filmov
tv
RIMeshGNN: A Rotation-Invariant Graph Neural Network for Mesh Classification
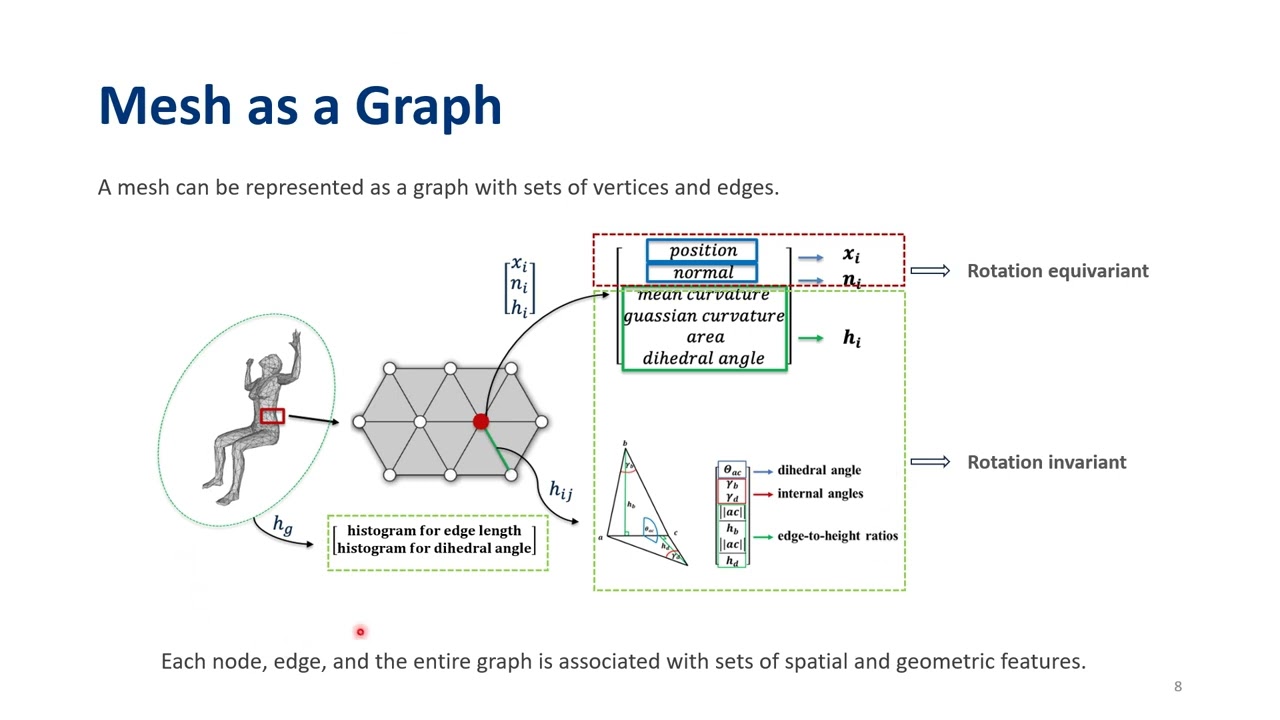
Показать описание
Authors: Bahareh Shakibajahromi; Edward Kim; David E. Breen
Description: Shape analysis tasks, including mesh classification, segmentation, and retrieval demonstrate symmetries in Euclidean space and should be invariant to geometric transformations such as rotation and translation. However, existing methods in mesh analysis often rely on extensive data augmentation and more complex analysis models to handle 3D rotations. Despite these efforts, rotation invariance is not guaranteed, which can significantly reduce accuracy when test samples undergo arbitrary rotations, because the analysis method struggles to generalize to the unknown orientations of the test samples. To address these challenges, our work presents a novel approach that employs graph neural networks (GNNs) to analyze mesh-structured data. Our proposed GNN layer, aggregation function, and local pooling layer are equivariant to the rotation, reflection and translation of 3D shapes, making them suitable building blocks for our proposed rotation-invariant network for the classification of mesh models. Therefore, our proposed approach does not need rotation augmentation, and we can maintain accuracy even when test samples undergo arbitrary rotations. Extensive experiments on various datasets demonstrate that our methods achieve state-of-the-art performance.
Description: Shape analysis tasks, including mesh classification, segmentation, and retrieval demonstrate symmetries in Euclidean space and should be invariant to geometric transformations such as rotation and translation. However, existing methods in mesh analysis often rely on extensive data augmentation and more complex analysis models to handle 3D rotations. Despite these efforts, rotation invariance is not guaranteed, which can significantly reduce accuracy when test samples undergo arbitrary rotations, because the analysis method struggles to generalize to the unknown orientations of the test samples. To address these challenges, our work presents a novel approach that employs graph neural networks (GNNs) to analyze mesh-structured data. Our proposed GNN layer, aggregation function, and local pooling layer are equivariant to the rotation, reflection and translation of 3D shapes, making them suitable building blocks for our proposed rotation-invariant network for the classification of mesh models. Therefore, our proposed approach does not need rotation augmentation, and we can maintain accuracy even when test samples undergo arbitrary rotations. Extensive experiments on various datasets demonstrate that our methods achieve state-of-the-art performance.