filmov
tv
A new neural network for optimal time series processing
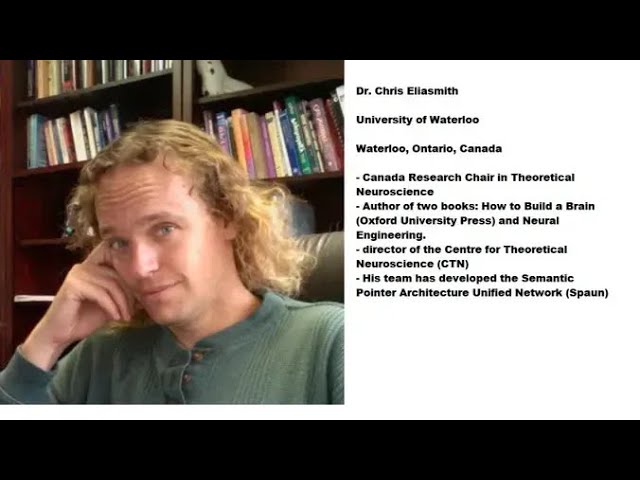
Показать описание
Dr, Chris Eliasmith, cofounder of Applied Brain Research
We have recently discovered a new kind of neural network, called a Legendre Memory Unit (LMU) that is provably optimal for compressing streaming time series data. In this talk, I describe this network, and a variety of state-of-the-art results that have been set using the LMU. I will include recent results on speech and language applications that demonstrate significant improvements over transformers. I will also describe the new ASIC design we have developed to implement this architecture directly in hardware, enabling new large-scale functionality at extremely low power and latency.
Professor Chris Eliasmith is co-CEO and President of Applied Brain Research, an advanced AI company. He is the co-inventor of the Neural Engineering Framework (NEF), the Nengo neural development environment, and the Semantic Pointer Architecture, all of which are dedicated to leveraging our understanding of the brain to advance AI efficiency and scale. His team has developed Spaun, the world's largest functional brain simulation. He won the prestigious 2015 NSERC Polanyi Award for this research.
Chris has published two books, over 120 journal articles and patents, and holds the Canada Research Chair in Theoretical Neuroscience. He is jointly appointed in the Philosophy, Systems Design Engineering faculties, as well being cross-appointed to Computer Science. He is the founding director of the Centre for Theoretical Neuroscience (CTN) at the University of Waterloo. Chris has a Bacon-Erdos number of 8.
Times subject to change
0:00 Chapter Intro
4:38 Speaker Intro
7:25 Presentation
7:57 Time Series AI
8:00 Time Series Problems
9:55 Long-Short Term Memory (LSTM)
11:55 Limitations of LSTM
15:04 Transformer
17:11 A New Neural Network
17:14 The problem
20:01 The solution: Legendre Delay Network (LDN)
24:29 ABR's Legendre Memory Unit (LMU)
25:46 Efficient and Accurate
26:45 A State-of-the-art Neural Network
26:48 Benchmarks: SotA performance on psMNIST
27:32 Practical: SotA on Keyword Spotting
28:28 Practical: SotA on RF Classification
29:02 LMUs for R-peak Detection
29:46 Practical: SotA for Size and Accuracy
32:55 ABR's plans for the LMU
33:16 Hardware: AI Time Series Processor
35:02 Example: Climate control dialog
36:56 Example: Drone interface
38:48 ABR Summary
39:45 Executive Team
40:03 Q&A
We have recently discovered a new kind of neural network, called a Legendre Memory Unit (LMU) that is provably optimal for compressing streaming time series data. In this talk, I describe this network, and a variety of state-of-the-art results that have been set using the LMU. I will include recent results on speech and language applications that demonstrate significant improvements over transformers. I will also describe the new ASIC design we have developed to implement this architecture directly in hardware, enabling new large-scale functionality at extremely low power and latency.
Professor Chris Eliasmith is co-CEO and President of Applied Brain Research, an advanced AI company. He is the co-inventor of the Neural Engineering Framework (NEF), the Nengo neural development environment, and the Semantic Pointer Architecture, all of which are dedicated to leveraging our understanding of the brain to advance AI efficiency and scale. His team has developed Spaun, the world's largest functional brain simulation. He won the prestigious 2015 NSERC Polanyi Award for this research.
Chris has published two books, over 120 journal articles and patents, and holds the Canada Research Chair in Theoretical Neuroscience. He is jointly appointed in the Philosophy, Systems Design Engineering faculties, as well being cross-appointed to Computer Science. He is the founding director of the Centre for Theoretical Neuroscience (CTN) at the University of Waterloo. Chris has a Bacon-Erdos number of 8.
Times subject to change
0:00 Chapter Intro
4:38 Speaker Intro
7:25 Presentation
7:57 Time Series AI
8:00 Time Series Problems
9:55 Long-Short Term Memory (LSTM)
11:55 Limitations of LSTM
15:04 Transformer
17:11 A New Neural Network
17:14 The problem
20:01 The solution: Legendre Delay Network (LDN)
24:29 ABR's Legendre Memory Unit (LMU)
25:46 Efficient and Accurate
26:45 A State-of-the-art Neural Network
26:48 Benchmarks: SotA performance on psMNIST
27:32 Practical: SotA on Keyword Spotting
28:28 Practical: SotA on RF Classification
29:02 LMUs for R-peak Detection
29:46 Practical: SotA for Size and Accuracy
32:55 ABR's plans for the LMU
33:16 Hardware: AI Time Series Processor
35:02 Example: Climate control dialog
36:56 Example: Drone interface
38:48 ABR Summary
39:45 Executive Team
40:03 Q&A
Комментарии