filmov
tv
Neural Implicit Flow (NIF) [Physics Informed Machine Learning]
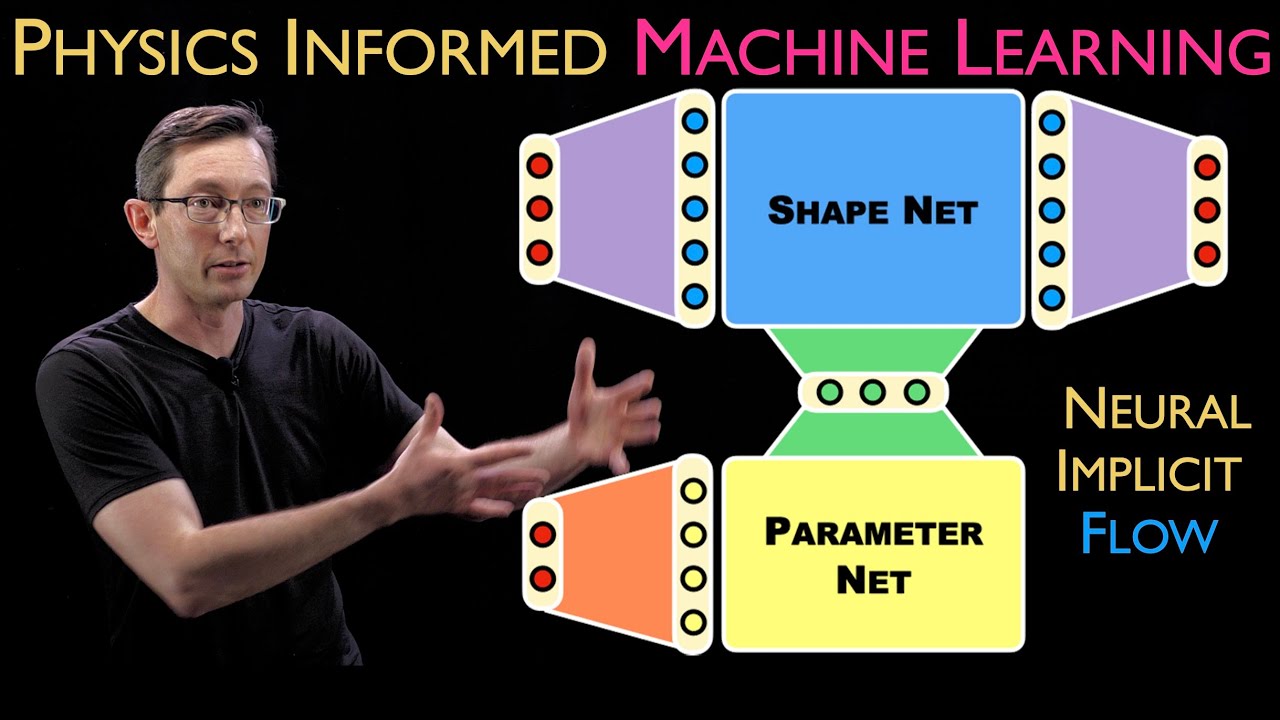
Показать описание
This video was produced at the University of Washington, and we acknowledge funding support from the Boeing Company
%%% CHAPTERS %%%
00:00 Intro
01:25 Underlying Concept
// 02:32 Example Problem
04:36 Example Application: Turbulent Data Compression
06:23 Example Application: Sparse Sensor Placement
08:09 NIF is Mesh Agnostic
10:30 Results/Benchmark Data
// 11:00 Growing Vortices/ Cool Pictures
11:40 Shape Net Architectures
12:30 Outro
%%% CHAPTERS %%%
00:00 Intro
01:25 Underlying Concept
// 02:32 Example Problem
04:36 Example Application: Turbulent Data Compression
06:23 Example Application: Sparse Sensor Placement
08:09 NIF is Mesh Agnostic
10:30 Results/Benchmark Data
// 11:00 Growing Vortices/ Cool Pictures
11:40 Shape Net Architectures
12:30 Outro
Комментарии