filmov
tv
DDPS | Towards reliable, efficient, and automated model reduction of parametrized nonlinear PDEs
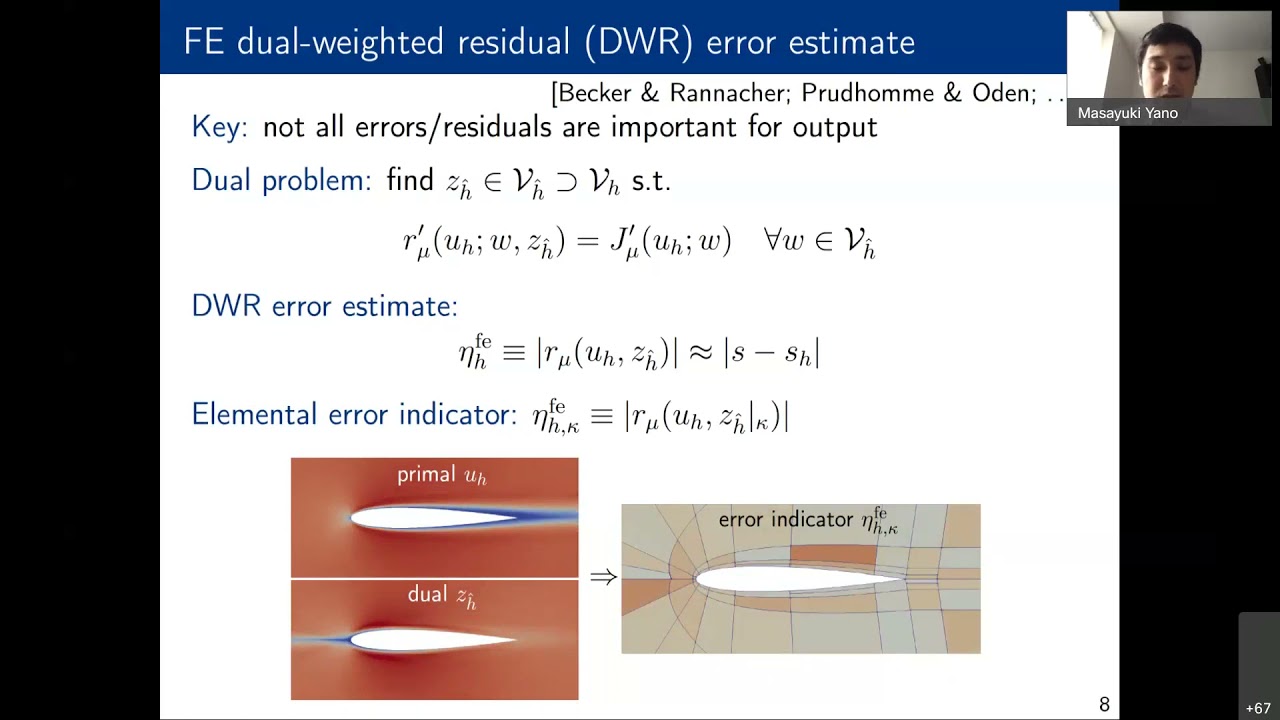
Показать описание
Description: Many engineering tasks, such as parametric study and uncertainty quantification, require rapid and reliable solution of partial differential equations (PDEs) for many different configurations. In this talk, we consider goal-oriented model reduction of parametrized nonlinear PDEs with an emphasis on aerodynamics problems. The key ingredients are as follows: the discontinuous Galerkin (DG) method, which provides stability for convection-dominated flows; adaptive mesh refinement, which controls DG spatial error; reduced basis (RB) spaces, which provide rapidly convergent approximations of the parametric manifolds; the dual-weighted residual (DWR) method, which provides effective error estimates for quantities of interest; the empirical quadrature procedure (EQP), which provides hyper-reduction of the nonlinear residual and error estimates; and adaptive greedy algorithms, which simultaneously trains the DG spaces, RB spaces, and EQP to meet the user-specified output error tolerance. We demonstrate the framework for parametrized aerodynamics problems modeled by the compressible Euler and Reynolds-averaged Navier-Stokes (RANS) equations. In the offline stage, the adaptive greedy algorithm trains reduced models in a fully automated manner. In the online stage, the reduced models accelerate the computation by several orders of magnitude and provide the associated error estimate for the quantities of interest in predictive settings.
LLNL-VIDEO-828661
LLNL-VIDEO-828661