filmov
tv
Stable Diffusion from Scratch in PyTorch | Unconditional Latent Diffusion Models
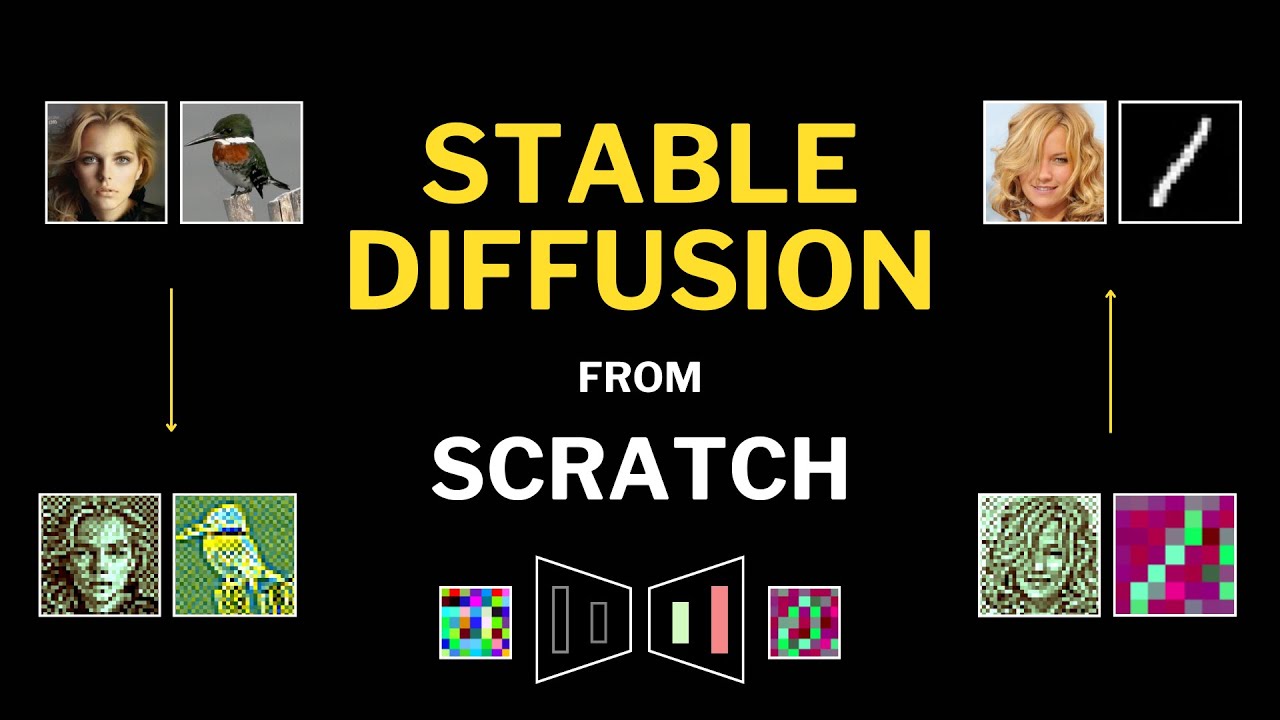
Показать описание
In this video, we'll cover everything from the building blocks of stable diffusion to its implementation in PyTorch and see how to build and train Stable Diffusion from scratch.
This is Part I of the tutorial where I explain latent diffusion models specifically unconditional latent diffusion models. We dive deep into what is latent diffusion , how latent diffusion works , what are the components and losses used in training latent diffusion models and then finally implement and train latent diffusion models.
The second part will cover conditional latent diffusion models and we will transition to Stable diffusion. The series will be a stable diffusion guide from scratch and you will be able to code stable diffusion in pytorch by yourself by end of it.
⏱️ Timestamps
00:00 Intro
00:56 Recap of Diffusion Models
3:31 Why Latent Diffusion Models
4:31 Introduction to Latent Diffusion Models
5:55 Review of VAE : Variational Auto Encoder
6:29 Review of VQVAE : Vector Quantised Variational Auto Encoder
7:36 Issue with L2 Reconstruction Loss for Latent Diffusion Models
8:50 Perceptual Loss
13:44 LPIPS Implementation
16:40 Adversarial Loss in Latent Diffusion Models
19:38 AutoEncoder Architecture in Latent Diffusion Models
23:04 VAE Implementation vs VQVAE Implementation
24:22 Autoencoder Implementation for Latent Diffusion Models
32:23 Training AutoEncoder for Latent Diffusion Models
32:37 Discriminator for Latent Diffusion Models
36:33 Results of Autoencoder Training
37:40 VQGAN = VQVAE + LPIPS + Discriminator
38:26 Latent Diffusion Model Architecture
39:37 Training Diffusion of Latent Diffusion Models
41:11 Latent Diffusion Model Results
41:45 Whats Next
42:18 Outro
🔔 Subscribe :
📌 Keywords:
#stablediffusion #stable_diffusion
This is Part I of the tutorial where I explain latent diffusion models specifically unconditional latent diffusion models. We dive deep into what is latent diffusion , how latent diffusion works , what are the components and losses used in training latent diffusion models and then finally implement and train latent diffusion models.
The second part will cover conditional latent diffusion models and we will transition to Stable diffusion. The series will be a stable diffusion guide from scratch and you will be able to code stable diffusion in pytorch by yourself by end of it.
⏱️ Timestamps
00:00 Intro
00:56 Recap of Diffusion Models
3:31 Why Latent Diffusion Models
4:31 Introduction to Latent Diffusion Models
5:55 Review of VAE : Variational Auto Encoder
6:29 Review of VQVAE : Vector Quantised Variational Auto Encoder
7:36 Issue with L2 Reconstruction Loss for Latent Diffusion Models
8:50 Perceptual Loss
13:44 LPIPS Implementation
16:40 Adversarial Loss in Latent Diffusion Models
19:38 AutoEncoder Architecture in Latent Diffusion Models
23:04 VAE Implementation vs VQVAE Implementation
24:22 Autoencoder Implementation for Latent Diffusion Models
32:23 Training AutoEncoder for Latent Diffusion Models
32:37 Discriminator for Latent Diffusion Models
36:33 Results of Autoencoder Training
37:40 VQGAN = VQVAE + LPIPS + Discriminator
38:26 Latent Diffusion Model Architecture
39:37 Training Diffusion of Latent Diffusion Models
41:11 Latent Diffusion Model Results
41:45 Whats Next
42:18 Outro
🔔 Subscribe :
📌 Keywords:
#stablediffusion #stable_diffusion
Комментарии