filmov
tv
How to Build an AI Math Compiler from Scratch?
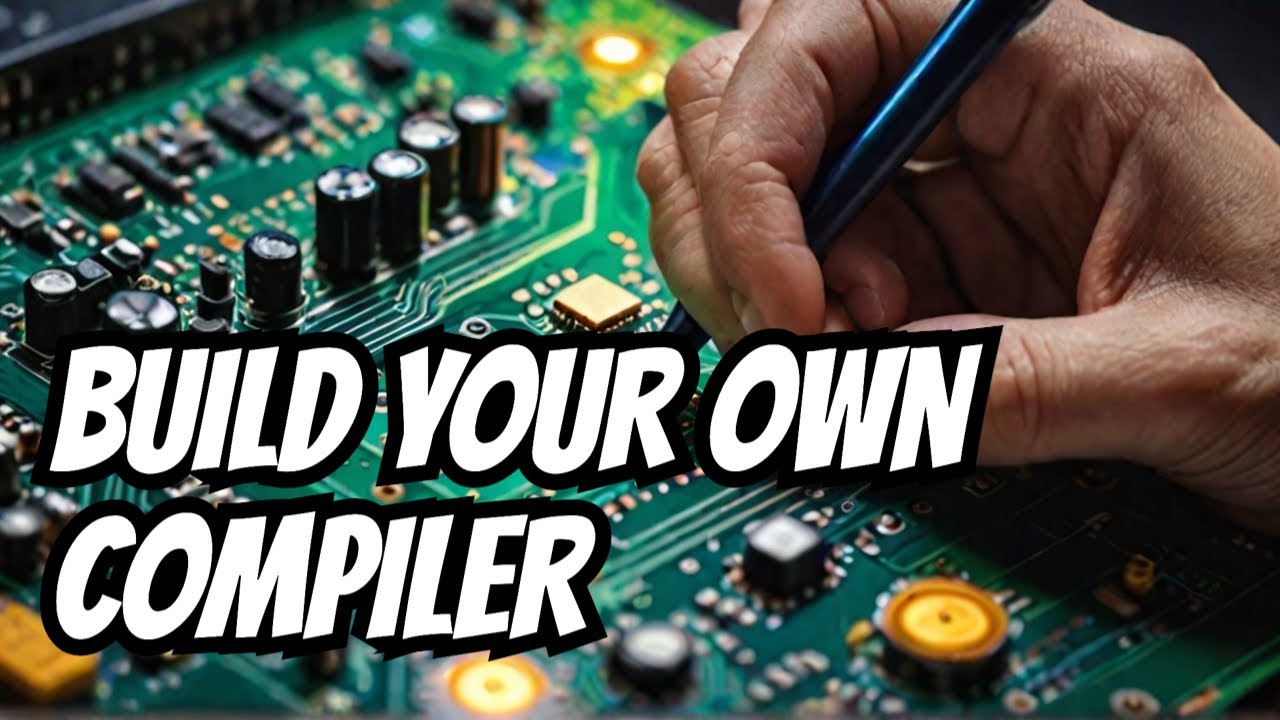
Показать описание
One of the big challenges in AI is synthetically generating math data including the reasoning steps to train large language models such as GPT, Llama3.1, Mistral.. Only with reasoning can you truly train the model. In this video Chris shows how to generate a synthetic dataset using generative ai for math using his new AI math compiler, which accurately returns questions, answers and step by step explanations.. This seems to be a similar technique to Google Deepmind used with Alphaproof and maybe how OpenAI synthetic data generation with Q* or Project Strawberry. If you wish to understand synthetic data generation for ai models or even how compilers work, this is the video for you.
To get accurate results for the step by step explanations, chris couldn't rely on Large Language Models LLM's generating the wrong data, so he had to build a math compiler that could reliably produce the result and the explanations. This is a true compiler with tokenizer, parser, abstract syntax tree and instruction emitter. Instead of emitting assembly instructions however, it natural language prompts. This is a pretty unique technique in the open source world. The compiler also produces explanations. The final trick is to use an LLM such a mistral-nemo to prettify the output into a human readable form.
The code is available here:
To get accurate results for the step by step explanations, chris couldn't rely on Large Language Models LLM's generating the wrong data, so he had to build a math compiler that could reliably produce the result and the explanations. This is a true compiler with tokenizer, parser, abstract syntax tree and instruction emitter. Instead of emitting assembly instructions however, it natural language prompts. This is a pretty unique technique in the open source world. The compiler also produces explanations. The final trick is to use an LLM such a mistral-nemo to prettify the output into a human readable form.
The code is available here:
Комментарии