filmov
tv
Backpropagation explained | Part 5 - What puts the 'back' in backprop?
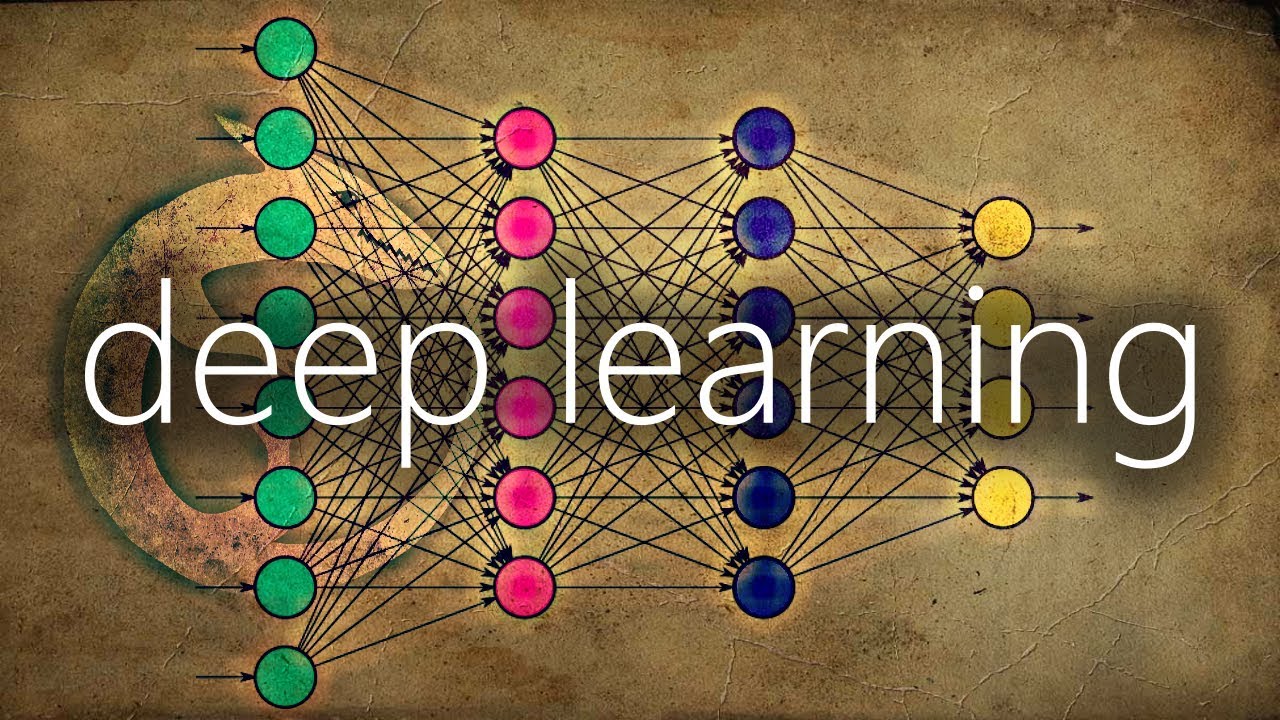
Показать описание
Let's see the math that explains how backpropagation works backwards through a neural network.
We've seen how to calculate the gradient of the loss function using backpropagation in the previous video. We haven't yet seen though where the backwards movement comes into play that we talked about when we discussed the intuition for backprop.
So now, we're going to build on the knowledge that we've already developed to understand what exactly puts the back in backpropagation. The explanation we'll give for this will be math-based, so we're first going to start out by exploring the motivation needed for us to understand the calculations we'll be working through.
We'll then jump right into the calculations, which, we'll see, are actually quite similar to ones we've worked through in the previous video.
After we've got the math down, we'll then bring everything together to achieve the mind-blowing realization for how these calculations are mathematically done in a backwards fashion.
🕒🦎 VIDEO SECTIONS 🦎🕒
00:43 Agenda
01:13 Calculations - Derivative of the loss with respect to activation outputs
13:06 Summary
13:40 Collective Intelligence and the DEEPLIZARD HIVEMIND
💥🦎 DEEPLIZARD COMMUNITY RESOURCES 🦎💥
👋 Hey, we're Chris and Mandy, the creators of deeplizard!
👉 Check out the website for more learning material:
💻 ENROLL TO GET DOWNLOAD ACCESS TO CODE FILES
🧠 Support collective intelligence, join the deeplizard hivemind:
🧠 Use code DEEPLIZARD at checkout to receive 15% off your first Neurohacker order
👉 Use your receipt from Neurohacker to get a discount on deeplizard courses
👀 CHECK OUT OUR VLOG:
❤️🦎 Special thanks to the following polymaths of the deeplizard hivemind:
Tammy
Mano Prime
Ling Li
🚀 Boost collective intelligence by sharing this video on social media!
👀 Follow deeplizard:
🎓 Deep Learning with deeplizard:
🎓 Other Courses:
🛒 Check out products deeplizard recommends on Amazon:
🎵 deeplizard uses music by Kevin MacLeod
❤️ Please use the knowledge gained from deeplizard content for good, not evil.
We've seen how to calculate the gradient of the loss function using backpropagation in the previous video. We haven't yet seen though where the backwards movement comes into play that we talked about when we discussed the intuition for backprop.
So now, we're going to build on the knowledge that we've already developed to understand what exactly puts the back in backpropagation. The explanation we'll give for this will be math-based, so we're first going to start out by exploring the motivation needed for us to understand the calculations we'll be working through.
We'll then jump right into the calculations, which, we'll see, are actually quite similar to ones we've worked through in the previous video.
After we've got the math down, we'll then bring everything together to achieve the mind-blowing realization for how these calculations are mathematically done in a backwards fashion.
🕒🦎 VIDEO SECTIONS 🦎🕒
00:43 Agenda
01:13 Calculations - Derivative of the loss with respect to activation outputs
13:06 Summary
13:40 Collective Intelligence and the DEEPLIZARD HIVEMIND
💥🦎 DEEPLIZARD COMMUNITY RESOURCES 🦎💥
👋 Hey, we're Chris and Mandy, the creators of deeplizard!
👉 Check out the website for more learning material:
💻 ENROLL TO GET DOWNLOAD ACCESS TO CODE FILES
🧠 Support collective intelligence, join the deeplizard hivemind:
🧠 Use code DEEPLIZARD at checkout to receive 15% off your first Neurohacker order
👉 Use your receipt from Neurohacker to get a discount on deeplizard courses
👀 CHECK OUT OUR VLOG:
❤️🦎 Special thanks to the following polymaths of the deeplizard hivemind:
Tammy
Mano Prime
Ling Li
🚀 Boost collective intelligence by sharing this video on social media!
👀 Follow deeplizard:
🎓 Deep Learning with deeplizard:
🎓 Other Courses:
🛒 Check out products deeplizard recommends on Amazon:
🎵 deeplizard uses music by Kevin MacLeod
❤️ Please use the knowledge gained from deeplizard content for good, not evil.
Комментарии