filmov
tv
Dimensionally Consistent Learning with Buckingham Pi
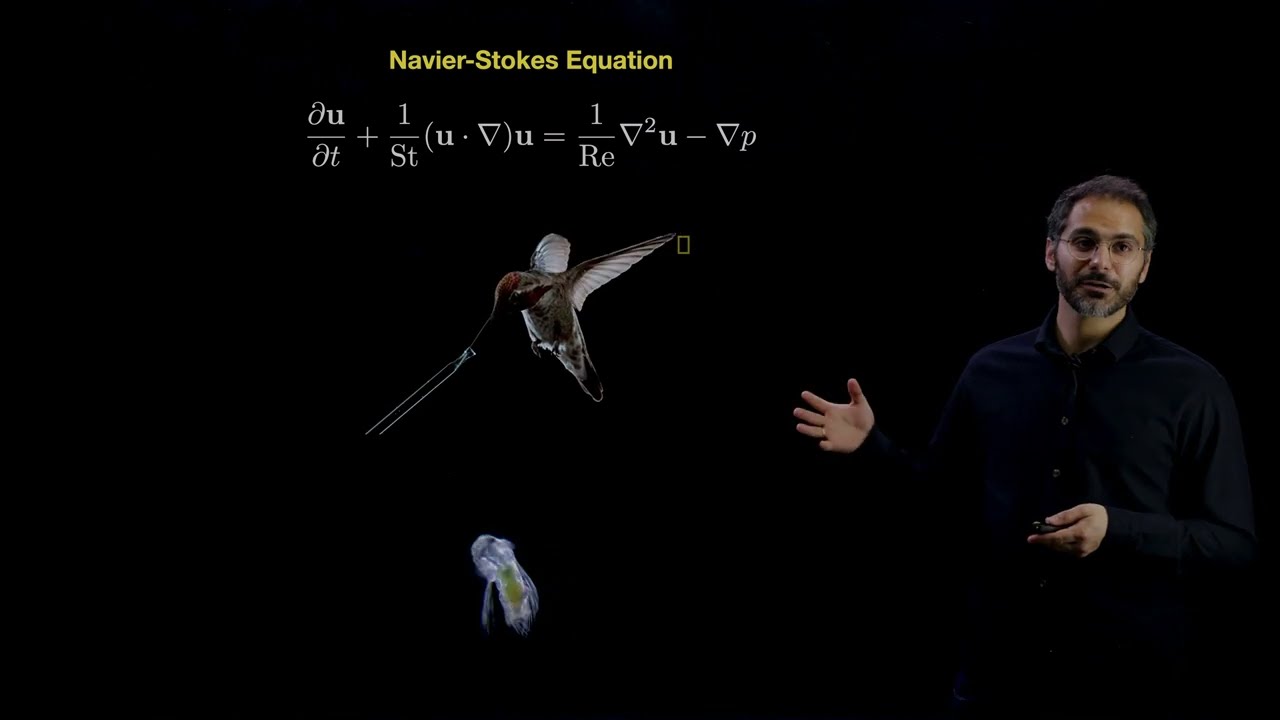
Показать описание
In the absence of governing equations, dimensional analysis is a robust technique for extracting insights and finding symmetries in physical systems. Given measurement variables and parameters, the Buckingham Pi theorem provides a procedure for finding a set of dimensionless groups that spans the solution space, although this set is not unique. We propose an automated approach using the symmetric and self-similar structure of available measurement data to discover the dimensionless groups that best collapse this data to a lower dimensional space according to an optimal fit. We develop three data-driven techniques that use the Buckingham Pi theorem as a constraint: (i) a constrained optimization problem with a non-parametric input-output fitting function, (ii) a deep learning algorithm (BuckiNet) that projects the input parameter space to a lower dimension in the first layer, and (iii) a technique based on sparse identification of nonlinear dynamics (SINDy) to discover dimensionless equations whose coefficients parameterize the dynamics. We explore the accuracy, robustness and computational complexity of these methods as applied to three example problems: a bead on a rotating hoop, a laminar boundary layer, and Rayleigh-Bénard convection.
Комментарии