filmov
tv
MACHINE LEARNING THROUGH THE INFORMATION BOTTLENECK
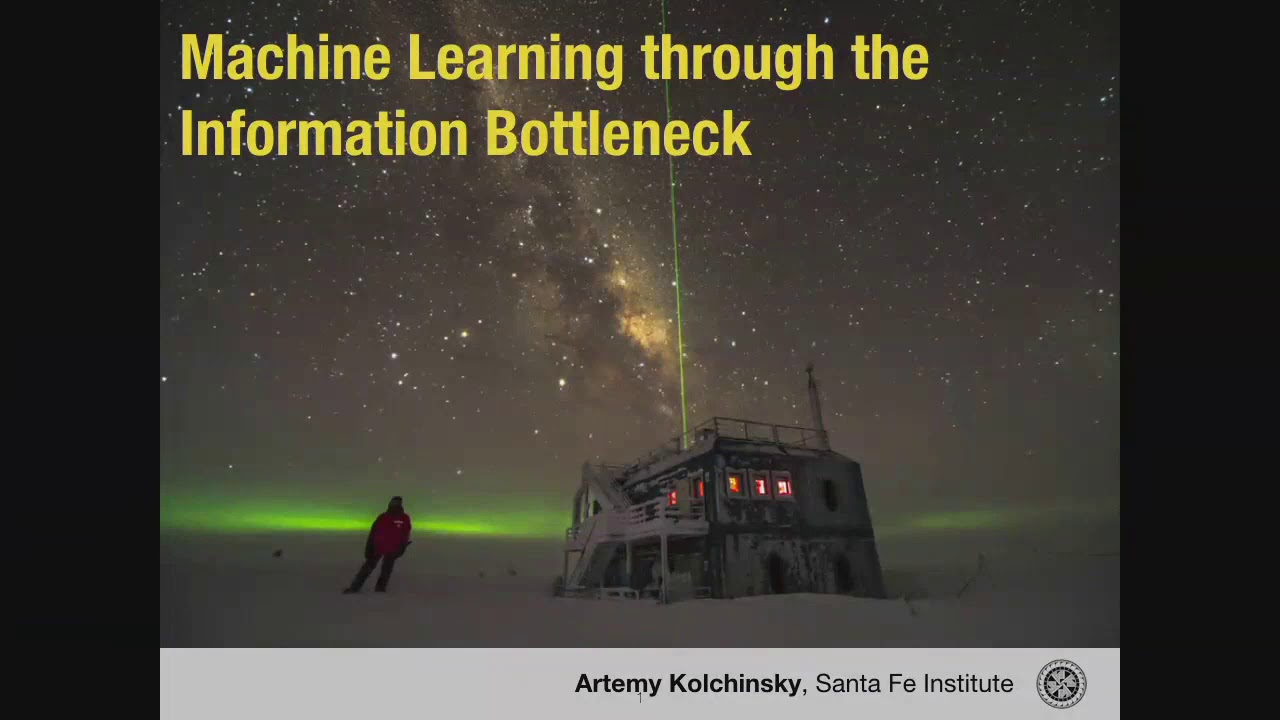
Показать описание
2/7/20
Artemy Kolchinsky (Santa Fe Inst)
Abstract:
The information bottleneck (IB) has been proposed as a principled way to compress a random variable, while only preserving that information which is relevant for predicting another random variable. In recent times, the IB has been proposed --- and challenged --- as a theoretical framework for understanding why and how deep learning architectures achieve good performance. I will cover: (1) an introduction to the ideas behind IB, (2) methods for implementing information-theoretic compression in neural networks + some possible applications of such methods, (3) the current status of the IB theory of deep learning, (4) recently discovered caveats that arise for IB in machine learning scenarios
Artemy Kolchinsky (Santa Fe Inst)
Artemy Kolchinsky is a postdoctoral fellow at the Santa Fe Institute (Santa Fe, NM). His work lies at the intersection of information theory, statistical physics, and machine learning. He is interested in using tools from statistical physics to derive fundamental bounds on the ability of real-world agents -- whether protocells, organisms, or computers -- to acquire and exploit information in adaptive ways.
Artemy Kolchinsky (Santa Fe Inst)
Abstract:
The information bottleneck (IB) has been proposed as a principled way to compress a random variable, while only preserving that information which is relevant for predicting another random variable. In recent times, the IB has been proposed --- and challenged --- as a theoretical framework for understanding why and how deep learning architectures achieve good performance. I will cover: (1) an introduction to the ideas behind IB, (2) methods for implementing information-theoretic compression in neural networks + some possible applications of such methods, (3) the current status of the IB theory of deep learning, (4) recently discovered caveats that arise for IB in machine learning scenarios
Artemy Kolchinsky (Santa Fe Inst)
Artemy Kolchinsky is a postdoctoral fellow at the Santa Fe Institute (Santa Fe, NM). His work lies at the intersection of information theory, statistical physics, and machine learning. He is interested in using tools from statistical physics to derive fundamental bounds on the ability of real-world agents -- whether protocells, organisms, or computers -- to acquire and exploit information in adaptive ways.