filmov
tv
Unlocking the True Value of Big Data with Open Data Science
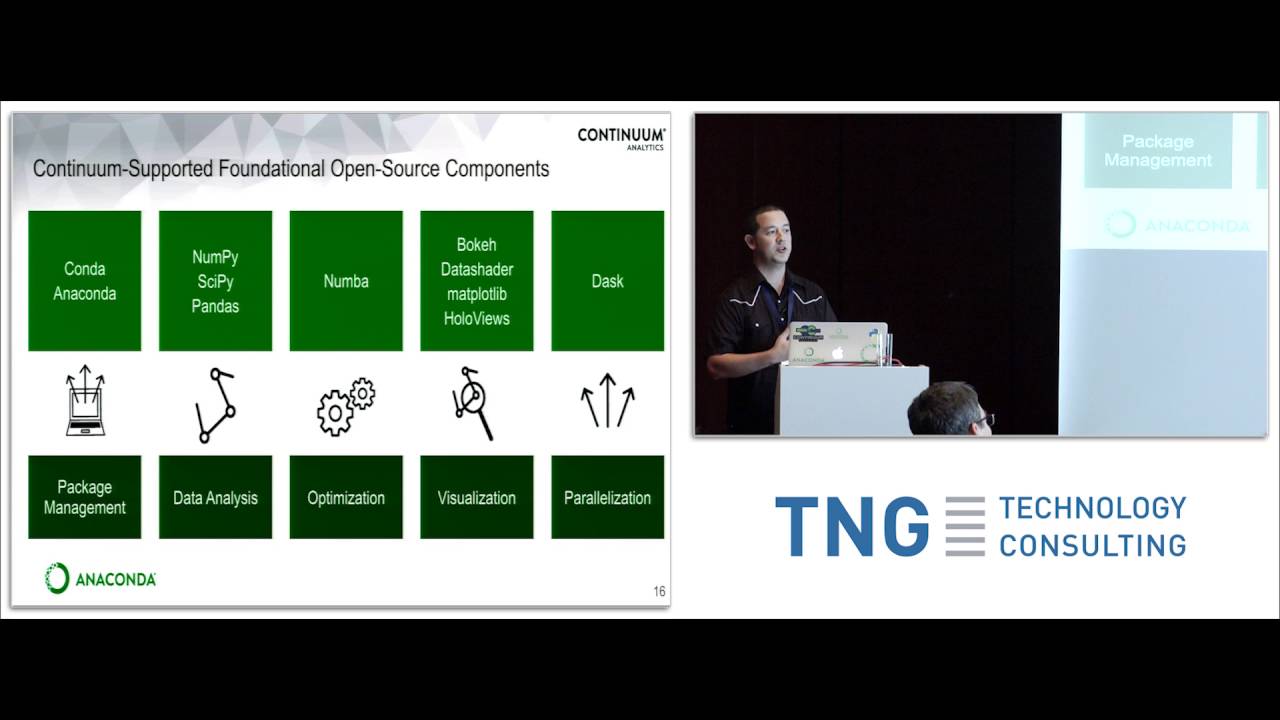
Показать описание
Speaker: Dr. Kristopher Overholt, Solution Architect, Continuum Analytics
The high potential of Big Data Analytics is clear across all verticals and the industry, yet organizations still struggle to unlock its true value. Even with the latest distributed execution frameworks in the Hadoop ecosystem, they are challenged with leveraging Big Data, advanced analytics and computing power in their clusters. Organizations need to enable data scientists and analysts to leverage solutions that include data analytics, visualization, parallelization and optimization. Also, they need to scale up and scale out to perform distributed computations in cluster environments using tools in Open Data Science, including the Python and R ecosystems. Enterprises demand flexibility, high performance and efficient use of memory to scale up their Big Data workloads, especially for numerical and statistical computations. They need cost effective business results from their Big Data investments and the ability to leverage the latest innovations to outperform their last generation technology. In order to deliver on this promise, Big Data Analytics has to be simplified so that the skills that exist in the enterprise can easily maximize the benefits of Big Data. This requires scalable package and dependency management of existing analytics tools, as well as flexible parallel frameworks to scale up their Big Data workflows, including machine learning, image processing and natural language processing. In this session, enterprises will learn how to leverage the power of Open Data Science to extract value and get high performance and interactive analytics from Big Data. The speaker will demonstrate examples of high-performance, distributed Python computations that include:
In-memory natural language processing and interactive queries on text data in HDFS
Analysis of NYC taxi data through distributed dataframes on a cluster on HDFS
Creation of interactive distributed visualizations of global temperature data
Parallelization of existing legacy code with custom file formats
Data Scientists will hear about how to achieve lightning fast processing of computationally intensive distributed analytics with Python to realize the full value of their Big Data.
Kristopher Overholt is a solution architect at Continuum Analytics who works with scientific software development and distributed/cluster computing, including Python, Hadoop and Spark for data analysis and data engineering workflows. Kristopher received a Ph.D. in Civil Engineering from The University of Texas at Austin in 2013 and holds a B.S. and M.S. in Fire Protection Engineering. Prior to joining Continuum, he worked at the National Institute of Standards and Technology (NIST), Southwest Research Institute and The University of Texas at Austin. Kristopher has more than a decade of experience in areas including applied research, scientific computing, small-scale and large-scale experiments, system administration, open-source software development, computational modeling and hands-on technician/electronics work.
The high potential of Big Data Analytics is clear across all verticals and the industry, yet organizations still struggle to unlock its true value. Even with the latest distributed execution frameworks in the Hadoop ecosystem, they are challenged with leveraging Big Data, advanced analytics and computing power in their clusters. Organizations need to enable data scientists and analysts to leverage solutions that include data analytics, visualization, parallelization and optimization. Also, they need to scale up and scale out to perform distributed computations in cluster environments using tools in Open Data Science, including the Python and R ecosystems. Enterprises demand flexibility, high performance and efficient use of memory to scale up their Big Data workloads, especially for numerical and statistical computations. They need cost effective business results from their Big Data investments and the ability to leverage the latest innovations to outperform their last generation technology. In order to deliver on this promise, Big Data Analytics has to be simplified so that the skills that exist in the enterprise can easily maximize the benefits of Big Data. This requires scalable package and dependency management of existing analytics tools, as well as flexible parallel frameworks to scale up their Big Data workflows, including machine learning, image processing and natural language processing. In this session, enterprises will learn how to leverage the power of Open Data Science to extract value and get high performance and interactive analytics from Big Data. The speaker will demonstrate examples of high-performance, distributed Python computations that include:
In-memory natural language processing and interactive queries on text data in HDFS
Analysis of NYC taxi data through distributed dataframes on a cluster on HDFS
Creation of interactive distributed visualizations of global temperature data
Parallelization of existing legacy code with custom file formats
Data Scientists will hear about how to achieve lightning fast processing of computationally intensive distributed analytics with Python to realize the full value of their Big Data.
Kristopher Overholt is a solution architect at Continuum Analytics who works with scientific software development and distributed/cluster computing, including Python, Hadoop and Spark for data analysis and data engineering workflows. Kristopher received a Ph.D. in Civil Engineering from The University of Texas at Austin in 2013 and holds a B.S. and M.S. in Fire Protection Engineering. Prior to joining Continuum, he worked at the National Institute of Standards and Technology (NIST), Southwest Research Institute and The University of Texas at Austin. Kristopher has more than a decade of experience in areas including applied research, scientific computing, small-scale and large-scale experiments, system administration, open-source software development, computational modeling and hands-on technician/electronics work.