filmov
tv
YOLO Object Detection (TensorFlow tutorial)
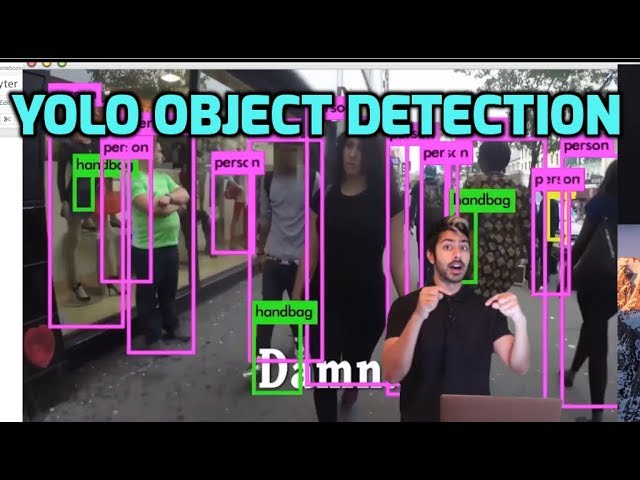
Показать описание
You Only Look Once - this object detection algorithm is currently the state of the art, outperforming R-CNN and it's variants. I'll go into some different object detection algorithm improvements over the years, then dive into YOLO theory and a programmatic implementation using Tensorflow!
Code for this video:
Please Subscribe! And like. And comment. That's what keeps me going.
Want more inspiration & education? Follow me:
More learning resources:
Join us in the Wizards Slack channel:
And please support me on Patreon:
Signup for my newsletter for exciting updates in the field of AI:
Code for this video:
Please Subscribe! And like. And comment. That's what keeps me going.
Want more inspiration & education? Follow me:
More learning resources:
Join us in the Wizards Slack channel:
And please support me on Patreon:
Signup for my newsletter for exciting updates in the field of AI:
YOLO Object Detection (TensorFlow tutorial)
Object detection using YOLO v4 and pre trained model | Deep Learning Tutorial 32 (Tensorflow)
YOLO Object Detection (TensorFlow tutorial)
What is YOLO algorithm? | Deep Learning Tutorial 31 (Tensorflow, Keras & Python)
Object Detection in 10 minutes with YOLOv5 & Python!
Yolo Object Detection tutorial in 5 minutes on Windows | TensorFlow
Yolo Object Detection Tutorial TensorFlow | Complete Guide for Beginners Part #1
Building an Object Detection App with Tensorflow.JS and React.JS in 15 Minutes | COCO SSD
Train Yolov8 object detection on a custom dataset | Step by step guide | Computer vision tutorial
Complete YOLO v8 Custom Object Detection Tutorial | Windows & Linux
Build an Object Detector for Any Game Using YOLO
How To Train an Object Detection Neural Network Using TensorFlow (GPU) on Windows 10
Yolo Object Detection Tutorial TensorFlow | Complete Guide for Beginners Part #2
Train a custom object detection model using your data
Object Detection Explained | Tensorflow Object Detection | AI ML for Beginners | Edureka
Deep Drowsiness Detection using YOLO, Pytorch and Python
Real-time Yolov3 Object Detection for Webcam and Video (using Tensorflow)
YOLOv8 Comparison with Latest YOLO models
Real Time Object Detection on the browser with Yolov7 & Tensorflow.JS
Yolo Object Detection in Google Colab [Full Tutorial]
Yolo Object Detection Tutorial TensorFlow | Complete Guide for Beginners Part #3
TFOD 2.0 Custom Object Detection Step By Step Tutorial
Tensorflow Object Detection in 5 Hours with Python | Full Course with 3 Projects
Object Detection 101 Course - Including 4xProjects | Computer Vision
Комментарии