filmov
tv
Terence Tao's Central Limit Theorem Proof, Double Factorials (!!) and the Moment Method #SoME3
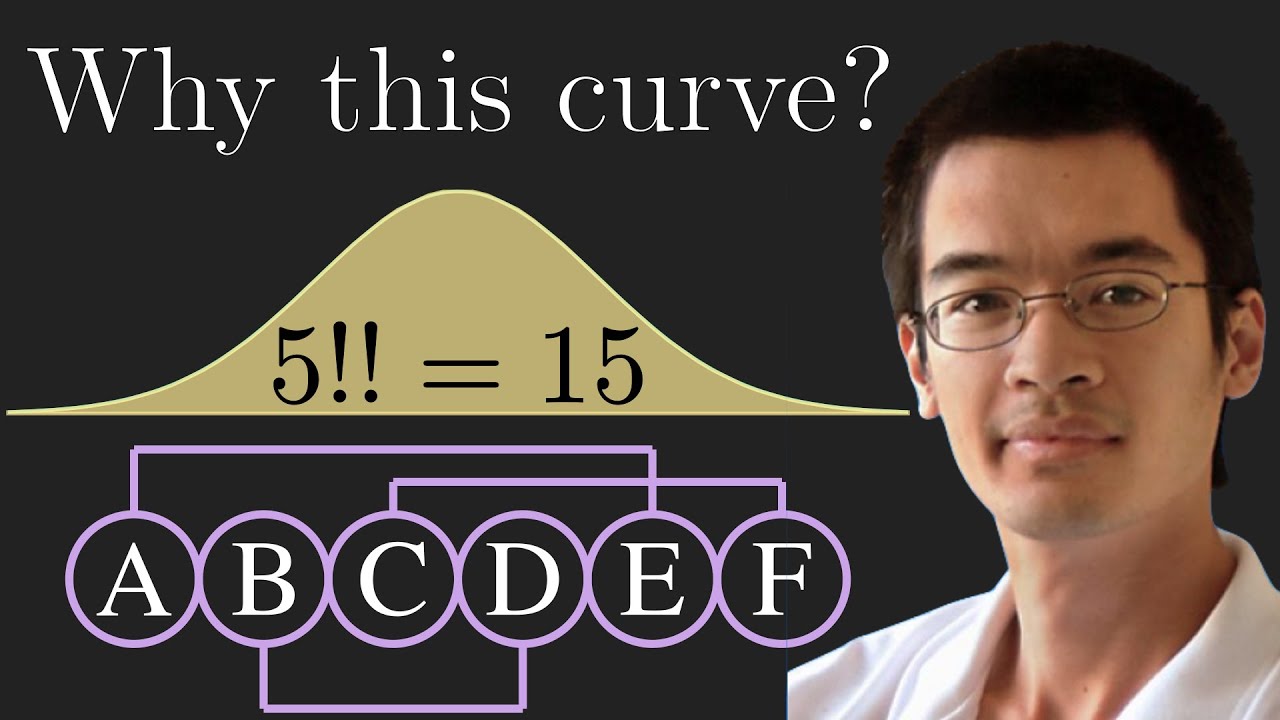
Показать описание
==================================================
FOLLOW UP QUESTIONS
==================================================
Possible questions to answer in a follow up videos. Let me know in the comments if you would want to see any of these!
Q: Why is this in a random matrix book? A: There is a similar proof for something called the semi-circle law for random matrices. If you understand this proof, you can understand that one quite easily!
Q: Why is a sum of Gaussian’s still a Gaussian? A: There is a cute way to see this using only moments! It is a cute counting argument with pair partitions which are labeled with some extra labels.
Q: How is this related to the characteristic function/Fourier transform? A: The moments can be added together in a generating series to get things like the characteristic function .
============================================
LINKS
============================================
Link to Terence Tao’s book (from his terrytao blog). The moment method proof is section 2.2.3 starting on page 106.
Short pdf that includes the main proof ideas
3Blue1Brown Central Limit Theorem playlist:
But what is the Central Limit Theorem?
Why π is in the normal distribution (beyond integral tricks)
Convolutions | Why X+Y in probability is a beautiful mess
Why is the "central limit" a normal distribution?
==================================
Info on how the video was made
==================================
===============
Timestamps
===============
0:00 Terence Tao
0:38 What is the CLT
2:40 But why?
3:30 Double factorials
4:30 Gaussian moments
5:52 Moments of sums
6:29 Pair partitions
9:00 Two main results
10:08 Part 1-Gaussian moments
13:08 Solving the recurrence relation
15:35 Part 2-Moments of sums
17:17 k equals 1
18:00 k equals 2
21:47 k equals 3
25:42 k equals 4
29:41 Recap and overview
#3blue1brown #some #some3 #probability #Gaussian #math #integration #integrationbyparts #combinatorics
Комментарии