filmov
tv
Lessons from the regulatory process for medical software for image analysis and AI
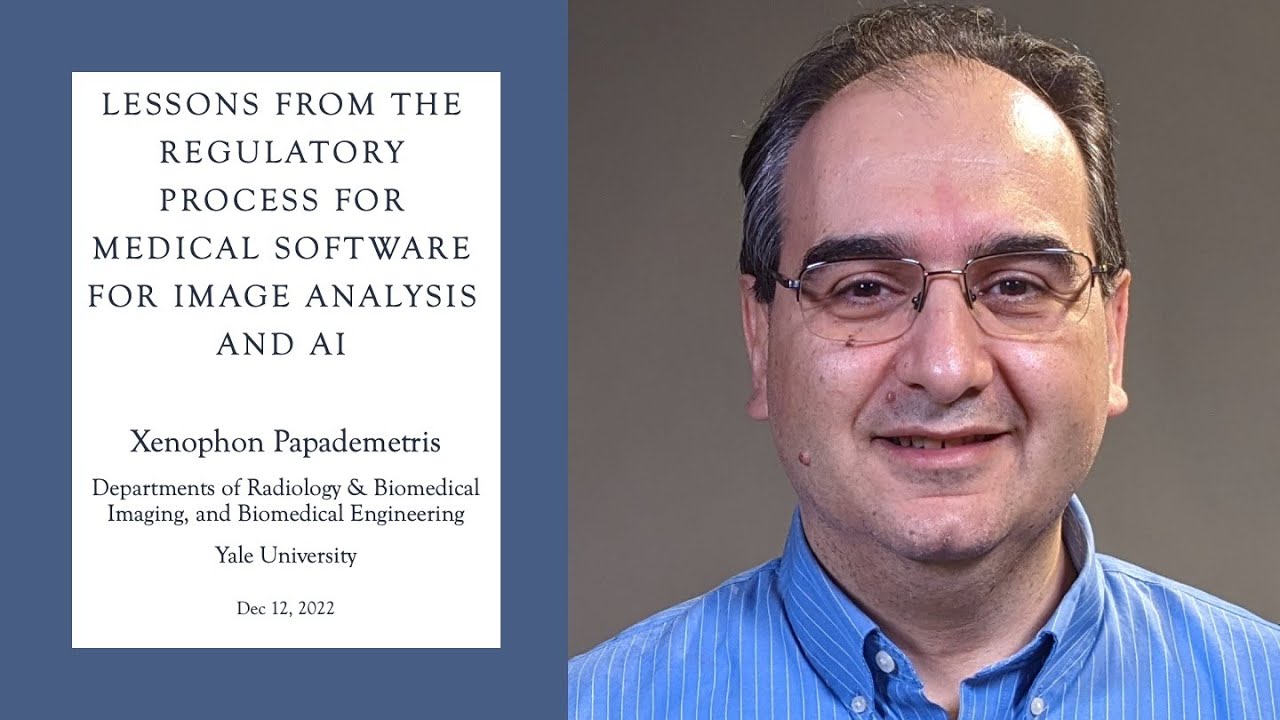
Показать описание
Speaker: Xenophon Papademetris, PhD
Professor, Depts of Radiology & Biomedical Imaging, and Biomedical Engineering, Yale University
This video is a recording of a seminar that was part of the National Institute of Aging (NIH/NIA) Artificial Intelligence Lecture Series. It was presented on December 12th, 2022.
--
Abstract: Artificial intelligence/machine learning (AI/ML) algorithms are currently driving much new research in medical image analysis research. The growth in the number of image analysis publications using such techniques has been exponential. Similarly, in the medical software world, we have also seen an explosion in the number of FDA-cleared standalone medical software devices known as SaMD (Software-as-a-Medical Device), also largely fueled by by AI/ML methods.
Recent developments in AI/ML (primarily deep learning) have been surrounded by a large amount of hype and overpromise; a phenomenon that is common in the history of AI. One of the major problems we face is how to avoid overlearning or overtraining an algorithm from the relatively small training datasets available (as compared to what is used for non-medical applications.) Researchers in the field are familiar with how an algorithm’s performance can deteriorate over time as it gets applied to data from slightly different scanners (or even the same scanner after a minor software upgrade), which are both fundamental due to such overtraining. So, while there are many papers advertising exceptional performance, much of this is artificially inflated. The situation is analogous to the p-hacking (reproducibility) crisis seen in other areas of science.
In this talk, I will review the medical software regulatory process and recent developments in the use of AI in medical image analysis and present some thoughts as to how some of the procedures used in regulated medical software development (explicit quality procedures, risk classification, risk management, usability engineering, external validation) could be applied to AI/ML to potentially allow this potentially game-changing technology to transform human health.
--
00:00:00 Introduction by Dr. Leonid Tsap (NIH/NIA)
00:01:14 Beginning of Talk
00:08:20 An Overview of the Regulatory Process for Medical Software
00:15:19 Machine Learning Background
00:21:44 Some Problems with Current Machine Learning Techniques
00:36:26 Using Lessons from the Regulatory Process to Improve AI Research
00:45:00 Discussion and Conclusions
Professor, Depts of Radiology & Biomedical Imaging, and Biomedical Engineering, Yale University
This video is a recording of a seminar that was part of the National Institute of Aging (NIH/NIA) Artificial Intelligence Lecture Series. It was presented on December 12th, 2022.
--
Abstract: Artificial intelligence/machine learning (AI/ML) algorithms are currently driving much new research in medical image analysis research. The growth in the number of image analysis publications using such techniques has been exponential. Similarly, in the medical software world, we have also seen an explosion in the number of FDA-cleared standalone medical software devices known as SaMD (Software-as-a-Medical Device), also largely fueled by by AI/ML methods.
Recent developments in AI/ML (primarily deep learning) have been surrounded by a large amount of hype and overpromise; a phenomenon that is common in the history of AI. One of the major problems we face is how to avoid overlearning or overtraining an algorithm from the relatively small training datasets available (as compared to what is used for non-medical applications.) Researchers in the field are familiar with how an algorithm’s performance can deteriorate over time as it gets applied to data from slightly different scanners (or even the same scanner after a minor software upgrade), which are both fundamental due to such overtraining. So, while there are many papers advertising exceptional performance, much of this is artificially inflated. The situation is analogous to the p-hacking (reproducibility) crisis seen in other areas of science.
In this talk, I will review the medical software regulatory process and recent developments in the use of AI in medical image analysis and present some thoughts as to how some of the procedures used in regulated medical software development (explicit quality procedures, risk classification, risk management, usability engineering, external validation) could be applied to AI/ML to potentially allow this potentially game-changing technology to transform human health.
--
00:00:00 Introduction by Dr. Leonid Tsap (NIH/NIA)
00:01:14 Beginning of Talk
00:08:20 An Overview of the Regulatory Process for Medical Software
00:15:19 Machine Learning Background
00:21:44 Some Problems with Current Machine Learning Techniques
00:36:26 Using Lessons from the Regulatory Process to Improve AI Research
00:45:00 Discussion and Conclusions