filmov
tv
Simpson Paradox - Explained with Simple Example
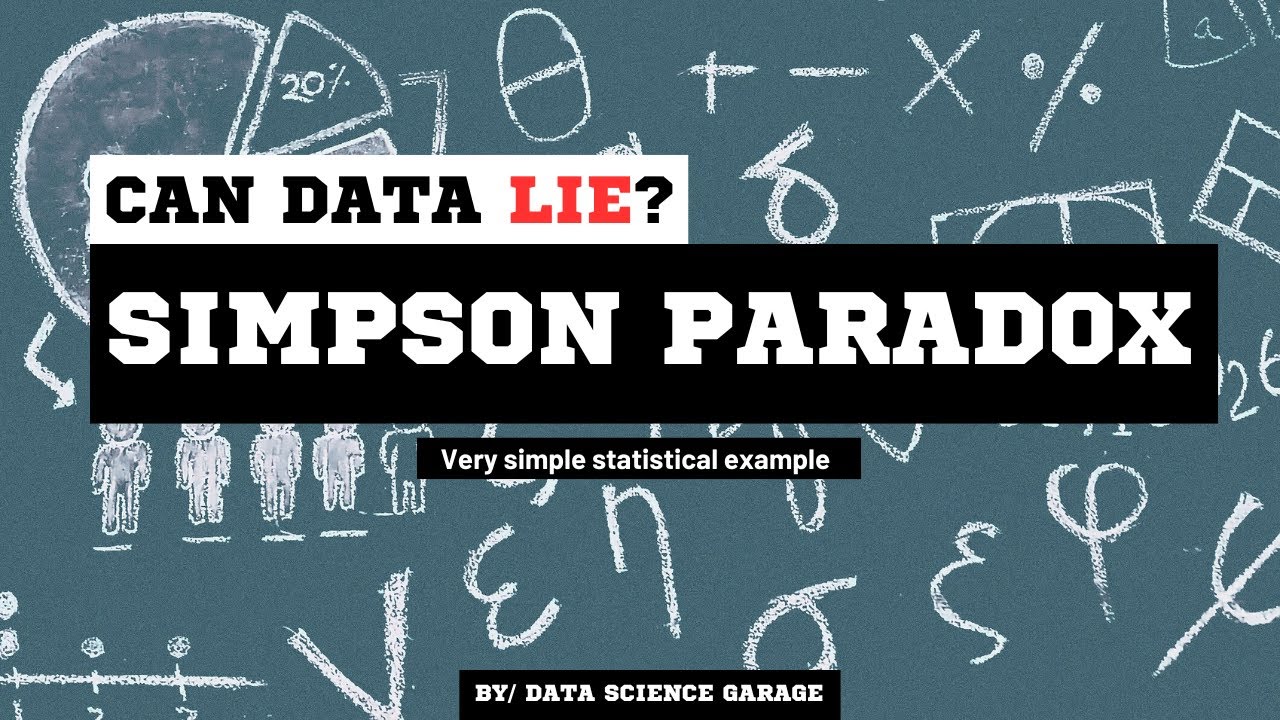
Показать описание
Data is like magic. Why things aren't always as they appear? Can data lie? Yes! Data is the tool for manipulation. There are a series of paradox in math and statistics to do that by purpose or accidentally.
Discover Simpson's Paradox - the mind-bending phenomenon where trends in groups reverse when combined. It is quite difficult to understand for many learners. That is the reason why I decided to provide very simple statistical example why and when Simpson Paradox (a.k.a. Yule-Simpson effect) can happen.
Simpson paradox appears when data partitioning significantly changes the outcome of the analysis. How to choose the correct partitioning? That's the question, going to cover in my next videos.
Probably many times you heard: Correlation doesn't always imply causation. Simpson's Paradox doesn't directly prove or disprove causation, but it highlights why we need to be cautious about inferring causal relationships from observational data, especially when dealing with aggregated statistics. Why? Here is the short list you need to keep in mind:
- Misleading correlations.
- Hidden variables.
- Importances of stratification.
- Causal models (very interesting, more videos will be soon about that, a.k.a. Causal AI)
- Interventions vs. Observation.
This video gets you into the example about drugs (D) A and B. And you will be surprised why data partitioning by Total results in ones outcomes while partitioning by genders turns it upside down and results in another outcomes.
Enjoy!
#simpsonparadox #correlation #causalAI
Discover Simpson's Paradox - the mind-bending phenomenon where trends in groups reverse when combined. It is quite difficult to understand for many learners. That is the reason why I decided to provide very simple statistical example why and when Simpson Paradox (a.k.a. Yule-Simpson effect) can happen.
Simpson paradox appears when data partitioning significantly changes the outcome of the analysis. How to choose the correct partitioning? That's the question, going to cover in my next videos.
Probably many times you heard: Correlation doesn't always imply causation. Simpson's Paradox doesn't directly prove or disprove causation, but it highlights why we need to be cautious about inferring causal relationships from observational data, especially when dealing with aggregated statistics. Why? Here is the short list you need to keep in mind:
- Misleading correlations.
- Hidden variables.
- Importances of stratification.
- Causal models (very interesting, more videos will be soon about that, a.k.a. Causal AI)
- Interventions vs. Observation.
This video gets you into the example about drugs (D) A and B. And you will be surprised why data partitioning by Total results in ones outcomes while partitioning by genders turns it upside down and results in another outcomes.
Enjoy!
#simpsonparadox #correlation #causalAI
Комментарии