for Vehicle Control
DDPS | Modeling and controlling turbulent flows through deep learning
Deep Reinforcement Learning: Field Development Optimization | Paper Explained
AI Learns to Park - Deep Reinforcement Learning
Controlling turbulence through deep reinforcement learning
Deep reinforcement learning - Traffic Light Control System
Stable baselines 3 Reinforcement Learning using Tensor flow 2.x with PPO Algorithm
Job Shop Scheduling via Deep Reinforcement Learning: a Sequence to Sequence approach (LION17)
Deep Reinforcement Learning Based Optimization Algorithm for Permutation Flow Shop Scheduling
Offline Deep Reinforcement Learning Algorithms
Multi-agent reinforcement learning (MARL) versus single-agent RL (SARL) for flow control
Комментарии