filmov
tv
The Ethics of AI Navigating the Challenges of Machine Learning
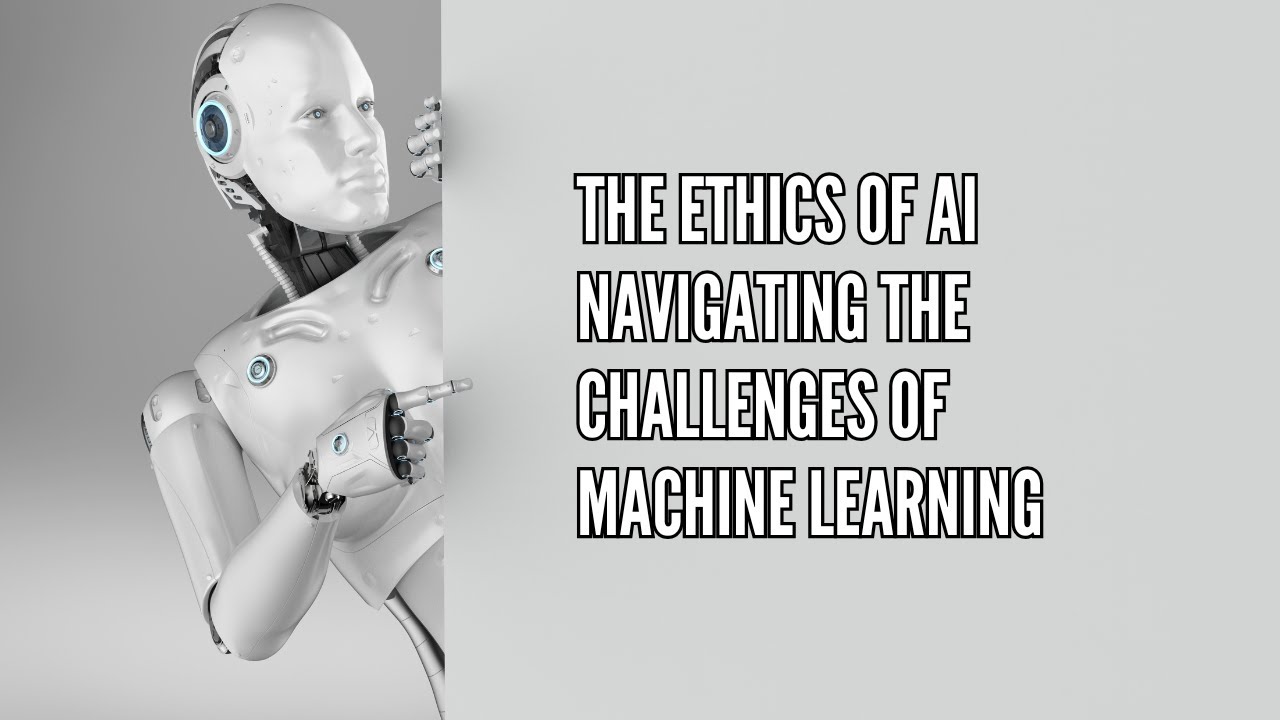
Показать описание
# The Ethics of AI: Navigating the Challenges of Machine Learning
Artificial intelligence (AI) and machine learning (ML) have become essential components of modern technology, revolutionizing industries ranging from healthcare to finance and education. However, the rapid development and deployment of these technologies raise significant ethical challenges that demand careful attention. As AI systems increasingly influence decision-making and shape the way society functions, it is critical to examine the ethical implications they bring. Navigating these challenges requires a balance between innovation and responsibility to ensure AI serves humanity fairly, transparently, and sustainably.
One of the most pressing ethical concerns in AI is the issue of bias. Machine learning models are trained on datasets that reflect real-world behaviors and patterns. However, these datasets often contain historical biases, leading to algorithms that reinforce discrimination or inequality. For example, AI systems used in hiring processes have been shown to favor candidates from specific demographic groups while excluding others. Similarly, facial recognition technologies have demonstrated lower accuracy when identifying individuals with darker skin tones. Addressing algorithmic bias requires diversifying datasets, auditing systems regularly, and ensuring that AI solutions are designed with inclusivity in mind.
Another significant challenge revolves around transparency and explainability. Many AI models, particularly those based on deep learning, operate as “black boxes,” making it difficult to understand how they reach their conclusions. This lack of transparency can lead to ethical dilemmas, especially in high-stakes scenarios like healthcare or criminal justice, where people’s lives are directly impacted. If users cannot trust or comprehend the decision-making process, it undermines confidence in AI systems. Developing explainable AI models that offer clarity without compromising performance is essential for fostering trust and accountability.
Privacy is also a crucial concern in the ethical use of AI. Machine learning relies on vast amounts of data, including personal information, to train and improve algorithms. While this data is valuable, it raises questions about consent, ownership, and potential misuse. AI-driven surveillance systems, for example, can monitor individuals without their knowledge, eroding personal privacy and creating a culture of constant observation. Striking a balance between leveraging data for innovation and respecting individual privacy is a fundamental challenge for AI developers and policymakers alike.
The deployment of AI in workplaces and industries introduces the ethical issue of labor displacement. Automation powered by machine learning has streamlined processes and increased efficiency, but it has also rendered certain jobs obsolete. As AI systems take over repetitive tasks, workers face unemployment or the need for reskilling. Ensuring that the benefits of AI are distributed fairly requires governments and companies to invest in training programs that help displaced workers transition into new roles. A socially responsible approach to AI adoption involves planning for both technological progress and the people affected by it.
AI also raises ethical concerns about accountability. When an AI system makes a mistake—such as a self-driving car causing an accident or a predictive policing algorithm making false predictions—determining who is responsible becomes complex. Is it the developer, the user, or the company behind the technology? Current legal frameworks are not fully equipped to handle such situations, and new regulations are needed to assign responsibility and provide clear guidelines for accountability. The ethical use of AI demands that all stakeholders take responsibility for their roles in deploying and managing these systems.
**#AIEthics #MachineLearningChallenges #ResponsibleAI #BiasInAI #TransparentAI #ExplainableAI #AIPrivacy #DigitalEthics #AlgorithmicBias #EthicalTech #SustainableAI #PrivacyMatters #AIAccountability #FairAI #InclusiveTechnology #AIRegulation #FutureOfAI #TechForGood #AITrust #MLEthics #DataEthics #LaborDisplacement #AIAndJobs #SocialImpactOfAI #EthicalAI #GreenAI #AITransparency #OpenSourceAI #AIForAll #UnintendedConsequences #PredictiveAlgorithms #AIInSociety #AIAndGovernance #DigitalInclusion #TechResponsibility #AIAndPrivacy #AIInnovation #DataOwnership #SustainableTechnology #AIAndAccountability #DigitalEquity #GlobalAI #AIAndMisinformation #AlgorithmicResponsibility #AIAndWorkforce #TechnologyForHumanity #AIAndEnvironment #InclusiveInnovation #MLBias #AITrustworthiness**
Artificial intelligence (AI) and machine learning (ML) have become essential components of modern technology, revolutionizing industries ranging from healthcare to finance and education. However, the rapid development and deployment of these technologies raise significant ethical challenges that demand careful attention. As AI systems increasingly influence decision-making and shape the way society functions, it is critical to examine the ethical implications they bring. Navigating these challenges requires a balance between innovation and responsibility to ensure AI serves humanity fairly, transparently, and sustainably.
One of the most pressing ethical concerns in AI is the issue of bias. Machine learning models are trained on datasets that reflect real-world behaviors and patterns. However, these datasets often contain historical biases, leading to algorithms that reinforce discrimination or inequality. For example, AI systems used in hiring processes have been shown to favor candidates from specific demographic groups while excluding others. Similarly, facial recognition technologies have demonstrated lower accuracy when identifying individuals with darker skin tones. Addressing algorithmic bias requires diversifying datasets, auditing systems regularly, and ensuring that AI solutions are designed with inclusivity in mind.
Another significant challenge revolves around transparency and explainability. Many AI models, particularly those based on deep learning, operate as “black boxes,” making it difficult to understand how they reach their conclusions. This lack of transparency can lead to ethical dilemmas, especially in high-stakes scenarios like healthcare or criminal justice, where people’s lives are directly impacted. If users cannot trust or comprehend the decision-making process, it undermines confidence in AI systems. Developing explainable AI models that offer clarity without compromising performance is essential for fostering trust and accountability.
Privacy is also a crucial concern in the ethical use of AI. Machine learning relies on vast amounts of data, including personal information, to train and improve algorithms. While this data is valuable, it raises questions about consent, ownership, and potential misuse. AI-driven surveillance systems, for example, can monitor individuals without their knowledge, eroding personal privacy and creating a culture of constant observation. Striking a balance between leveraging data for innovation and respecting individual privacy is a fundamental challenge for AI developers and policymakers alike.
The deployment of AI in workplaces and industries introduces the ethical issue of labor displacement. Automation powered by machine learning has streamlined processes and increased efficiency, but it has also rendered certain jobs obsolete. As AI systems take over repetitive tasks, workers face unemployment or the need for reskilling. Ensuring that the benefits of AI are distributed fairly requires governments and companies to invest in training programs that help displaced workers transition into new roles. A socially responsible approach to AI adoption involves planning for both technological progress and the people affected by it.
AI also raises ethical concerns about accountability. When an AI system makes a mistake—such as a self-driving car causing an accident or a predictive policing algorithm making false predictions—determining who is responsible becomes complex. Is it the developer, the user, or the company behind the technology? Current legal frameworks are not fully equipped to handle such situations, and new regulations are needed to assign responsibility and provide clear guidelines for accountability. The ethical use of AI demands that all stakeholders take responsibility for their roles in deploying and managing these systems.
**#AIEthics #MachineLearningChallenges #ResponsibleAI #BiasInAI #TransparentAI #ExplainableAI #AIPrivacy #DigitalEthics #AlgorithmicBias #EthicalTech #SustainableAI #PrivacyMatters #AIAccountability #FairAI #InclusiveTechnology #AIRegulation #FutureOfAI #TechForGood #AITrust #MLEthics #DataEthics #LaborDisplacement #AIAndJobs #SocialImpactOfAI #EthicalAI #GreenAI #AITransparency #OpenSourceAI #AIForAll #UnintendedConsequences #PredictiveAlgorithms #AIInSociety #AIAndGovernance #DigitalInclusion #TechResponsibility #AIAndPrivacy #AIInnovation #DataOwnership #SustainableTechnology #AIAndAccountability #DigitalEquity #GlobalAI #AIAndMisinformation #AlgorithmicResponsibility #AIAndWorkforce #TechnologyForHumanity #AIAndEnvironment #InclusiveInnovation #MLBias #AITrustworthiness**