filmov
tv
Graphical Models: A Combinatorial and Geometric Perspective
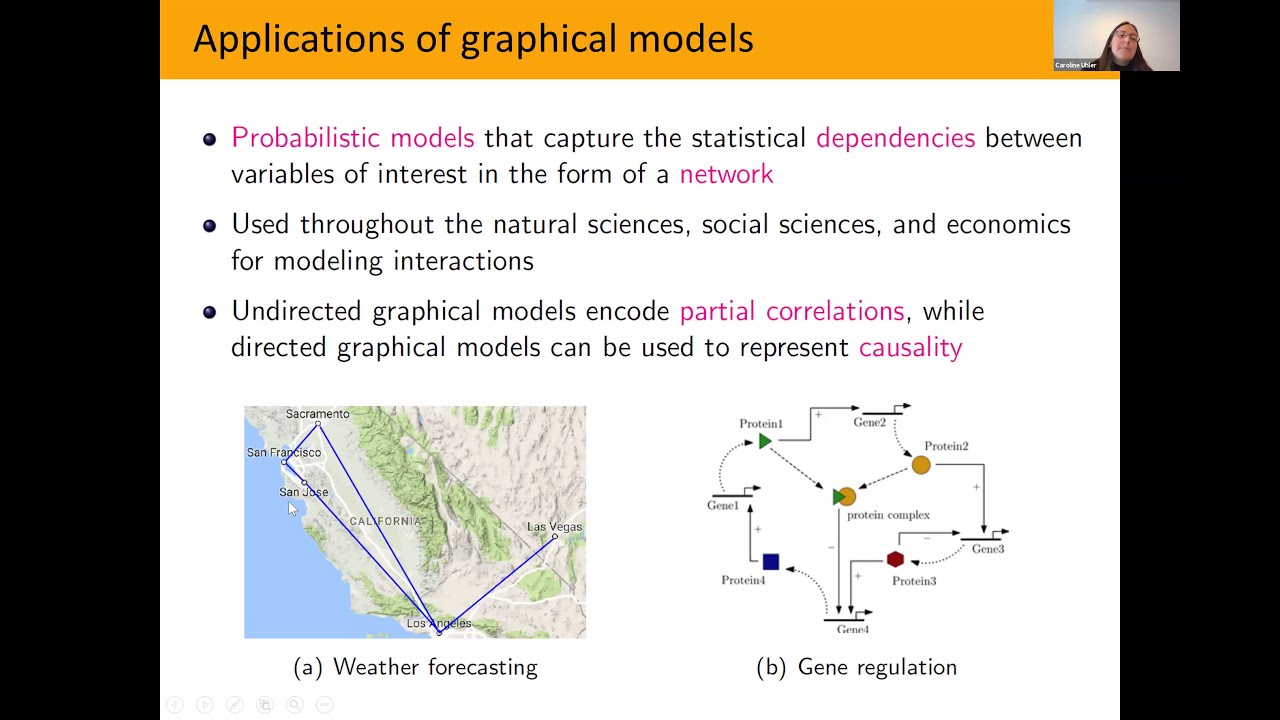
Показать описание
Caroline Uhler, MIT
Winter School on Geometric Constraint Systems
Abstract: Graphical models are used throughout the natural sciences, social sciences, and economics to model statistical relationships between variables of interest. When studying graphical models, combinatorial and geometric constraints naturally arise and their understanding is required to advance the statistical methodology. The first lecture introduces graphical models, providing the foundations to connect networks with probability distributions. The second lecture discusses undirected graphical models and additional structure such as total positivity and its impact on structure learning. The last lecture discusses causality and the inherent combinatorial and geometric constraints underlying causal structure discovery.
Winter School on Geometric Constraint Systems
Abstract: Graphical models are used throughout the natural sciences, social sciences, and economics to model statistical relationships between variables of interest. When studying graphical models, combinatorial and geometric constraints naturally arise and their understanding is required to advance the statistical methodology. The first lecture introduces graphical models, providing the foundations to connect networks with probability distributions. The second lecture discusses undirected graphical models and additional structure such as total positivity and its impact on structure learning. The last lecture discusses causality and the inherent combinatorial and geometric constraints underlying causal structure discovery.
Graphical Models: A Combinatorial and Geometric Perspective
Graphical Models: A Combinatorial and Geometric Perspective (Lecture 2)
Graphical Models: A Combinatorial and Geometric Perspective (Lecture 3)
Networks and Graphical Models
Salar Fattahi: Scalable Inference of Dynamic Graphical Models with Combinatorial Structures
Lecture 1, Advanced Inference in Graphical Models
An Efficient Graph Generative Model for Navigating Ultra-Large Combinatorial Synthesis Libraries
Connections between graphical models and rigidity theory
Tensor Graphical Models For Complex And High Dimensional Data
[MISS 2016] Carsten Rother - Introduction to Graphical Models
Roman Schutski: Graphical models for tensor networks and machine learning
Biological Models with Combinatorial Spaces
Can We Learn Heuristics for Graphical Model Inference Using Reinforcement Learning?
Nonconvex Sparse Graph Learning under Laplacian-structured Graphical Models
Deep Dive into Combinatorics (Introduction)
CIMPA_2024_Dr. Cordian Riener_Tutorial_02
July 22nd 2 Tutorial Composing graphical models with neural networks
Frederic Koehler (MIT) -- Learning Some Popular Gaussian Graphical Models
Rigidity theory for Gaussian graphical models: the maximum likelihood threshold of a graph
Sparse plus low-rank graphical models of time series for functional connectivity in MEG
Directed Graphical Models for Extreme Value Statistics
Random Combinatorial Matrices
Information Theoretic Optimal Learning of Gaussian Graphical Models
Roman Schutski: Graphical models for tensor networks and machine learning
Комментарии