filmov
tv
Carlo Lucibello - Entropic algorithms and wide flat minima in neural networks
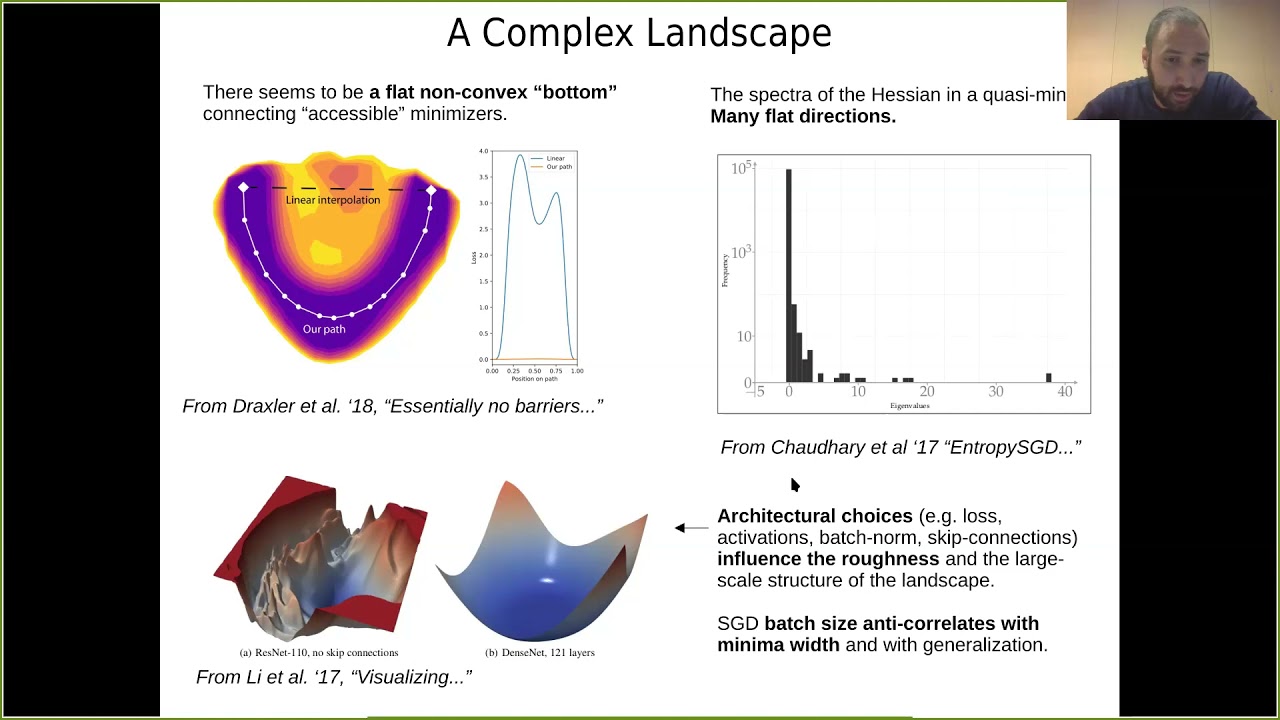
Показать описание
Entropic algorithms and wide flat minima in neural networks
Carlo Lucibello
13.00-14.00, Wednesday 3 November 2021, Zoom
Abstract: The properties of flat minima in the training loss landscape of neural networks have been debated for some time. Increasing evidence suggests they possess better generalization capabilities with respect to sharp ones. First, we'll discuss simple neural network models. Using analytical tools from spin glass theory of disordered systems, we are able to probe the geometry of the landscape and highlight the presence of flat minima that generalize well and are attractive for learning dynamics.
Next, we extend the analysis to the deep learning scenario by extensive numerical validations. Using two algorithms, Entropy- SGD and Replicated-SGD, that explicitly include in the optimization objective a non-local flatness measure known as local entropy, we consistently improve the generalization error for common architectures (e.g. ResNet, EfficientNet). Finally, we'll discuss the extension of message passing techniques (Belief Propagation) to deep networks as an alternative paradigm to SGD training.
Carlo Lucibello
13.00-14.00, Wednesday 3 November 2021, Zoom
Abstract: The properties of flat minima in the training loss landscape of neural networks have been debated for some time. Increasing evidence suggests they possess better generalization capabilities with respect to sharp ones. First, we'll discuss simple neural network models. Using analytical tools from spin glass theory of disordered systems, we are able to probe the geometry of the landscape and highlight the presence of flat minima that generalize well and are attractive for learning dynamics.
Next, we extend the analysis to the deep learning scenario by extensive numerical validations. Using two algorithms, Entropy- SGD and Replicated-SGD, that explicitly include in the optimization objective a non-local flatness measure known as local entropy, we consistently improve the generalization error for common architectures (e.g. ResNet, EfficientNet). Finally, we'll discuss the extension of message passing techniques (Belief Propagation) to deep networks as an alternative paradigm to SGD training.