filmov
tv
Materials Project Seminars – Tonio Buonassisi, 'Inverse Design: Why Aren't We There Yet?'
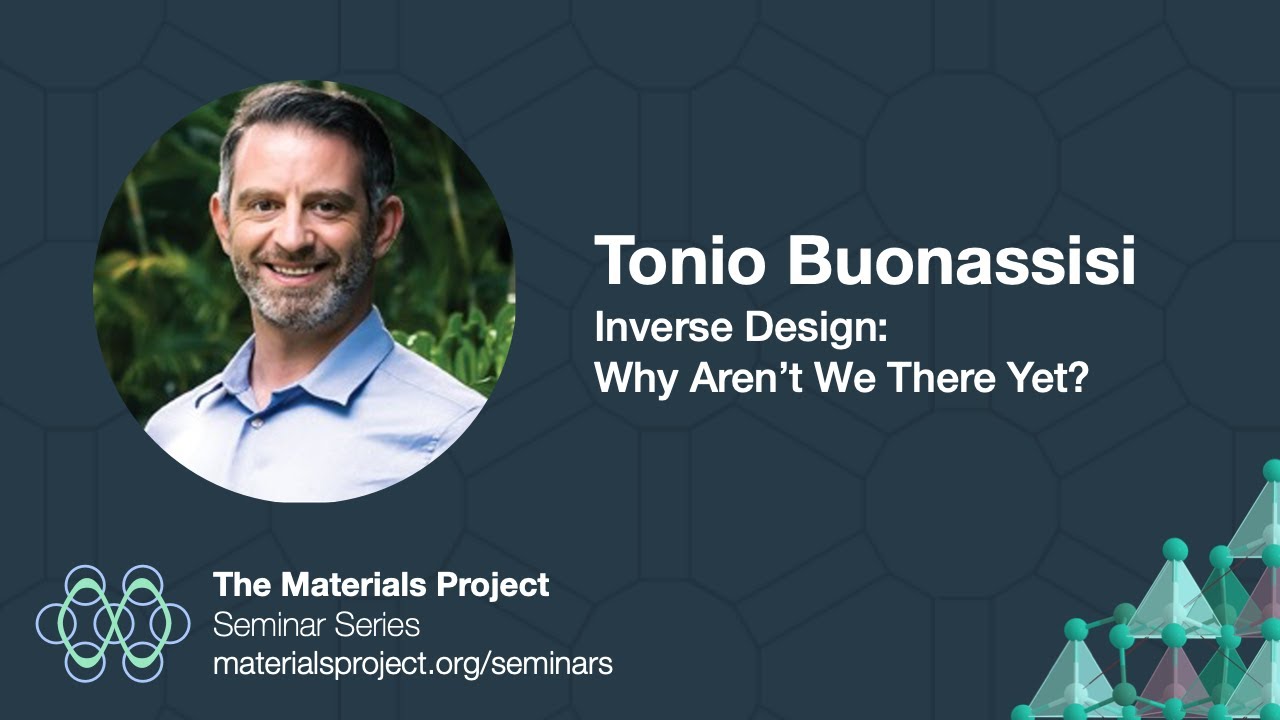
Показать описание
Title: "Inverse Design: Why Aren't We There Yet?"
Presented as a Materials Project Seminar on August 15th 2022.
Speaker: Tonio Buonassisi, Professor of Mechanical Engineering, Massachusetts Institute of Technology
Abstract: Inverse design entails the intentional creation of a material embodying user-defined properties. It’s widely accepted that only a small fraction of possible materials has been synthesized, and thus, an inverse-design tool can help navigate unexplored spaces more resource-efficiently than current approaches. Historically, inverse design was envisioned considering available toolsets, initially heuristics and intuition, later first-principles calculations, and more recently, machine-learning (ML) methods that leverage elements of the latter two as training data or constraints.
In this presentation, I’ll review the state of the art of ML-driven inverse design, and highlight outstanding gaps to be addressed. Highlights include novel materials representations that reflect underlying physics of elements and their diverse combinations, ML algorithms designed to capture and generalize patterns from known compounds, refinement approaches designed to eliminate junk data, and early attempts to integrate inverse design into experimental workflows. I hope the audience will leave energized to contribute to this high-risk, high-reward research area, which in this decade promises to deliver surprising breakthroughs and commensurate industrial impacts.
Presented as a Materials Project Seminar on August 15th 2022.
Speaker: Tonio Buonassisi, Professor of Mechanical Engineering, Massachusetts Institute of Technology
Abstract: Inverse design entails the intentional creation of a material embodying user-defined properties. It’s widely accepted that only a small fraction of possible materials has been synthesized, and thus, an inverse-design tool can help navigate unexplored spaces more resource-efficiently than current approaches. Historically, inverse design was envisioned considering available toolsets, initially heuristics and intuition, later first-principles calculations, and more recently, machine-learning (ML) methods that leverage elements of the latter two as training data or constraints.
In this presentation, I’ll review the state of the art of ML-driven inverse design, and highlight outstanding gaps to be addressed. Highlights include novel materials representations that reflect underlying physics of elements and their diverse combinations, ML algorithms designed to capture and generalize patterns from known compounds, refinement approaches designed to eliminate junk data, and early attempts to integrate inverse design into experimental workflows. I hope the audience will leave energized to contribute to this high-risk, high-reward research area, which in this decade promises to deliver surprising breakthroughs and commensurate industrial impacts.