filmov
tv
Every Distance in Data Science (Almost 100K Subs!)
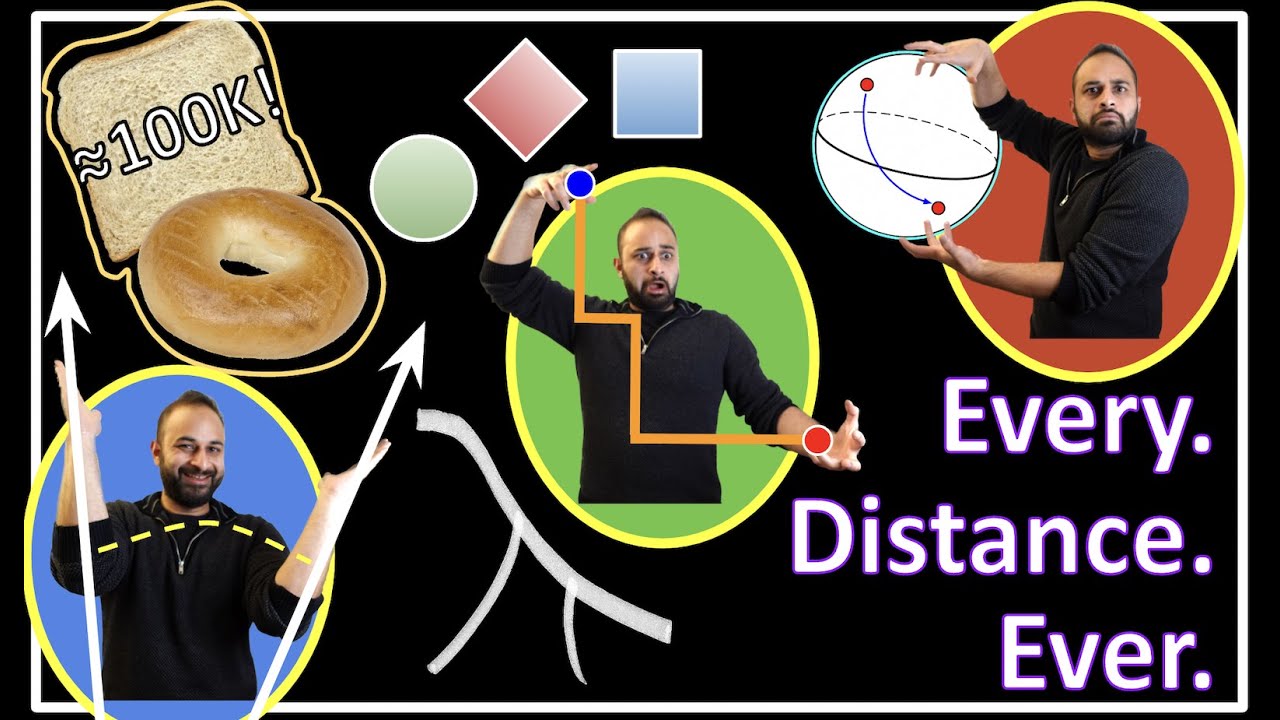
Показать описание
0:00 Intro
2:19 Euclidean Distance
5:47 Manhattan Distance
9:14 Minkowski Distance
12:49 Chebyshev Distance
15:40 Cosine Distance
19:35 Hamming Distance
20:17 Haversine Distance
2:19 Euclidean Distance
5:47 Manhattan Distance
9:14 Minkowski Distance
12:49 Chebyshev Distance
15:40 Cosine Distance
19:35 Hamming Distance
20:17 Haversine Distance
Every Distance in Data Science (Almost 100K Subs!)
Euclidean Distance and Manhattan Distance
Cosine similarity, cosine distance explained | Math, Statistics for data science, machine learning
Distance Metrics for Data Science (Euclidean, Manhattan, Mahalanobis, Levenshtein, and Cosine)
Euclidean Manhattan and Cosine Distance | Euclidean distance vs Cosine similarity
Euclidean Distance simply explained with examples | Science in Data
Euclidean Distance & Cosine Similarity | Introduction to Data Mining part 18
Distance Geometry and Data Science (Lecture 1)
Using IRSA & Gaia to make a star cluster CMD (Computational thinking and Data science)
Distance Geometry and Data Science (Lecture 2)
Distance Geometry and Data Science
Cosine Similarity and Cosine Distance
Understanding Distance & Linkage Methods | Cluster Analysis | Unsupervised Learning | Data Scien...
Gower Distance for Mixed Data
ML 20 : Distance Metrics Models | Euclidean | Manhattan | Minkowski | Hamming Distance with Examples
Leo Liberti -- Distance Geometry in Data Science (tutorial)
Euclidean Distance: Math, Applications and Code #SoME3
IIT Bombay Lecture Hall | IIT Bombay Motivation | #shorts #ytshorts #iit
Jensen-Shannon Distance Explained | Data Science Fundamentals
BEST DEFENCE ACADEMY IN DEHRADUN | NDA FOUNDATION COURSE AFTER 10TH | NDA COACHING #shorts #nda #ssb
Cosine Distance vs Euclidean Distance in Machine Learning and NLP with Word2Vec or Glove Vectors
Normal English vs IELTS English #studyabroad #shorts
How to find Euclidean Manhattan Minkowski distance Supremum distance Cosine Similarity Mahesh Huddar
Why did you choose data science? #shorts
Комментарии