filmov
tv
Simple Deep Neural Networks for Text Classification
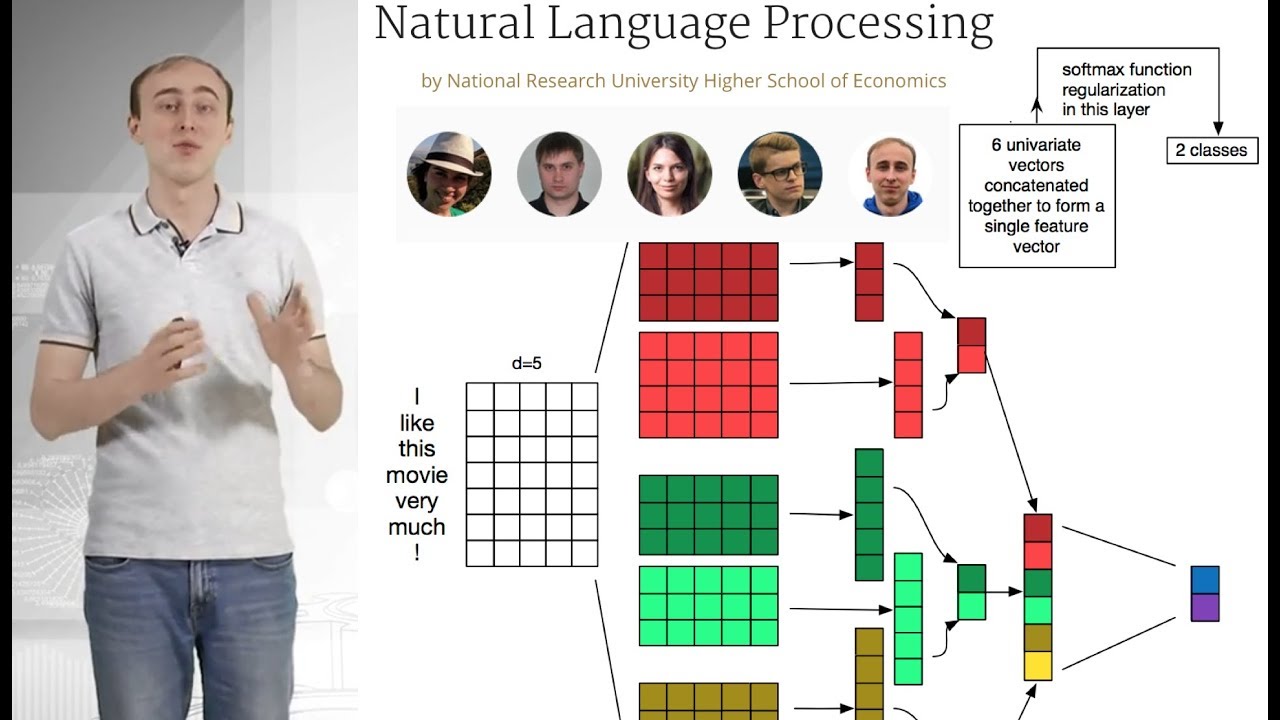
Показать описание
Hi. In this video, we will apply neural networks for text. And let's first remember, what is text? You can think of it as a sequence of characters, words or anything else. And in this video, we will continue to think of text as a sequence of words or tokens. And let's remember how bag of words works. You have every word and forever distinct word that you have in your dataset, you have a feature column. And you actually effectively vectorizing each word with one-hot-encoded vector that is a huge vector of zeros that has only one non-zero value which is in the column corresponding to that particular word. So in this example, we have very, good, and movie, and all of them are vectorized independently. And in this setting, you actually for real world problems, you have like hundreds of thousands of columns. And how do we get to bag of words representation? You can actually see that we can sum up all those values, all those vectors, and we come up with a bag of words vectorization that now corresponds to very, good, movie. And so, it could be good to think about bag of words representation as a sum of sparse one-hot-encoded vectors corresponding to each particular word. Okay, let's move to neural network way. And opposite to the sparse way that we've seen in bag of words, in neural networks, we usually like dense representation. And that means that we can replace each word by a dense vector that is much shorter. It can have 300 values, and now it has any real valued items in those vectors. And an example of such vectors is word2vec embeddings, that are pretrained embeddings that are done in an unsupervised manner. And we will actually dive into details on word2vec in the next two weeks. But, all we have to know right now is that, word2vec vectors have a nice property. Words that have similar context in terms of neighboring words, they tend to have vectors that are collinear, that actually point to roughly the same direction. And that is a very nice property that we will further use. Okay, so, now we can replace each word with a dense vector of 300 real values. What do we do next? How can we come up with a feature descriptor for the whole text? Actually, we can use the same manner as we used for bag of words. We can just dig the sum of those vectors and we have a representation based on word2vec embeddings for the whole text, like very good movie. And, that's some of word2vec vectors actually works in practice. It can give you a great baseline descriptor, a baseline features for your classifier and that can actually work pretty well. Another approach is doing a neural network over these embeddings.
Комментарии