filmov
tv
Fast approximation of complicated numerical models
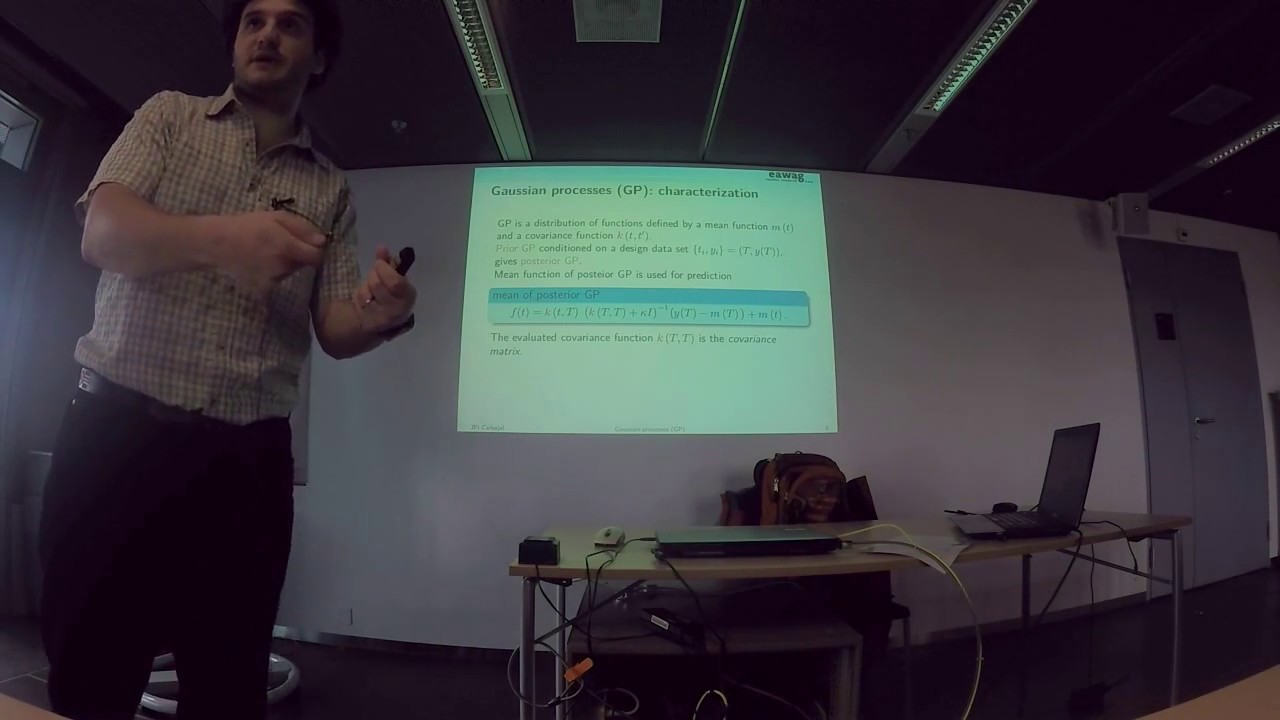
Показать описание
This is an overview talk given at
Eawag's SIAM seminar 28.02.2017
Organized by Dr. Carlo Albert
Abstract
Models play an important role in the understanding of measured data streams. When interpretable model parameters are inferred from the data we gain insights into the observed system. In the current Big Data hype, **differential equation**(DE) models, which explicitly represent our knowledge of underlying mechanisms, seem to be kept at bay due to the heterogeneous nature of the measurements and our poor understanding of the generating processes.
The most popular methods in the Big Data realm allow us to exploit
patchy prior knowledge when analyzing data streams. Among hese
methods we find **spatio-temporal Gaussian processes** (GP) regression and classification (Kriging for the geostatistician), which enable us to introduce prior knowledge in the covariance structure of the observed variables. Classical methods are also popular, such as **regularized nonlinear regression**, where prior knowledge is
introduced in the weight of acceptable solutions. In this talk I will present an unifying perspective that brings all these methods under the same hood. This will lead to a interchangeability of GP inference (O(N^3)) with Kalman or Bayesian filter type of methods (O(N)), which give us the interpretability of the GP formulation (or regularized regression) and the efficiency of the filtering approach.
Finally we will overview some clever ideas on how to speed up large distributed models using emulators constructed with the methods mentioned above.
Eawag's SIAM seminar 28.02.2017
Organized by Dr. Carlo Albert
Abstract
Models play an important role in the understanding of measured data streams. When interpretable model parameters are inferred from the data we gain insights into the observed system. In the current Big Data hype, **differential equation**(DE) models, which explicitly represent our knowledge of underlying mechanisms, seem to be kept at bay due to the heterogeneous nature of the measurements and our poor understanding of the generating processes.
The most popular methods in the Big Data realm allow us to exploit
patchy prior knowledge when analyzing data streams. Among hese
methods we find **spatio-temporal Gaussian processes** (GP) regression and classification (Kriging for the geostatistician), which enable us to introduce prior knowledge in the covariance structure of the observed variables. Classical methods are also popular, such as **regularized nonlinear regression**, where prior knowledge is
introduced in the weight of acceptable solutions. In this talk I will present an unifying perspective that brings all these methods under the same hood. This will lead to a interchangeability of GP inference (O(N^3)) with Kalman or Bayesian filter type of methods (O(N)), which give us the interpretability of the GP formulation (or regularized regression) and the efficiency of the filtering approach.
Finally we will overview some clever ideas on how to speed up large distributed models using emulators constructed with the methods mentioned above.