filmov
tv
Allen Downey: Bayesian Decision Analysis [Tutorial] | PyData Global 2022
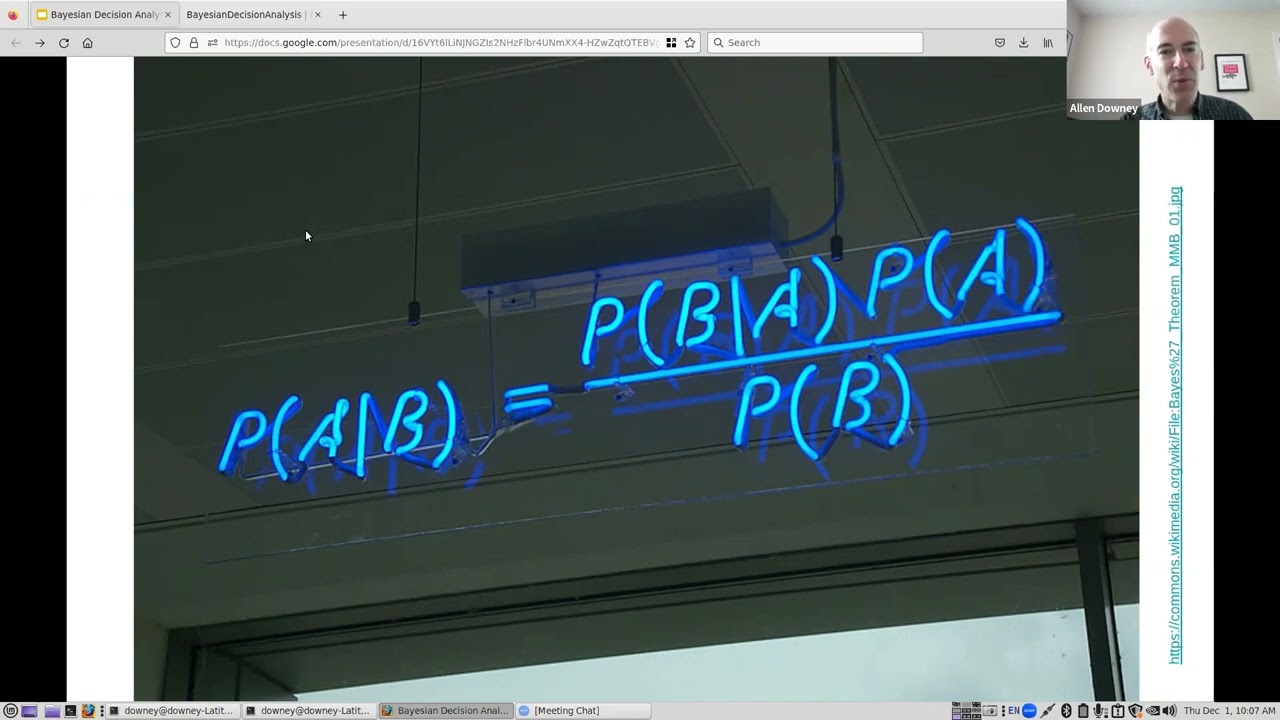
Показать описание
This tutorial is a hands-on introduction to Bayesian Decision Analysis (BDA), which is a framework for using probability to guide decision-making under uncertainty. I start with Bayes's Theorem, which is the foundation of Bayesian statistics, and work toward the Bayesian bandit strategy, which is used for A/B testing, medical tests, and related applications. For each step, I provide a Jupyter notebook where you can run Python code and work on exercises. In addition to the bandit strategy, I summarize two other applications of BDA, optimal bidding and deriving a decision rule. Finally, I suggest resources you can use to learn more.
Outline * Problem statement: A/B testing, medical tests, and the Bayesian bandit problem * Prerequisites and goals * Bayes's theorem and the five urn problem * Using Pandas to represent a PMF * Notebook 1: Estimating proportions * From belief to strategy: Thompson sampling * Notebook 2: Implementing and testing Thompson sampling * Debrief: why Bayesian decision analysis is better * More generally: two other examples of BDA * Resources and next steps
Prerequisites
For this tutorial, you should be familiar with Python at an intermediate level. We'll use NumPy, SciPy, and Pandas, but I'll explain what you need to know as we go. You should be familiar with basic probability, but you don't need to know anything about Bayesian statistics.
I'll provide Jupyter notebooks that run on Colab, so you don't have to install anything or prepare ahead of time. But you should be familiar with Jupyter notebooks.
Bio:
Allen Downey
Allen Downey is a Staff Scientist at DrivenData and Professor Emeritus at Olin College.
He is the author of several textbooks -- including Think Python, Think Bayes, and Elements of Data Science -- and "Probably Overthinking It", a blog about data science and Bayesian statistics. He received a Ph.D. in computer science from U.C. Berkeley and Bachelor's and Master's degrees from MIT.
===
PyData is an educational program of NumFOCUS, a 501(c)3 non-profit organization in the United States. PyData provides a forum for the international community of users and developers of data analysis tools to share ideas and learn from each other. The global PyData network promotes discussion of best practices, new approaches, and emerging technologies for data management, processing, analytics, and visualization. PyData communities approach data science using many languages, including (but not limited to) Python, Julia, and R.
PyData conferences aim to be accessible and community-driven, with novice to advanced level presentations. PyData tutorials and talks bring attendees the latest project features along with cutting-edge use cases.
00:00 Welcome!
00:10 Help us add time stamps or captions to this video! See the description for details.
Outline * Problem statement: A/B testing, medical tests, and the Bayesian bandit problem * Prerequisites and goals * Bayes's theorem and the five urn problem * Using Pandas to represent a PMF * Notebook 1: Estimating proportions * From belief to strategy: Thompson sampling * Notebook 2: Implementing and testing Thompson sampling * Debrief: why Bayesian decision analysis is better * More generally: two other examples of BDA * Resources and next steps
Prerequisites
For this tutorial, you should be familiar with Python at an intermediate level. We'll use NumPy, SciPy, and Pandas, but I'll explain what you need to know as we go. You should be familiar with basic probability, but you don't need to know anything about Bayesian statistics.
I'll provide Jupyter notebooks that run on Colab, so you don't have to install anything or prepare ahead of time. But you should be familiar with Jupyter notebooks.
Bio:
Allen Downey
Allen Downey is a Staff Scientist at DrivenData and Professor Emeritus at Olin College.
He is the author of several textbooks -- including Think Python, Think Bayes, and Elements of Data Science -- and "Probably Overthinking It", a blog about data science and Bayesian statistics. He received a Ph.D. in computer science from U.C. Berkeley and Bachelor's and Master's degrees from MIT.
===
PyData is an educational program of NumFOCUS, a 501(c)3 non-profit organization in the United States. PyData provides a forum for the international community of users and developers of data analysis tools to share ideas and learn from each other. The global PyData network promotes discussion of best practices, new approaches, and emerging technologies for data management, processing, analytics, and visualization. PyData communities approach data science using many languages, including (but not limited to) Python, Julia, and R.
PyData conferences aim to be accessible and community-driven, with novice to advanced level presentations. PyData tutorials and talks bring attendees the latest project features along with cutting-edge use cases.
00:00 Welcome!
00:10 Help us add time stamps or captions to this video! See the description for details.
Комментарии