filmov
tv
What are Assumptions of Linear Regression? Easy Explanation for Data Science Interviews
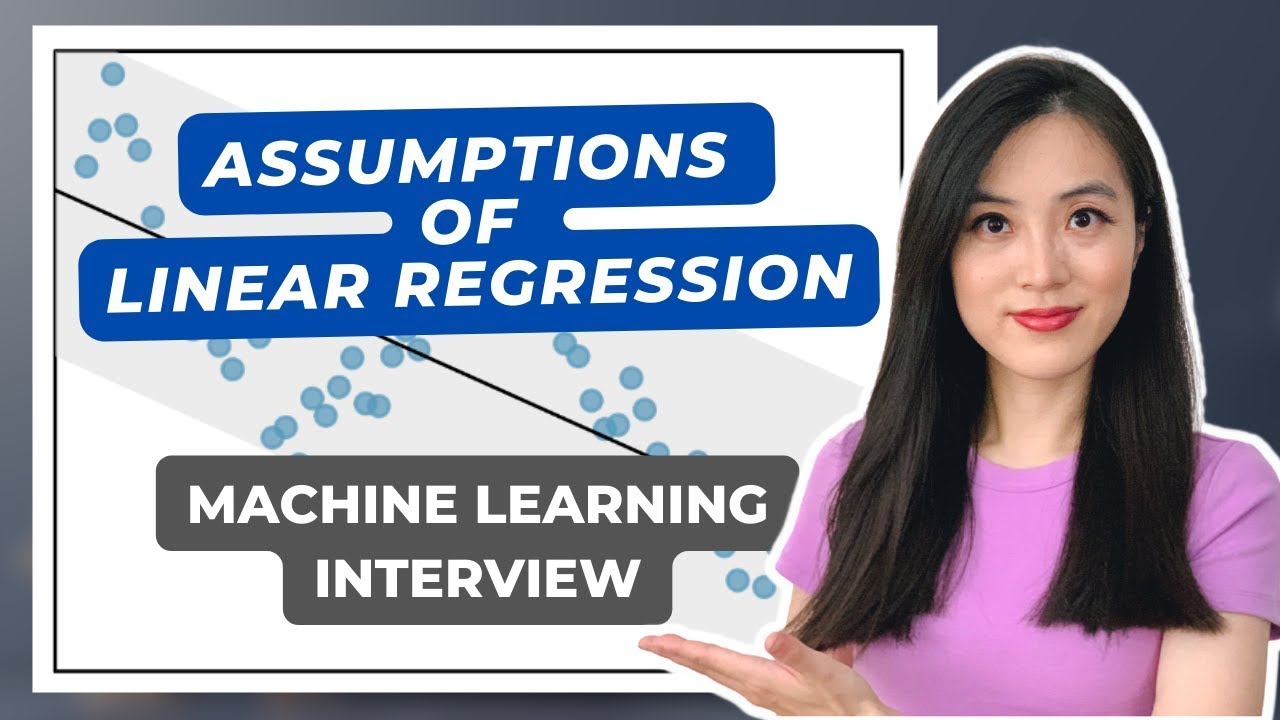
Показать описание
In this video, we’ll go over the four assumptions of linear regression. Interviewers frequently ask questions about linear regression, so we’ll dive into both the assumptions themselves, as well as how to diagnose violations of the assumptions. I’ll also offer insight into which assumptions are critical versus which are less important.
🟢Get all my free data science interview resources
// Comment
Got any questions? Something to add?
Write a comment below to chat.
// Let's connect on LinkedIn:
====================
Contents of this video:
====================
00:00 Introduction
00:43 The Assumptions
01:38 Things To Note
02:04 Assumption #1
02:50 How To Diagnose
03:16 Residual Plots
03:48 Assumption #2
04:17 How To Diagnose
04:52 Assumption #3
05:19 Violations of Normality
05:57 Q-Q Plots
07:12 Summary of Q-Q Plots
07:24 Assumption #4
07:55 How To Diagnose
08:15 Residual Plots
08:53 To Summarize
🟢Get all my free data science interview resources
// Comment
Got any questions? Something to add?
Write a comment below to chat.
// Let's connect on LinkedIn:
====================
Contents of this video:
====================
00:00 Introduction
00:43 The Assumptions
01:38 Things To Note
02:04 Assumption #1
02:50 How To Diagnose
03:16 Residual Plots
03:48 Assumption #2
04:17 How To Diagnose
04:52 Assumption #3
05:19 Violations of Normality
05:57 Q-Q Plots
07:12 Summary of Q-Q Plots
07:24 Assumption #4
07:55 How To Diagnose
08:15 Residual Plots
08:53 To Summarize
Assumptions of Linear Regression
What are Assumptions of Linear Regression? Easy Explanation for Data Science Interviews
Simple Linear Regression: Assumptions
Assumptions of Linear Regression | What are the assumptions for a linear regression model
Assumptions of Linear Regression
Assumptions of Linear Regression
Regression assumptions explained!
Assumptions of Linear Regression
Level 2 - Quantitative Methods & Derivatives
Assumptions in Linear Regression - explained | residual analysis
Simple Linear Regression: Checking Assumptions with Residual Plots
What are the main Assumptions of Linear Regression? | Top 5 Assumptions of Linear Regression
Assumptions of LP|Basic assumptions of Lp|Properties of LP Model|Limitations of LP|GTU|OR|LPP
What are the assumptions of Linear Regression? | Machine Learning Interview Questions
Checking assumptions of the linear model
5. Assumptions of Linear Regression
Assumptions of Linear Regression
What are the assumptions of linear regression model? - Machine Learning
Assumptions of Linear Regression | Part1
Assumptions of linear Regression | explained in simplest way
Econometrics Lecture: The Classical Assumptions
Assumptions Underlying Simple Linear Regression
Five assumptions of linear regression
Linear Regression Assumptions Part I - SAS for Beginners (Lesson 22)
Комментарии