filmov
tv
Residual Networks (ResNet) [Physics Informed Machine Learning]
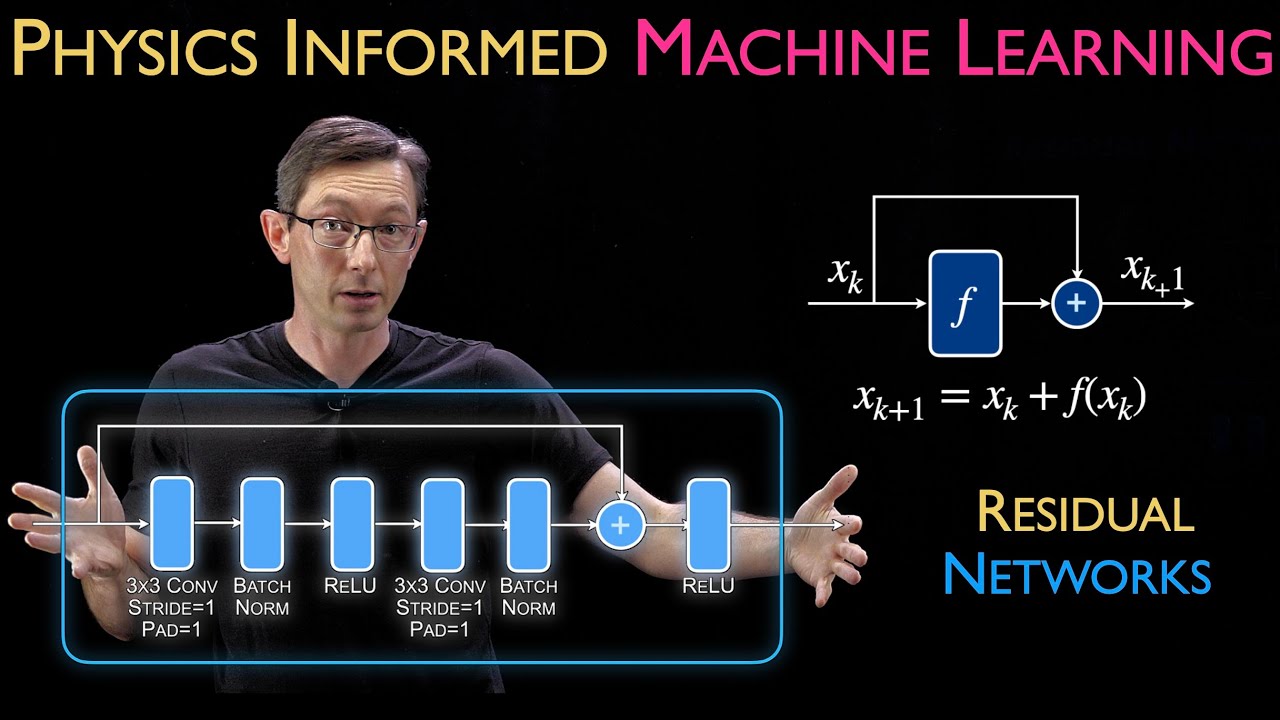
Показать описание
This video discusses Residual Networks, one of the most popular machine learning architectures that has enabled considerably deeper neural networks through jump/skip connections. This architecture mimics many of the aspects of a numerical integrator.
This video was produced at the University of Washington, and we acknowledge funding support from the Boeing Company
%%% CHAPTERS %%%
00:00 Intro
01:09 Concept: Modeling the Residual
03:26 Building Blocks
05:59 Motivation: Deep Network Signal Loss
07:43 Extending to Classification
09:00 Extending to DiffEqs
10:16 Impact of CVPR and Resnet
12:17 Resnets and Euler Integrators
13:34 Neural ODEs and Improved Integrators
16:07 Outro
This video was produced at the University of Washington, and we acknowledge funding support from the Boeing Company
%%% CHAPTERS %%%
00:00 Intro
01:09 Concept: Modeling the Residual
03:26 Building Blocks
05:59 Motivation: Deep Network Signal Loss
07:43 Extending to Classification
09:00 Extending to DiffEqs
10:16 Impact of CVPR and Resnet
12:17 Resnets and Euler Integrators
13:34 Neural ODEs and Improved Integrators
16:07 Outro
Residual Networks (ResNet) [Physics Informed Machine Learning]
Neural ODEs (NODEs) [Physics Informed Machine Learning]
A Hands-on Introduction to Physics-informed Machine Learning
AI/ML+Physics Part 3: Designing an Architecture [Physics Informed Machine Learning]
AI/ML+Physics Part 1: Choosing what to model [Physics Informed Machine Learning]
DataLearning: Physics-Informed Neural Networks in Medicine
Hamed Saidaoui, Deep Nurbs : Physics-Informed Neural Networks for Complex Geometries
Janaki Vamaraju: FResNet++: Augmenting Deep Residual Surrogates with Fourier Neural Operators...
ETH Zürich DLSC: Introduction to Deep Learning Part 1
A. Subramaniam - Leveraging physics information in neural networks for fluid flow problems
Neural Differential Equations
Robust learning with implicit residual networks
DeepOnet: Learning nonlinear operators based on the universal approximation theorem of operators.
DDPS | Physics-Informed Learning for Nonlinear Dynamical Systems
Continuous-in-Depth Neural Networks
DataLearning: Physics-Informed Deep Learning - Learning from Small Data
SoK: How Robust is Image Classification Deep Neural Network Watermarking?
What are Autoencoders?
Introduction to Week 9 - From Linear Models to Neural Networks
MSML2020 Invited Talk by Prof. George Karniadakis, Brown University
Physics Informed Attention Temporal Convolutional Network for EEG Based Motor Imagery Classification
How to use backpropagation to create a neural network out of any physical system - Peter McMahon
DeepXDE: A Deep Learning Library for Solving Differential Equations by Lu Lu
Machine Learning for Computational Fluid Dynamics
Комментарии