filmov
tv
J. Nathan Kutz: 'Coordinates, governing equations and limits of model discovery'
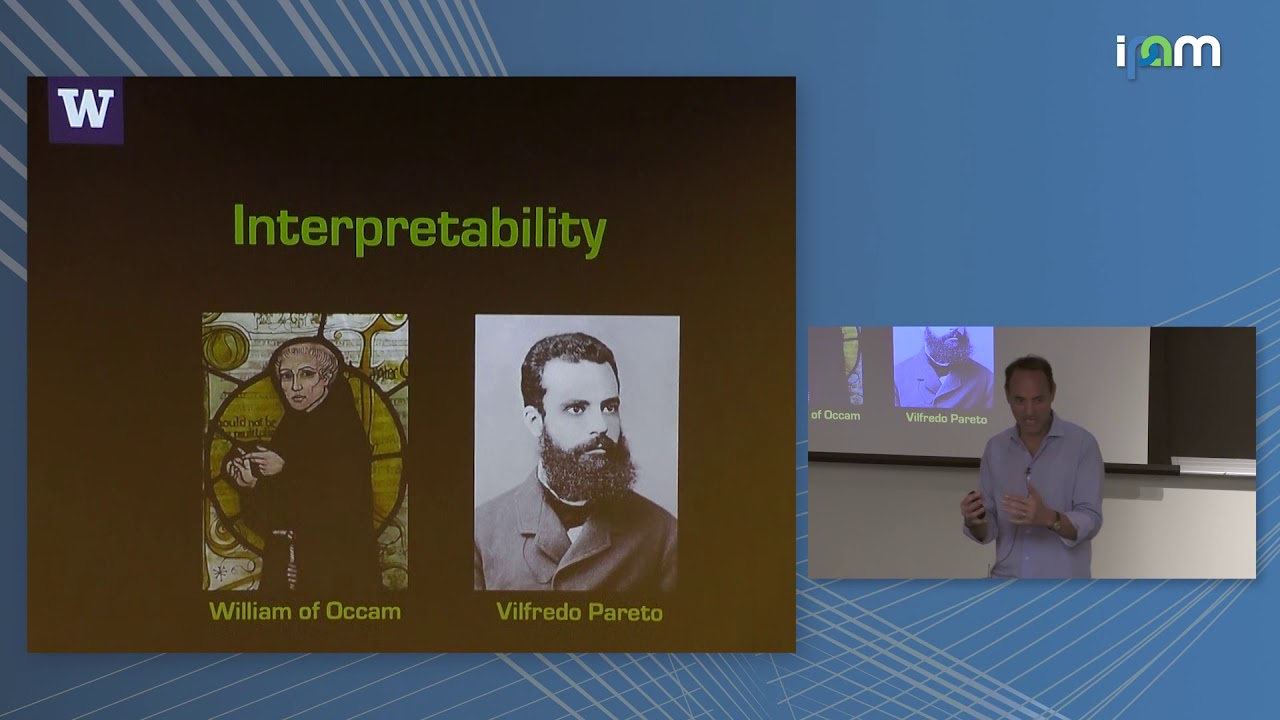
Показать описание
Machine Learning for Physics and the Physics of Learning 2019
Workshop II: Interpretable Learning in Physical Sciences
"Coordinates, governing equations and limits of model discovery"
J. Nathan Kutz - University of Washington, Applied Mathematics
Abstract: Machine learning (ML) and artificial intelligence (AI) algorithms are now being used to automate the discovery of physics principles and governing equations from measurement data alone. Critical for this task is the simultaneous discovery of coordinates and parsimonious governing equations from data. However, positing physical laws from data is challenging without simultaneously proposing an accompanying discrepancy model to account for the inevitable mismatch between theory and measurements. By revisiting the classic problem of modeling falling objects of different size and mass, we highlight a number of subtle and nuanced issues that must be addressed by modern data-driven methods for the automated discovery of physics. Specifically, we show that measurement noise and complex secondary physical mechanisms, such as unsteady fluid drag forces, can obscure the underlying law of gravitation, leading to an erroneous model. Without proposing an appropriate discrepancy model to handle these drag forces, the data supports an Aristotelian, versus a Galilean, theory of gravitation. Using the sparse identification of nonlinear dynamics (SINDy) algorithm, with the additional assumption that each separate falling object is governed by the same physical law, we are able to identify a viable discrepancy model to account for the fluid dynamic forces that explain the mismatch between a posited universal law of gravity and the measurement data. This work highlights the fact that the simple application of ML/AI will generally be insufficient to extract universal physical laws without further modification.
Institute for Pure and Applied Mathematics, UCLA
October 16, 2019
Workshop II: Interpretable Learning in Physical Sciences
"Coordinates, governing equations and limits of model discovery"
J. Nathan Kutz - University of Washington, Applied Mathematics
Abstract: Machine learning (ML) and artificial intelligence (AI) algorithms are now being used to automate the discovery of physics principles and governing equations from measurement data alone. Critical for this task is the simultaneous discovery of coordinates and parsimonious governing equations from data. However, positing physical laws from data is challenging without simultaneously proposing an accompanying discrepancy model to account for the inevitable mismatch between theory and measurements. By revisiting the classic problem of modeling falling objects of different size and mass, we highlight a number of subtle and nuanced issues that must be addressed by modern data-driven methods for the automated discovery of physics. Specifically, we show that measurement noise and complex secondary physical mechanisms, such as unsteady fluid drag forces, can obscure the underlying law of gravitation, leading to an erroneous model. Without proposing an appropriate discrepancy model to handle these drag forces, the data supports an Aristotelian, versus a Galilean, theory of gravitation. Using the sparse identification of nonlinear dynamics (SINDy) algorithm, with the additional assumption that each separate falling object is governed by the same physical law, we are able to identify a viable discrepancy model to account for the fluid dynamic forces that explain the mismatch between a posited universal law of gravity and the measurement data. This work highlights the fact that the simple application of ML/AI will generally be insufficient to extract universal physical laws without further modification.
Institute for Pure and Applied Mathematics, UCLA
October 16, 2019