filmov
tv
Importance Weighted Hierarchical Variational Inference
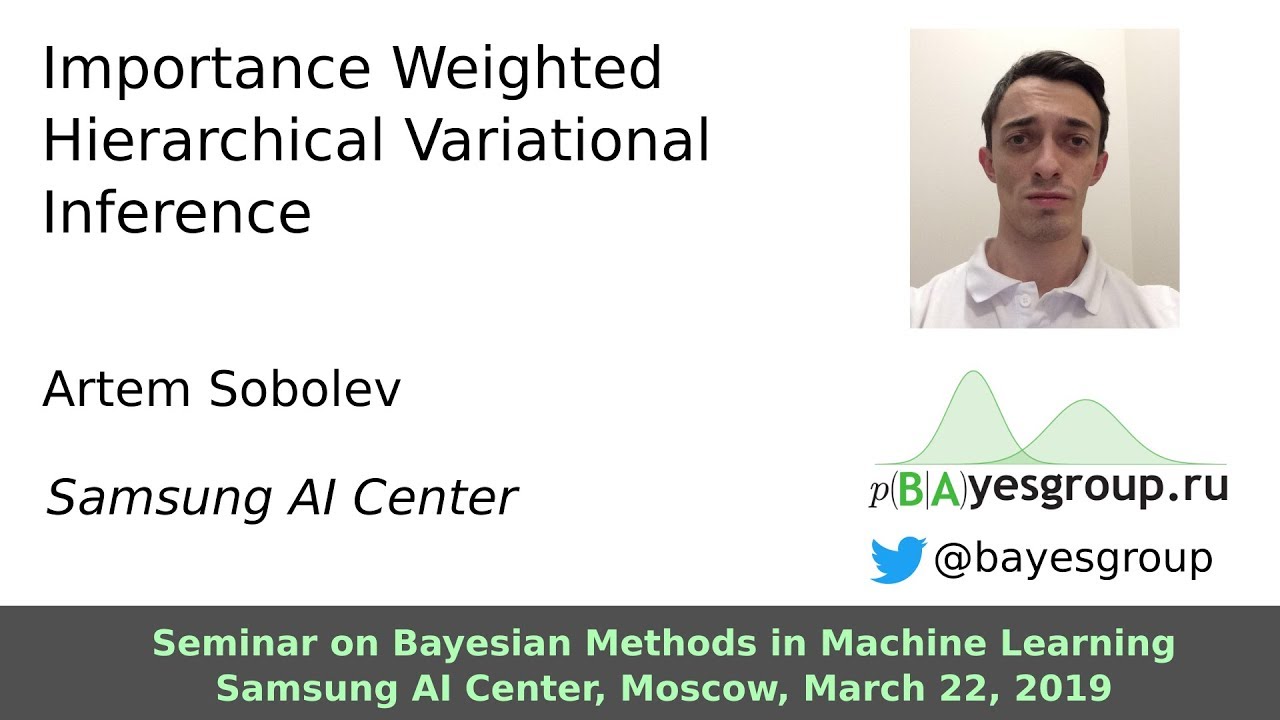
Показать описание
Variational Inference is a powerful tool in the Bayesian modeling toolkit, however, its effectiveness is determined by the expressivity of the utilized variational distributions in terms of their ability to match the true posterior distribution. Recently, there's been a lot of work on employing neural networks as powerful sample generators, but the need for a tractable density is a major limitation. In talk I will suggest a novel method based on a multisample variational bound that generalizes many previous works, most importantly, Hierarchical Variational Models and Semi-Implicit Variational Inference. The bound allows us learn more expressive approximate posteriors, and can be combined with many prior results.