filmov
tv
Demystifying the Black Box: Towards Explainable and Controllable Neural Networks
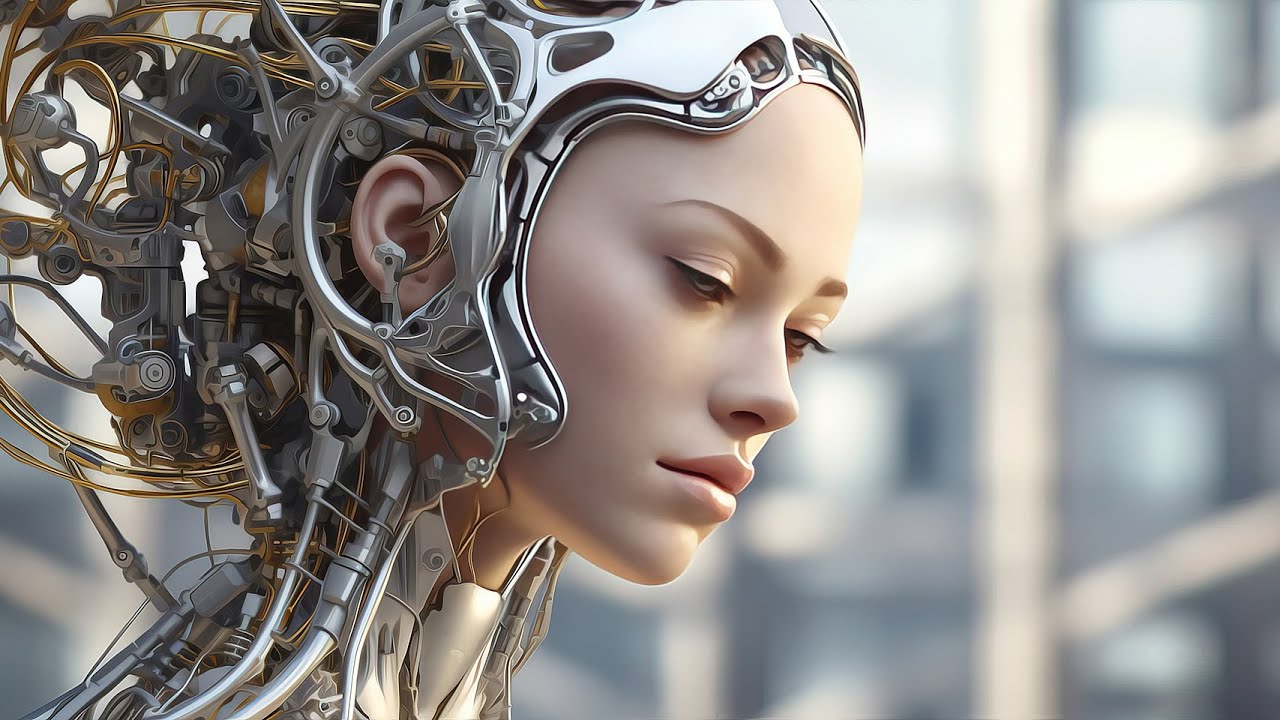
Показать описание
Author: #pooyanghamari
Have you ever thought about how neural networks see us? Perhaps so? Or so? Or like this? Interesting isn't it?
#neuralnetworks recognize us as numbers. After all, everything in this world is numbers.
Introduction to Neural Networks
Neural networks, inspired by the structure and function of the human brain, are a fundamental building block of artificial intelligence. These interconnected networks of artificial neurons are capable of learning from data, recognizing patterns, and making predictions with remarkable accuracy and efficiency. From image recognition and natural language processing to autonomous vehicles and medical diagnosis, neural networks have revolutionized countless applications across diverse industries.
Complexity and Non-Linearity
One of the primary reasons why controlling neural networks is challenging lies in their inherent complexity and non-linearity. Neural networks consist of thousands, or even millions, of interconnected neurons and layers, each with its own set of parameters and connections. The interactions between these neurons are highly complex and non-linear, making it difficult to predict how changes to one part of the network will affect its overall behavior.
Emergent Properties and Unintended Consequences
As a result of their complexity, neural networks often exhibit emergent properties – behaviors and patterns that arise from the interactions of individual components but are not explicitly programmed into the system. These emergent properties can lead to unexpected outcomes and unintended consequences, making it challenging to anticipate and control the behavior of neural networks, especially in complex and dynamic environments.
Black Box Nature
Another factor that complicates the control of neural networks is their 'black box' nature – the inability to fully understand or interpret the internal workings of the network. While neural networks are highly effective at learning and making predictions, the mechanisms underlying their decision-making processes can be opaque and difficult to interpret. This lack of transparency makes it challenging to diagnose and correct errors or biases in the network's behavior.
Training and Learning Dynamics
Neural networks learn and adapt through a process known as training, where they are exposed to large amounts of data and adjust their internal parameters to minimize errors and improve performance. However, the training process is highly dependent on the quality and quantity of the training data, as well as the choice of optimization algorithms and hyperparameters. Even small changes to these factors can have significant impacts on the network's behavior, making it difficult to achieve consistent and reliable control.
Adversarial Attacks and Robustness
Neural networks are also susceptible to adversarial attacks – deliberate manipulations of input data designed to deceive or mislead the network's predictions. These attacks can exploit vulnerabilities in the network's architecture or training data, leading to erroneous or unexpected outcomes. Ensuring the robustness and resilience of neural networks against adversarial attacks is a significant challenge that further complicates efforts to control their behavior.
Ethical and Societal Implications
The inability to fully control neural networks raises important ethical and societal implications for the deployment of artificial intelligence in various domains. Issues such as algorithmic bias, fairness, and accountability become more pronounced when dealing with systems that exhibit complex and unpredictable behavior. As neural networks become increasingly integrated into critical systems and decision-making processes, ensuring transparency, accountability, and ethical use of #AItechnologies becomes paramount.
The Future of Neural Networks
While achieving full control over neural networks may remain a formidable challenge, ongoing research and innovation offer hope for addressing some of the underlying complexities and limitations of these systems. Techniques such as explainable #AI, robust optimization, and adversarial training are emerging as promising approaches for improving the interpretability, robustness, and controllability of neural networks. By continuing to push the boundaries of AI research and development, we can work towards harnessing the full potential of neural networks while mitigating the risks and challenges they pose.
In conclusion, the quest to control neural networks is a journey fraught with complexity, uncertainty, and discovery. As we navigate this fascinating frontier of artificial intelligence, let us approach the challenges and opportunities with humility, curiosity, and a commitment to advancing our understanding of these powerful systems. Together, let us explore the mysteries of neural networks and unlock their transformative potential for the betterment of humanity.
Have you ever thought about how neural networks see us? Perhaps so? Or so? Or like this? Interesting isn't it?
#neuralnetworks recognize us as numbers. After all, everything in this world is numbers.
Introduction to Neural Networks
Neural networks, inspired by the structure and function of the human brain, are a fundamental building block of artificial intelligence. These interconnected networks of artificial neurons are capable of learning from data, recognizing patterns, and making predictions with remarkable accuracy and efficiency. From image recognition and natural language processing to autonomous vehicles and medical diagnosis, neural networks have revolutionized countless applications across diverse industries.
Complexity and Non-Linearity
One of the primary reasons why controlling neural networks is challenging lies in their inherent complexity and non-linearity. Neural networks consist of thousands, or even millions, of interconnected neurons and layers, each with its own set of parameters and connections. The interactions between these neurons are highly complex and non-linear, making it difficult to predict how changes to one part of the network will affect its overall behavior.
Emergent Properties and Unintended Consequences
As a result of their complexity, neural networks often exhibit emergent properties – behaviors and patterns that arise from the interactions of individual components but are not explicitly programmed into the system. These emergent properties can lead to unexpected outcomes and unintended consequences, making it challenging to anticipate and control the behavior of neural networks, especially in complex and dynamic environments.
Black Box Nature
Another factor that complicates the control of neural networks is their 'black box' nature – the inability to fully understand or interpret the internal workings of the network. While neural networks are highly effective at learning and making predictions, the mechanisms underlying their decision-making processes can be opaque and difficult to interpret. This lack of transparency makes it challenging to diagnose and correct errors or biases in the network's behavior.
Training and Learning Dynamics
Neural networks learn and adapt through a process known as training, where they are exposed to large amounts of data and adjust their internal parameters to minimize errors and improve performance. However, the training process is highly dependent on the quality and quantity of the training data, as well as the choice of optimization algorithms and hyperparameters. Even small changes to these factors can have significant impacts on the network's behavior, making it difficult to achieve consistent and reliable control.
Adversarial Attacks and Robustness
Neural networks are also susceptible to adversarial attacks – deliberate manipulations of input data designed to deceive or mislead the network's predictions. These attacks can exploit vulnerabilities in the network's architecture or training data, leading to erroneous or unexpected outcomes. Ensuring the robustness and resilience of neural networks against adversarial attacks is a significant challenge that further complicates efforts to control their behavior.
Ethical and Societal Implications
The inability to fully control neural networks raises important ethical and societal implications for the deployment of artificial intelligence in various domains. Issues such as algorithmic bias, fairness, and accountability become more pronounced when dealing with systems that exhibit complex and unpredictable behavior. As neural networks become increasingly integrated into critical systems and decision-making processes, ensuring transparency, accountability, and ethical use of #AItechnologies becomes paramount.
The Future of Neural Networks
While achieving full control over neural networks may remain a formidable challenge, ongoing research and innovation offer hope for addressing some of the underlying complexities and limitations of these systems. Techniques such as explainable #AI, robust optimization, and adversarial training are emerging as promising approaches for improving the interpretability, robustness, and controllability of neural networks. By continuing to push the boundaries of AI research and development, we can work towards harnessing the full potential of neural networks while mitigating the risks and challenges they pose.
In conclusion, the quest to control neural networks is a journey fraught with complexity, uncertainty, and discovery. As we navigate this fascinating frontier of artificial intelligence, let us approach the challenges and opportunities with humility, curiosity, and a commitment to advancing our understanding of these powerful systems. Together, let us explore the mysteries of neural networks and unlock their transformative potential for the betterment of humanity.