filmov
tv
Simulation-based inference in Cosmology using Bayesian Neural Networks: 9 Sep 2022
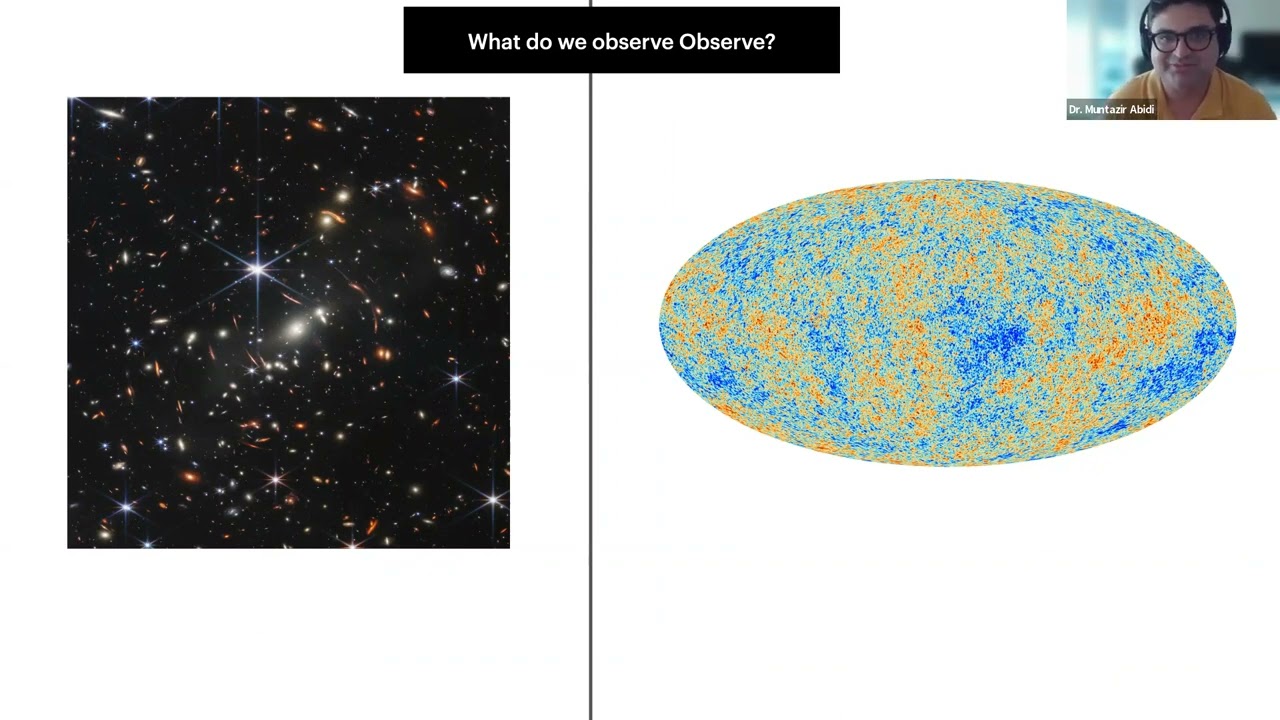
Показать описание
Bio: Dr Muntazir Abidi is a Research Scientist in the Department of Theoretical Physics at the University of Geneva. He completed his BS in Physics from LUMS in 2012. In 2013 he completed a postgraduate diploma in high energy physics from the Abdus Salam International Centre for Theoretical Physics, Trieste, Italy. He completed his Masters in Theoretical Physics and a PhD in Cosmology from the Stephan Hawking Centre for Theoretical Cosmology, University of Cosmology, in 2015 and 2020, respectively. His research interests are large-scale structure cosmology, simulation-based inference and deep learning applications in cosmology/astrophysics. He is a member of the collaboration Simulation-based Inference of Galaxies (SimBiG) which is funded by the Simon’s Foundation and Centre for Computational Astrophysics, Flatiron Institute.
Abstract: Cosmology has entered a new era with large-scale structure (LSS) surveys. The observations of the cosmic microwave background (CMB) radiations have improved our understanding of the Universe. However, there are still many unresolved questions that we want to understand. Current surveys are focused on galaxy clustering and have the potential to improve understanding of the Universe further. However, extracting information about the Universe from these complex datasets is a real challenge due to our inability to model observables accurately. The simulation-based inference is quickly becoming a standard machine learning technique for analyzing massive data in cosmological surveys. SBI relies on generating simulation data which looks like the real observed data. However, due to our inability to include all effects, the SBI can give biased results. In this talk, I will discuss how using Bayesian neural networks can mitigate biases and lead to more reliable inferences outside the training set.
Abstract: Cosmology has entered a new era with large-scale structure (LSS) surveys. The observations of the cosmic microwave background (CMB) radiations have improved our understanding of the Universe. However, there are still many unresolved questions that we want to understand. Current surveys are focused on galaxy clustering and have the potential to improve understanding of the Universe further. However, extracting information about the Universe from these complex datasets is a real challenge due to our inability to model observables accurately. The simulation-based inference is quickly becoming a standard machine learning technique for analyzing massive data in cosmological surveys. SBI relies on generating simulation data which looks like the real observed data. However, due to our inability to include all effects, the SBI can give biased results. In this talk, I will discuss how using Bayesian neural networks can mitigate biases and lead to more reliable inferences outside the training set.