filmov
tv
EUSIPCO 2020 Keynote - Rebecca Willet 'Model Adaptation for Inverse Problems in Imaging'
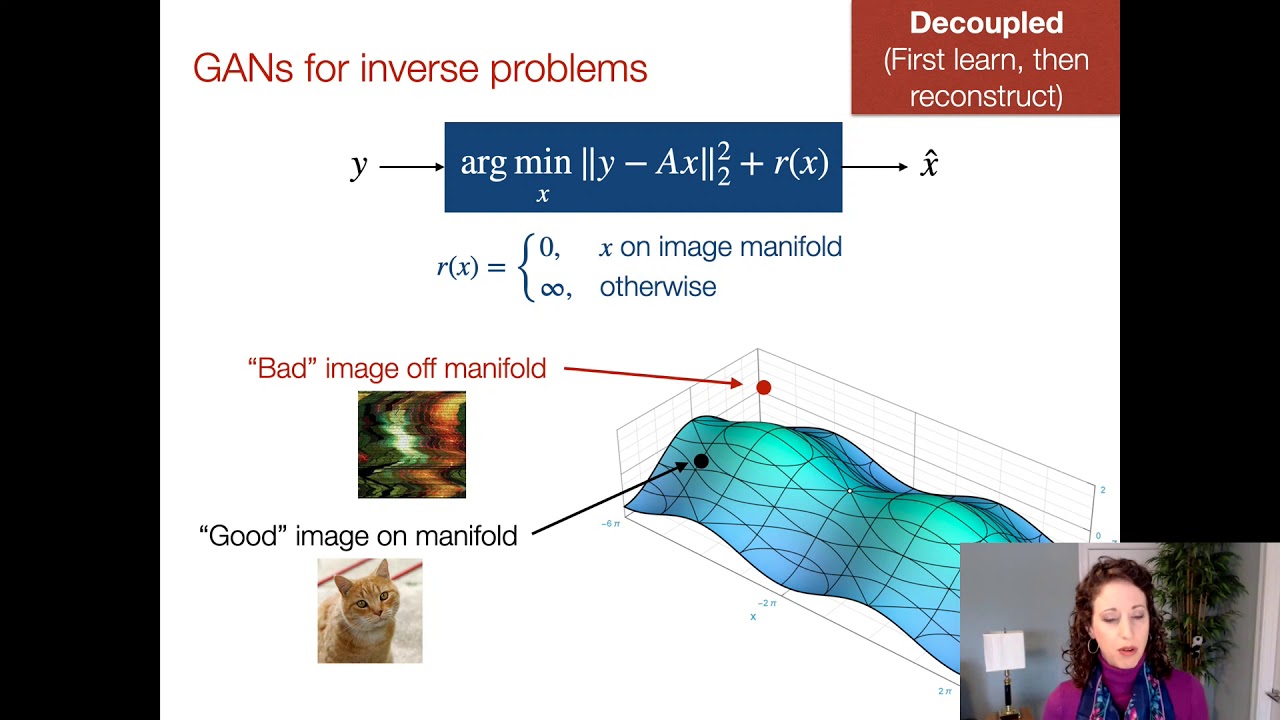
Показать описание
Many challenging image processing tasks can be described by an ill-posed linear inverse problem: deblurring, deconvolution, inpainting, compressed sensing, and superresolution all lie in this framework. Traditional inverse problem solvers minimize a cost function consisting of a data-fit term, which measures how well an image matches the observations, and a regularizer, which reflects prior knowledge and promotes images with desirable properties like smoothness. Recent advances in machine learning and image processing have illustrated that it is often possible to learn a regularizer from training data that can outperform more traditional regularizers. In this talk, I describe the central prevailing themes of this emerging area and present a taxonomy that can be used to categorize different problems and reconstruction methods. We will also explore the lack of robustness of such methods to misspecification of the forward model: if at test time the forward model varies (even slightly) from the one the network was trained on, the network performance can degrade substantially. I will describe novel retraining procedures that adapt the network to reconstruct measurements from a perturbed forward model, even without full knowledge of the perturbation.