filmov
tv
Predictive Profiling of SARS-CoV-2 Variants by Deep Mutational Learning | Sai Reddy
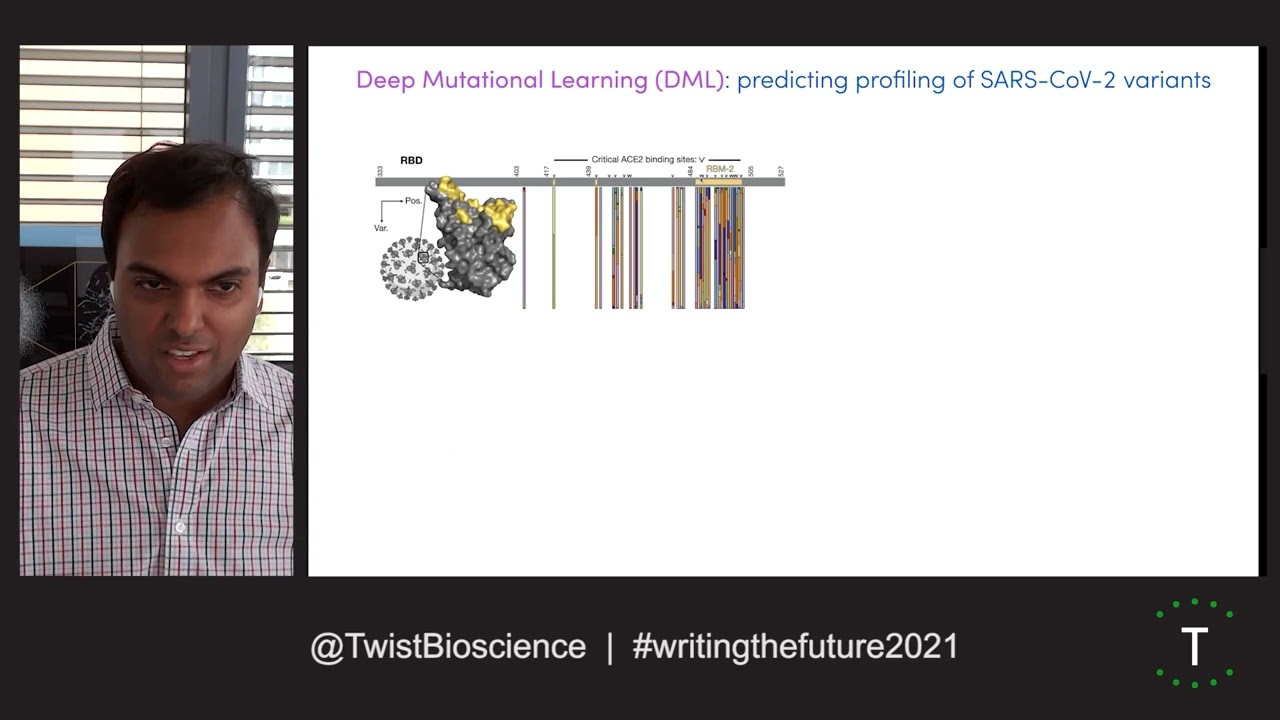
Показать описание
The continual evolution of the severe acute respiratory syndrome coronavirus-2 (SARS-CoV-2) and the emergence of variants that show resistance to vaccines and neutralizing antibodies threatens to prolong the coronavirus disease 2019 (COVID-19) pandemic. Selection and emergence of SARS-CoV-2 variants are driven in part by mutations within the viral spike protein and in particular, the receptor-binding domain (RBD), which binds to the human ACE2 receptor and is a primary target site for neutralizing antibodies. Comprehensive mapping of single-position substitutions in the RBD has revealed key mutations that enhance binding to ACE2 and provide an escape from neutralizing antibodies. However, several SARS-CoV-2 variants such as Beta (B.1.351) Gamma (P.1) and Delta (B.1.617.2) possess multiple, combinatorial mutations in their RBD. Here, we develop deep mutational learning (DML) — a machine learning-guided protein engineering technology — that enables the comprehensive interrogation of combinatorial mutations in the RBD and prediction of their impact on ACE2 binding and antibody escape. DML reveals a highly diverse sequence landscape of possible variants that maintain or enhance binding to ACE2 and escape from different classes of neutralizing antibodies. DML may be used in the future to comprehensively profile the breadth of candidate therapeutic antibodies against existing and prospective variants of SARS-CoV-2.