filmov
tv
NEW Llama 3.2 90B - TEST for Image Reasoning (careful!)
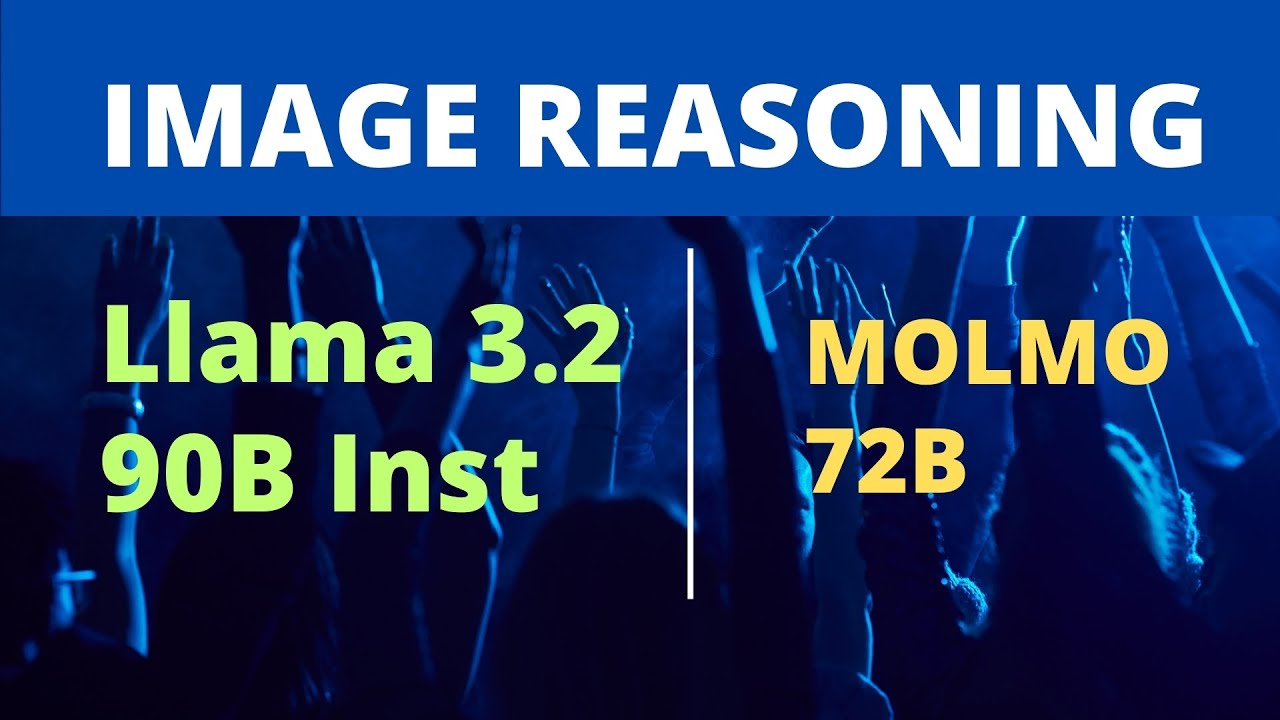
Показать описание
I test the brand new released Llama 3.2 90B VISION model from META and compare it with the new MOLMO Multimodal new model from AllenAI.
Test #1 is simple, both model fail my Test #2.
All rights with authors:
Llama 3.2: Revolutionizing edge AI and vision with open, customizable models
MOLMO
Multimodal Open Language Model built by Ai2
00:00 NEW Llama 3.2 and AllenAI MOLMO Model
01:21 TEST 1 for Image reasoning - MOLMO
02:03 TEST 1 Llama 3.2 90B Instruct VISION
03:02 TEST 2 Llama 3.2 90B Instruct VISION
04:44 TEST 2 on MOLMO Multimodal Model
08:46 TEST 2 Llama 3.2 90B continued and FAIL
#ai
#meta
#artificialintelligence
Test #1 is simple, both model fail my Test #2.
All rights with authors:
Llama 3.2: Revolutionizing edge AI and vision with open, customizable models
MOLMO
Multimodal Open Language Model built by Ai2
00:00 NEW Llama 3.2 and AllenAI MOLMO Model
01:21 TEST 1 for Image reasoning - MOLMO
02:03 TEST 1 Llama 3.2 90B Instruct VISION
03:02 TEST 2 Llama 3.2 90B Instruct VISION
04:44 TEST 2 on MOLMO Multimodal Model
08:46 TEST 2 Llama 3.2 90B continued and FAIL
#ai
#meta
#artificialintelligence
NEW Llama 3.2 90B - TEST for Image Reasoning (careful!)
Meta's New Llama 3.2 with Vision is here - Run it Privately on your Computer
Llama 3.2 is HERE and has VISION 👀
Meta releases Llama 3.2 | NEW small & vision models are here!
Llama 3.2 is Beating OpenAI at Their Own Game (Real-Time AI Voice, Vision...)
Llama 3.2: Best Multimodal Model Yet?
LLaMA 3.2 is HERE - 1B, 3B, 11B & 90B Multimodal - Complete Guide To Run Locally & Finetune
Getting Started With Meta Llama 3.2 And its Variants With Groq And Huggingface
Metas Llama 3.2 Is Much Bigger Than You Think!
Compared! Open Source AI Vision Models Tested, Llama 3.2 vs Pixtral
Meta LLaMA 3.2 is the GAME CHANGER Released From 1B To 90B Multimodal LLM
Meta’s LLaMA 3.2: A Multimodal AI Game-Changer
Napkins + Llama-3.2 : Generate Apps from Screenshots for FREE with Llama-3.2 90B!
Introducing Llama 3.2: BEST Opensource Multimodal LLM Ever!
Testing Llama 3.2 by @meta w/ Langchain & Streamlit (Testing the 3B, 11B, and 90B models)
How to Setup and Test Llama 3.2 VISION Model
NEW: Llama 3.2 Open Source Multimodal🤖🦙 1B, 3B, 11B, & 90 Vision LLM & Orion Glasses Mark Zu...
Try Llama3.2 (11B, 90B) for FREE. Create App with screenshots. #nocode #generativeai #llama3 #vision
How to Use Llama 3.2 to Create Vision Apps and Multimodal Agents in AutoGen
Llama 3.2 Destroys GPT-4 in Vision AI!
How Did Llama-3 Beat Models x200 Its Size?
MultiModal Llama 3.2 has ARRIVED!!!
EASIEST Way to Fine-Tune LLAMA-3.2 and Run it in Ollama
Meta's Llama 3.2 Just STUNNED the Industry! OpenAI is in DANGER (Vision, Real-Time Voice and Mo...
Комментарии