filmov
tv
Machine Learning Lecture 31 'Random Forests / Bagging' -Cornell CS4780 SP17
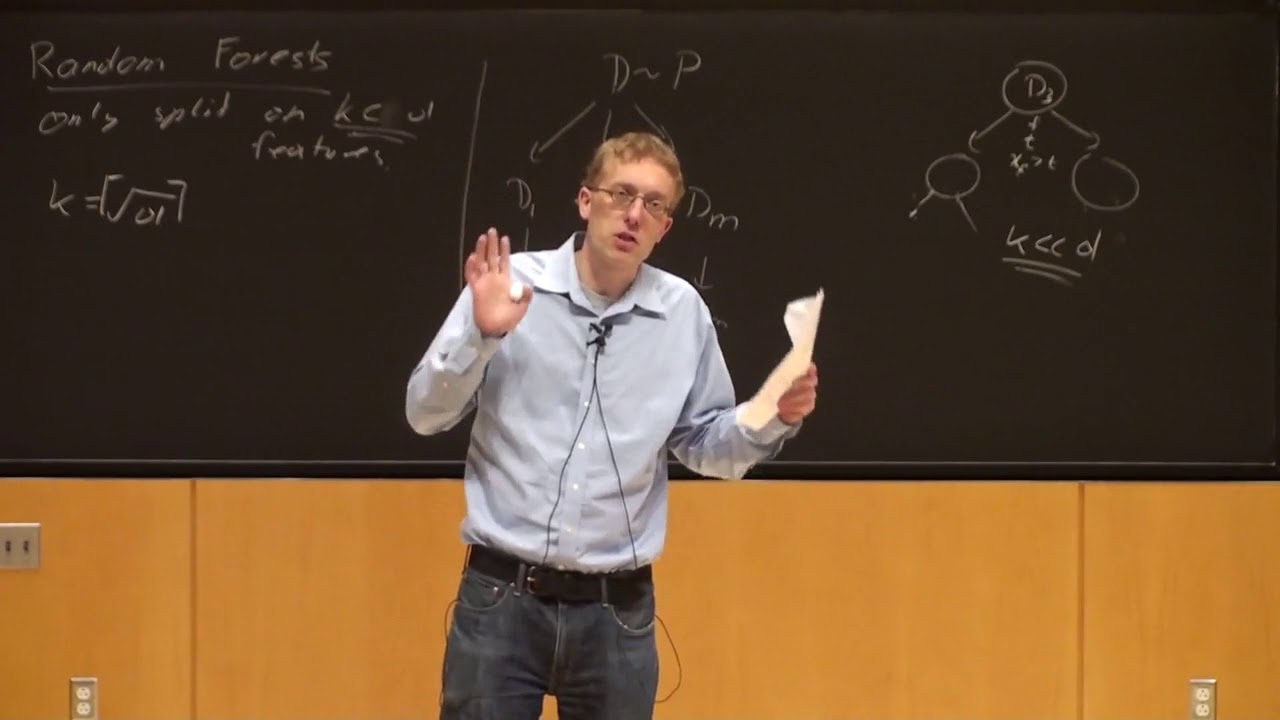
Показать описание
Lecture Notes:
Machine Learning Lecture 31 'Random Forests / Bagging' -Cornell CS4780 SP17
ENSEMBLE LEARNING : BAGGING, BOOSTING et STACKING (25/30)
undergraduate machine learning 31: Decision trees
Random Forest in Machine Learning | Machine Learning Training | Edureka | Machine Learning Live - 3
#31 Machine Learning Specialization [Course 1, Week 3, Lesson 1]
Machine learning - Random forests
Machine Learning in Python | EP. 31 | Random Forest Classifier/Regressor
Introduction to Machine Learning - 08 - Boosting, bagging, and random forests
L61 KCET 2025 Maths | Probability- 3 | Bayes' Theorem #kcet #puboard
What is Random Forest?
06 Bagging & Random Forest - Machine Learning - Winter Term 20/21 - Freie Universität Berlin
Random Forest in Machine Learning | Machine Learning Training | Edureka | Machine Learning Live - 1
Random Forest in Machine Learning: Easy Explanation for Data Science Interviews
Random Forest Classification & Regression | Machine Learning in Tamil - Part 31 | #61
Random Forest in Machine Learning | Machine Learning Training | Edureka | Machine Learning Rewind- 5
Random Forest in Machine Learning | Machine Learning Training | Edureka | Rewind- 7
Tutorial 43-Random Forest Classifier and Regressor
Random Forest in Machine Learning | Machine Learning Training | Edureka | Machine Learning Rewind- 5
Random Forest in Machine Learning | Machine Learning Training | Edureka | Machine Learning Rewind- 5
Random Forest in Machine Learning | Machine Learning Training | Edureka | Machine Learning Rewind- 3
10 ML algorithms in 45 minutes | machine learning algorithms for data science | machine learning
Random Forest Classifier | Data Science | Edureka
Python Machine Learning Tutorial #5 - Decision Trees and Random Forest Classification
Lecture 31 : Autoencoder Training
Комментарии