filmov
tv
14: Distributed Logging & Metrics Framework | Systems Design Interview Questions With Ex-Google SWE
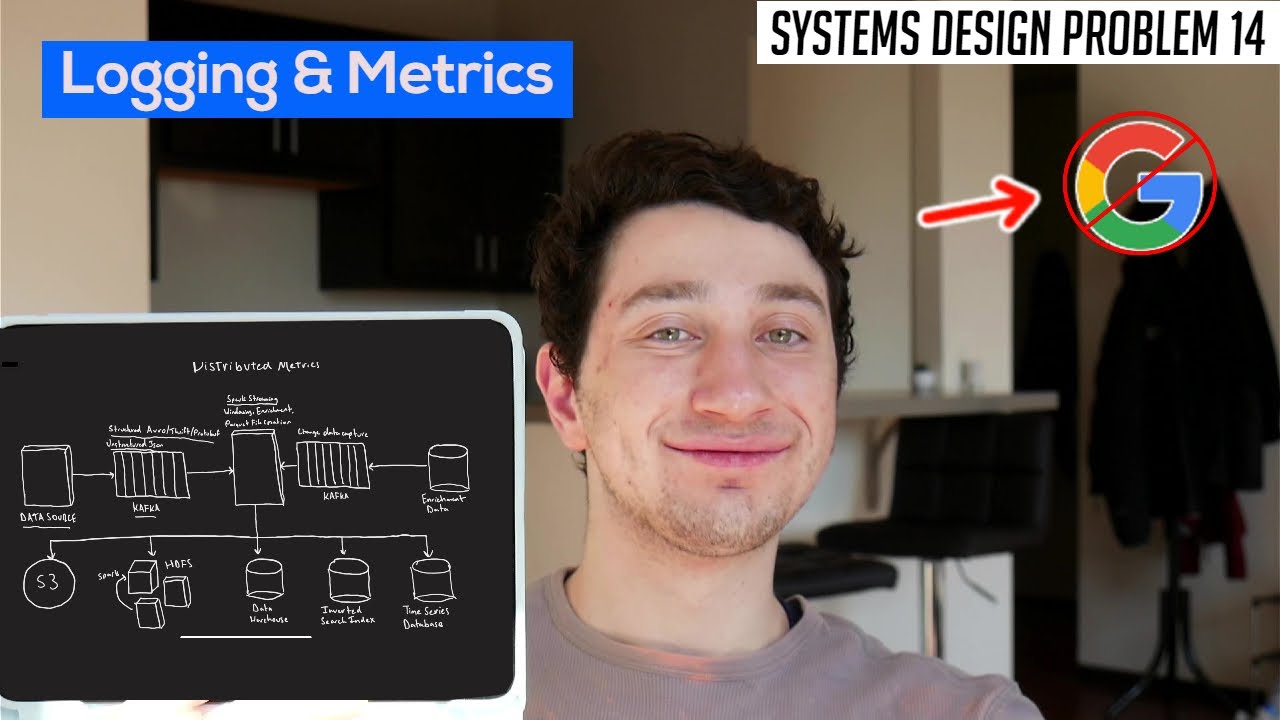
Показать описание
200 videos and we're still talking about logs - thanks guys, you're the best
14: Distributed Logging & Metrics Framework | Systems Design Interview Questions With Ex-Google ...
Distributed Metrics/Logging Design Deep Dive with Google SWE! | Systems Design Interview Question 14
System Design Mock Interview - Design distributed metrics logging system
System Design Fundamentals | Pt 10 | Logging, Metrics, Automation, and Monitoring
Logs, Metrics, and APM: The Holy Trinity of Ops - Thomas Watson
Effective troubleshooting with Grafana Loki - query basics
Distributed Logging & Metrics System Design | Microservices Logging | Distributed Systems in Hin...
7. Observability Coordinated: Prometheus Exemplars (Metrics) — Grafana Tempo (Traces) — Loki (Logs)...
Top 7 Most-Used Distributed System Patterns
OpenShift 3.1 Logging & Metrics Overview
Advanced Techniques for AWS Monitoring, Metrics and Logging
Task - 14 - GCP | Nodejs App | Cloud Logging | Log Based Metric | Dashboard | Ship to BigQuery
Fluent Bit 3.0 Observability: Elevating Logs, Metrics, and Traces!
time and work | time and work tricks | #shorts #short #shortvideo #viral
Microservices Logging | ELK Stack | Elastic Search | Logstash | Kibana | JavaTechie
How to do Structured Logging and Custom Metrics in your Serverless Applications?
OpenTelemetry Course - Understand Software Performance
Performance Metrics | System Design Tutorials | Lecture 14 | 2020
Unified Go-To-Market 14 | GTM Bloat and Financial Metrics in B2B SaaS Companies
Monitoring baseline on k3d: logs, metrics, ingress and testing the Linkerd service mesh
How Prometheus Monitoring works | Prometheus Architecture explained
Design a metrics monitoring system
Unified Logs and Metrics Demo
SREcon21 - Taking Control of Metrics Growth and Cardinality: Tips for Maximizing Your Observability
Комментарии