filmov
tv
Data Lake - Data Warehouse | Data Lake vs Data Warehouse | Big Data Course | Part (2/6)
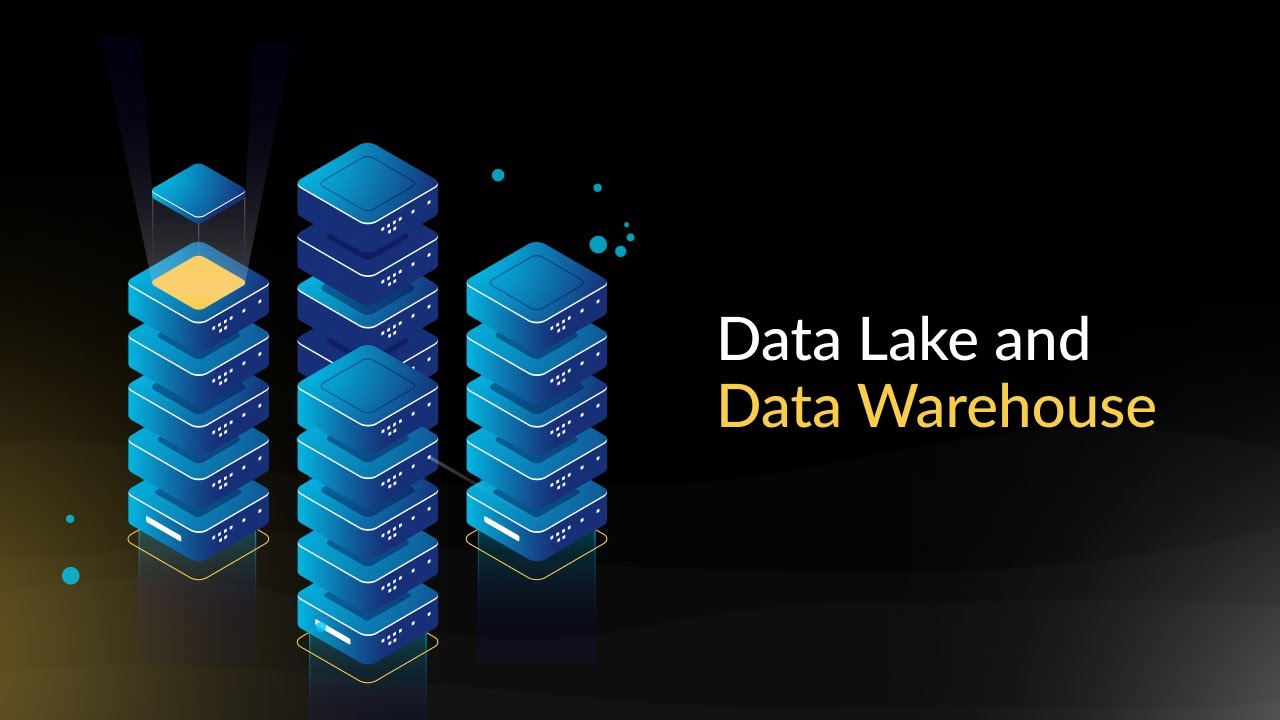
Показать описание
*Data Warehouse* and *Data Lake* are two popular concepts when it comes to data storage and analysis. They are often used together to create a comprehensive solution for Big Data and Analytics. But how do they differ? In this video, we explore the differences between Data Warehouse and Data Lake and how they're used in combination in *Data Analytics Pipeline*
🔖 *Topics Covered*
1- Intro (0:00)
2- What are Data Types (0:37)
3- Statistics about Data Types (2:00)
4- Introduction to Data Lake (3:43)
5- Benefits of Data Lake (4:46)
6- Introduction to Data Warehouse (6:29)
7- What is Data Mart (9:27)
8- Traditional Data Warehousing: Pros & Cons (10:15)
9- Analysing a Data Warehouse (11:39)
10- Data Lake extends Data Warehouse (12:46)
11- Data Lake vs Data Warehouse (12:46)
12- Lesson Summary (16:20)
*Data Lake:* is a centralized repository that allows you to store structured, semi-structured, and unstructured data at any scale. Data lakes promise the ability to store all data for a business in a single repository. You can leverage data lakes to store large volumes of data instead of persisting that data in data warehouses. Data lakes are generally less expensive than specialized big data storage solutions. That way, you only pay for the specialized solutions when using them for processing and analytics and not for long-term storage.
*Data Warehouse:* is a central repository of structured data from many data sources. This data is transformed, aggregated, and prepared for business reporting and analysis. Data flows into a data warehouse from transactional systems, relational databases, and other sources. These data sources can include structured, semi-structured, and unstructured data. These data sources are transformed into structured data before they are stored in the data warehouse.
A subset of data from a data warehouse is called a *Data Mart.* Data marts only focus on one subject or functional area. A warehouse might contain all relevant sources for an enterprise, but a data mart might store only a single department's sources. Because data marts are generally a copy of data already contained in a data warehouse, they are often fast and simple to implement.
When it comes to *choosing between Data Warehouse and Data Lake,* it depends on the type of data that needs to be stored and analyzed. If the data is structured and in multiple formats, then a Data Warehouse solution is the best option. However, if the data is unstructured or semi-structured, then a Data Lake is the best solution.
In conclusion, Data Warehousing and Data Lakes can be used in combination to create a comprehensive solution for Big Data and Analytics. AWS provides a comprehensive suite of cloud-based data warehouse and data lake solutions, and Big Data Course provides users with the knowledge and tools needed to build a data lake or data warehouse in the cloud. Understanding the differences between Data Warehouse and Data Lake will help organizations make the best decision when it comes to storing and analyzing their data.
--------------------------------------------------
Get In Touch
🔖 *Topics Covered*
1- Intro (0:00)
2- What are Data Types (0:37)
3- Statistics about Data Types (2:00)
4- Introduction to Data Lake (3:43)
5- Benefits of Data Lake (4:46)
6- Introduction to Data Warehouse (6:29)
7- What is Data Mart (9:27)
8- Traditional Data Warehousing: Pros & Cons (10:15)
9- Analysing a Data Warehouse (11:39)
10- Data Lake extends Data Warehouse (12:46)
11- Data Lake vs Data Warehouse (12:46)
12- Lesson Summary (16:20)
*Data Lake:* is a centralized repository that allows you to store structured, semi-structured, and unstructured data at any scale. Data lakes promise the ability to store all data for a business in a single repository. You can leverage data lakes to store large volumes of data instead of persisting that data in data warehouses. Data lakes are generally less expensive than specialized big data storage solutions. That way, you only pay for the specialized solutions when using them for processing and analytics and not for long-term storage.
*Data Warehouse:* is a central repository of structured data from many data sources. This data is transformed, aggregated, and prepared for business reporting and analysis. Data flows into a data warehouse from transactional systems, relational databases, and other sources. These data sources can include structured, semi-structured, and unstructured data. These data sources are transformed into structured data before they are stored in the data warehouse.
A subset of data from a data warehouse is called a *Data Mart.* Data marts only focus on one subject or functional area. A warehouse might contain all relevant sources for an enterprise, but a data mart might store only a single department's sources. Because data marts are generally a copy of data already contained in a data warehouse, they are often fast and simple to implement.
When it comes to *choosing between Data Warehouse and Data Lake,* it depends on the type of data that needs to be stored and analyzed. If the data is structured and in multiple formats, then a Data Warehouse solution is the best option. However, if the data is unstructured or semi-structured, then a Data Lake is the best solution.
In conclusion, Data Warehousing and Data Lakes can be used in combination to create a comprehensive solution for Big Data and Analytics. AWS provides a comprehensive suite of cloud-based data warehouse and data lake solutions, and Big Data Course provides users with the knowledge and tools needed to build a data lake or data warehouse in the cloud. Understanding the differences between Data Warehouse and Data Lake will help organizations make the best decision when it comes to storing and analyzing their data.
--------------------------------------------------
Get In Touch