filmov
tv
RecSys 2016: Paper Session 1 - A Scalable Approach for Periodical Personalized Recommendations
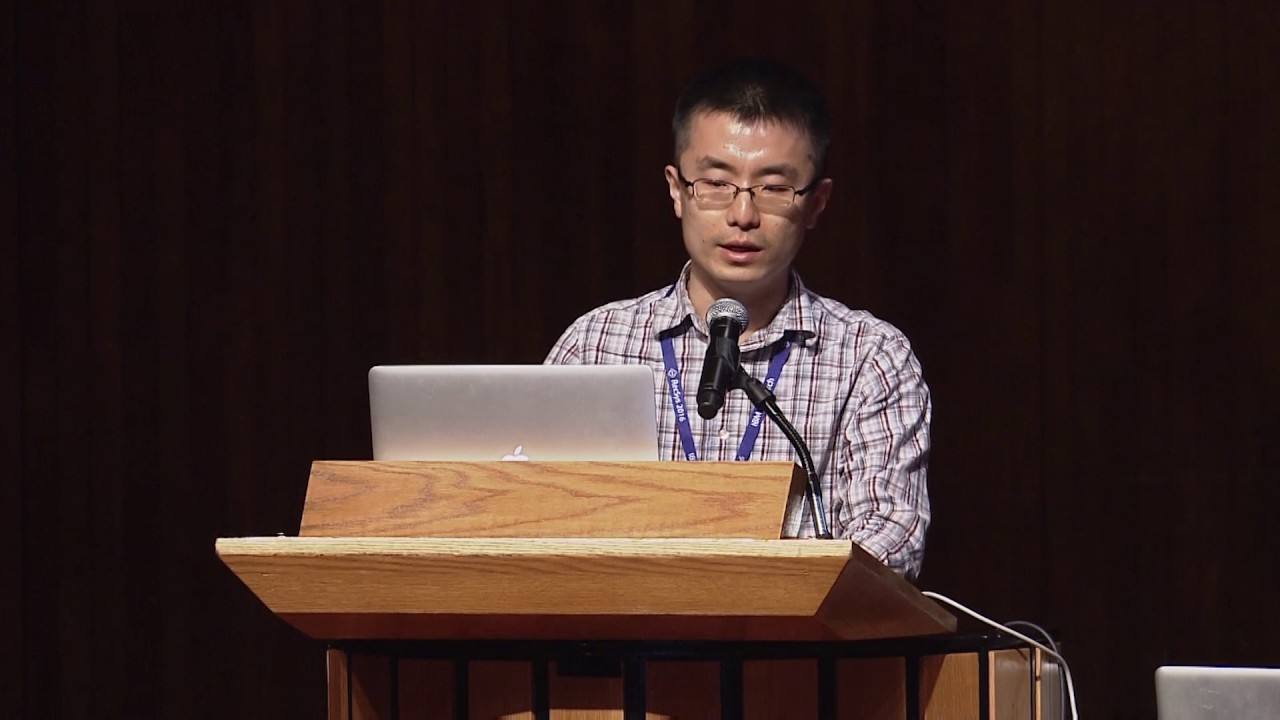
Показать описание
Zhen Qin, Ish Rishabh, John Carnahan
We develop a highly scalable and effective contextual bandit approach towards periodical personalized recommendations. The online bootstrapping-based technique provides a principled way for UCB-type exploitation-exploration algorithms, while being able to handle arbitrary sized datasets, well suited to learn the ever evolving user preference drift from streaming data, and essentially parameter-free. We further introduce techniques to handle arbitrary sized feature spaces using feature hashing, leverage existing state-of-art machine learning via learning reduction, and increase cache hits by managing bootstrapped models in memory effectively. The resulted model trains on millions of examples and billions of features within minutes on a single personal computer. It shows persistent performance in both offline and online evaluation. We observe around 10\% click through rate (CTR) and conversion lift over a collaborative filtering approach in real-world A/B testing across more than 40 million users on the major Ticketmaster email recommendation product.
We develop a highly scalable and effective contextual bandit approach towards periodical personalized recommendations. The online bootstrapping-based technique provides a principled way for UCB-type exploitation-exploration algorithms, while being able to handle arbitrary sized datasets, well suited to learn the ever evolving user preference drift from streaming data, and essentially parameter-free. We further introduce techniques to handle arbitrary sized feature spaces using feature hashing, leverage existing state-of-art machine learning via learning reduction, and increase cache hits by managing bootstrapped models in memory effectively. The resulted model trains on millions of examples and billions of features within minutes on a single personal computer. It shows persistent performance in both offline and online evaluation. We observe around 10\% click through rate (CTR) and conversion lift over a collaborative filtering approach in real-world A/B testing across more than 40 million users on the major Ticketmaster email recommendation product.