filmov
tv
I made OD bots for Gaming in 30 MINUTES
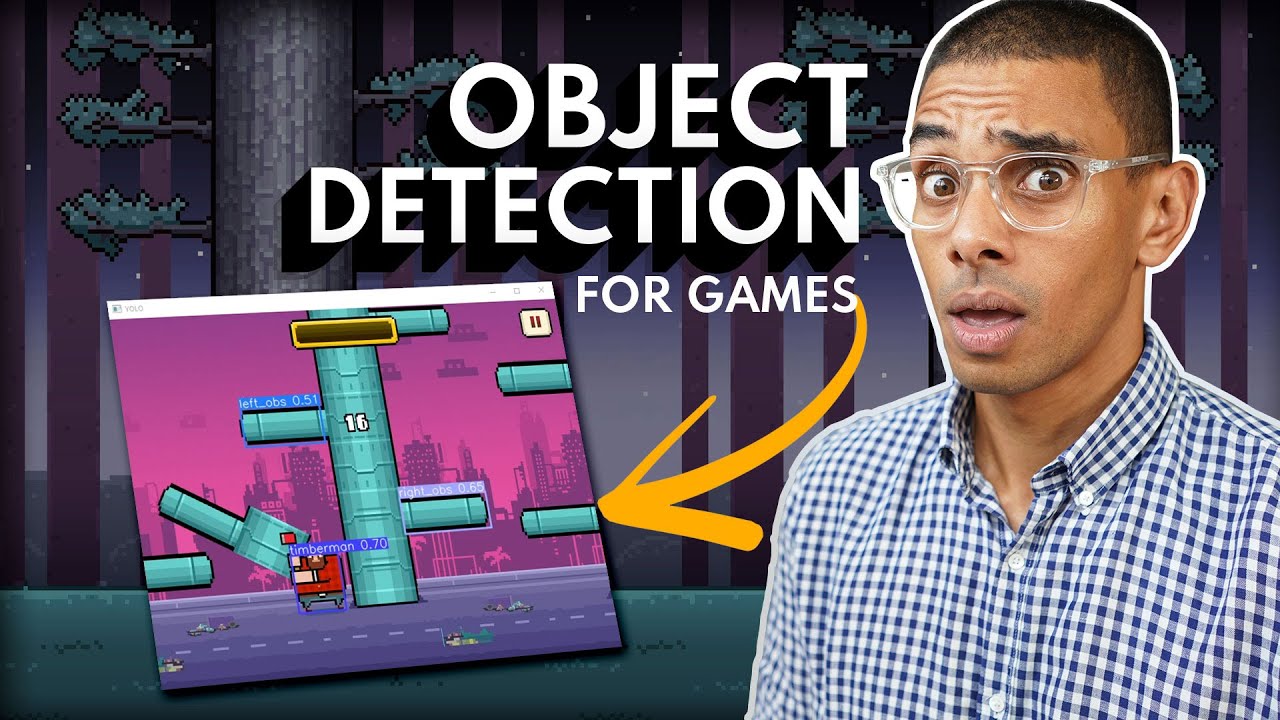
Показать описание
Well...I tried to lol.
Want to learn how to use Object Detection in games? Say no more! In this video I ridiculously tried to do it in 30 minutes using Python and a Pytorch implementation of the famous YOLO model.
Chapters
0:00 - START
0:33 - Explainer
1:35 - Mission 1: Setup YOLO
2:00 - Breakdown Boar
19:58 - Mission 2: Capture Timberman Data
30:50 - Mission 3: Label Images
43:03 - Mission 4: Train and Test Deep Learning model
1:09:14 - Fixing Up Performance
Oh, and don't forget to connect with me!
Happy coding!
Nick
P.s. Let me know how you go and drop a comment if you need a hand!
#yolo #python
Want to learn how to use Object Detection in games? Say no more! In this video I ridiculously tried to do it in 30 minutes using Python and a Pytorch implementation of the famous YOLO model.
Chapters
0:00 - START
0:33 - Explainer
1:35 - Mission 1: Setup YOLO
2:00 - Breakdown Boar
19:58 - Mission 2: Capture Timberman Data
30:50 - Mission 3: Label Images
43:03 - Mission 4: Train and Test Deep Learning model
1:09:14 - Fixing Up Performance
Oh, and don't forget to connect with me!
Happy coding!
Nick
P.s. Let me know how you go and drop a comment if you need a hand!
#yolo #python
I made OD bots for Gaming in 30 MINUTES
Chcecie to zobaczyć?🤫😏
Co dostaliscie od mikolaja? ☺️🎄
Tary používá místo pásku tkaničku od bot! 🤯
Why you should make useless things | Simone Giertz
Magnet Power! Making Awesome INFINITY GAUNTLET
Made Andy můj první programovatelný robot od 4kids.cz
BotSquad™ WowWee® od MaDe
Fortnite makes no sense...
PAW Patrol Fluffy Slime Time Game 🐶 Guess the Character! | Stay Home #WithMe | Nick Jr.
Make $300 a Day with AI Software (How To Make Money Online 2025)
Made Robot policejní Guliver 32 cm od maxikovi hračky.cz
Mop Vileda Made in China nie podaje pary.Pompka od zabawki😉
Discord Bot od podstaw w DiscordJS (#15) Subkomendy i grupy subkomend / Wstęp do Embed
Opening a dam gate after years of it being closed #amazing
Vložky do bot od Pedag mi pomáhají při náročném programu. #florbal #běh #run
Bot Od Rajdowania Tutorial pt. 2
Made Robofotbal na dálkové ovládání od 4kids.cz
The Greek myth of Talos, the first robot - Adrienne Mayor
Emo what's your sign? And what od your sing? #emo #shorts #trend #cute #sing #funny #pets #tech...
How To Become A Sonic Sway PRO In Dragon Ball Sparking Zero
Solar System Model From a Drone's View
Is soy bad for you? - Francesca Bot
The Furnace Bots | Think Like A Coder, Ep 3
Комментарии