filmov
tv
Interpretable Deep Learning - Deep Learning in Life Sciences - Lecture 05 (Spring 2021)
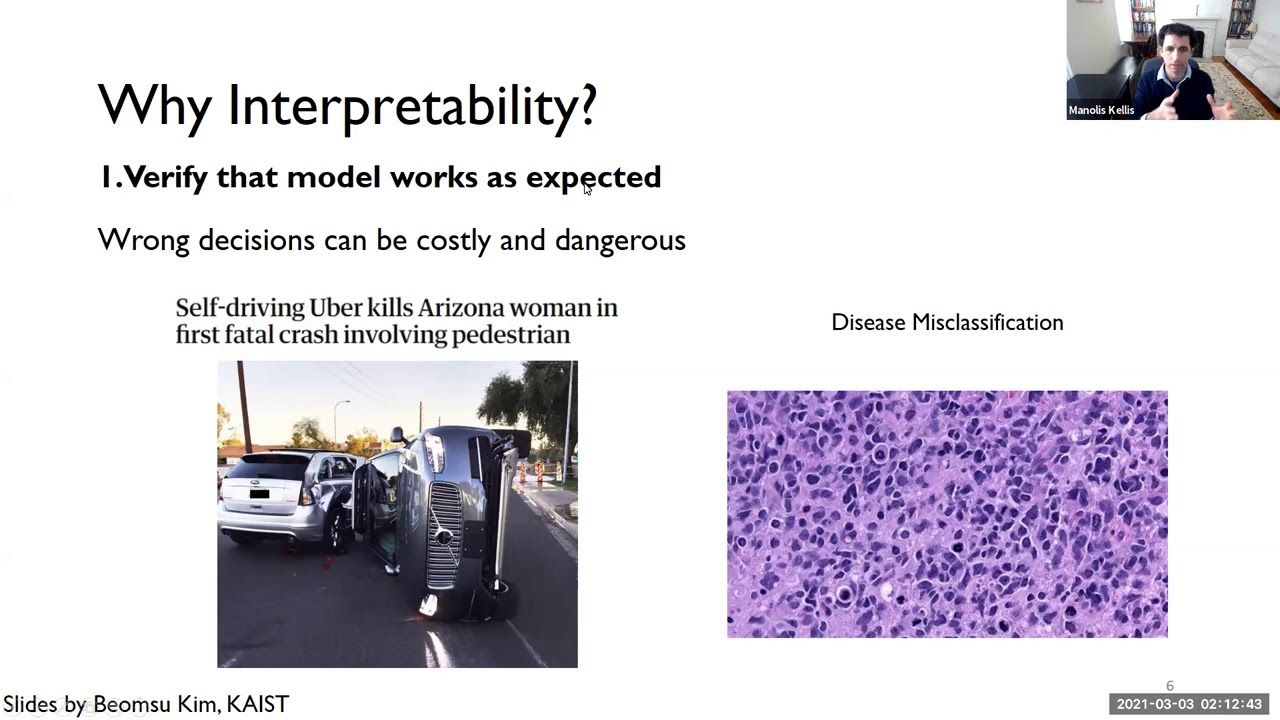
Показать описание
Deep Learning in Life Sciences - Lecture 05 - Interpretable Deep Learning (Spring 2021)
6.874/6.802/20.390/20.490/HST.506 Spring 2021 Prof. Manolis Kellis
Deep Learning in the Life Sciences / Computational Systems Biology
0:00 Lecture outline
3:08 Interpretability: definition, importance
10:30 Interpretability: ante-hoc vs. post-hoc
18:26 Interpreting models: Weight visualization
22:20 Interpreting models: Surrogate model
24:14 Interpreting models: Activation Maximization / Data generation
34:26 Interpreting models: Example-based
39:36 Interpreting decisions
42:24 Interpreting decisions: Example based
45:39 Interpreting decisions: Attribution methods
1:01:17 Interpreting decisions: Gradient based
1:08:55 Interpreting decisions: Backprop-based
1:13:23 Evaluating attributions
1:14:15 Evaluating attributions: Coherence
1:15:30 Evaluating attributions: Class sensitivity
1:16:20 Evaluating attributions: Selectivity
1:19:45 Evaluating attributions: Remove and retrain/keep and retrain
1:21:15 Lecture summary
6.874/6.802/20.390/20.490/HST.506 Spring 2021 Prof. Manolis Kellis
Deep Learning in the Life Sciences / Computational Systems Biology
0:00 Lecture outline
3:08 Interpretability: definition, importance
10:30 Interpretability: ante-hoc vs. post-hoc
18:26 Interpreting models: Weight visualization
22:20 Interpreting models: Surrogate model
24:14 Interpreting models: Activation Maximization / Data generation
34:26 Interpreting models: Example-based
39:36 Interpreting decisions
42:24 Interpreting decisions: Example based
45:39 Interpreting decisions: Attribution methods
1:01:17 Interpreting decisions: Gradient based
1:08:55 Interpreting decisions: Backprop-based
1:13:23 Evaluating attributions
1:14:15 Evaluating attributions: Coherence
1:15:30 Evaluating attributions: Class sensitivity
1:16:20 Evaluating attributions: Selectivity
1:19:45 Evaluating attributions: Remove and retrain/keep and retrain
1:21:15 Lecture summary
Interpretable Deep Learning - Deep Learning in Life Sciences - Lecture 05 (Spring 2021)
Interpretable vs Explainable Machine Learning
Interpretable Deep Learning for New Physics Discovery
USENIX Security '20 - Interpretable Deep Learning under Fire
Interpretable Deep Learning-Based Forensic Iris Segmentation and Recognition
Dimanov Botty - Interpretable Deep Learning
Interpretable Machine Learning: Methods for understanding complex models
Interpretable Deep Learning from Earth Observation Data, Prof. Dr. Plamen Angelov
This Looks Like That: Deep Learning for Interpretable Image Recognition (NeurIPS 2019)
Interpretable Deep Neural Networks by Stéphane Mallat
Interpretable Machine Learning - Interpretable Models - GAM and Boosting
CACM Jan. 2020 - Techniques for Interpretable Machine Learning
Interpretable deep learning for healthcare
Stanford Seminar - ML Explainability Part 1 I Overview and Motivation for Explainability
Interpretable Neural Networks for Computer Vision: Clinical Decisions that are Aided, not Automated
Interpretable Machine Learning Models
Towards Interpretable Deep Neural Networks by Leveraging Adversarial Examples | AISC
Interpretable Deep Learning: More than just a pretty picture
Explainable AI explained! | #3 LIME
Interpretable Deep Learning for Physics
BayLearn 2020: Neural Additive Models: Interpretable Machine Learning with Neural Nets
Interpretable Machine Learning IML Making AI Understandable for Everyone
Interpretable Machine Learning - A Brief History, State-of-the-Art and Challenges
IDSC Day 2023 | Interpretable deep learning approaches for the analysis of medical images: An app...
Комментарии