filmov
tv
Certifying Almost All Quantum States with Few Single-Qubit Measurements | Qiskit Quantum Seminar
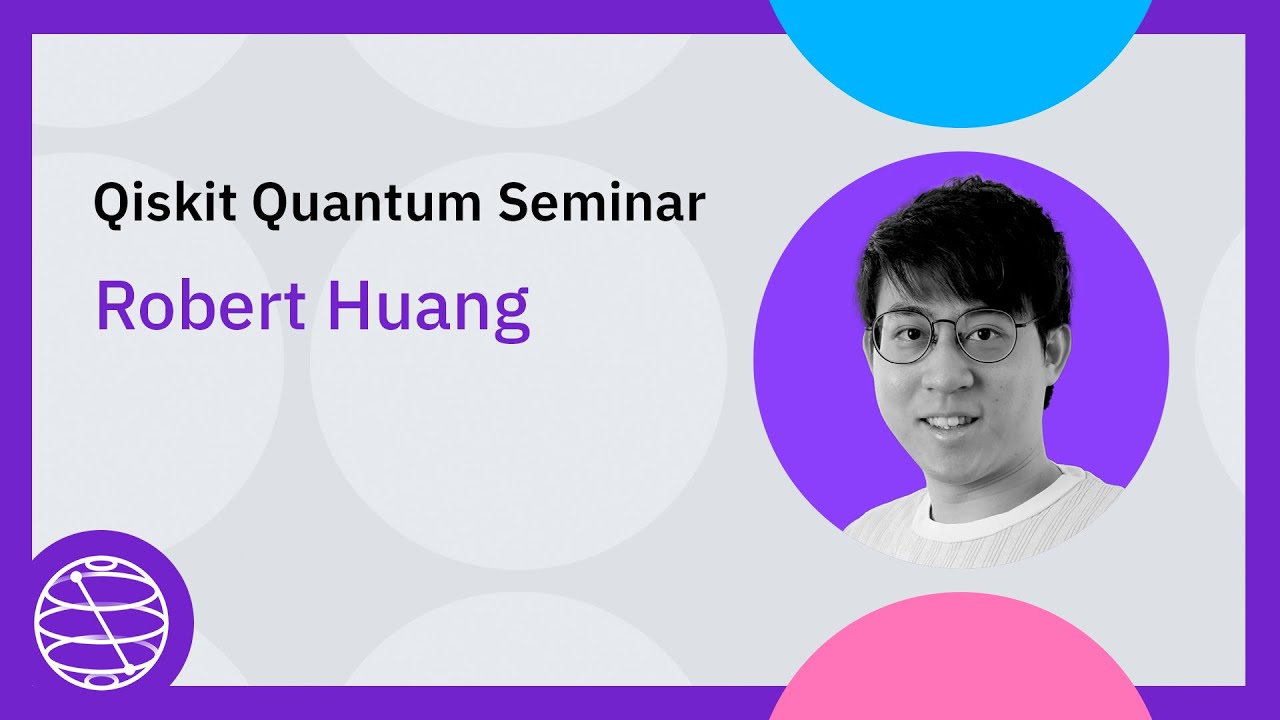
Показать описание
Episode 173
Certifying that an n-qubit state synthesized in the lab is close to the target state is a fundamental task in quantum information science. However, existing rigorous protocols either require deep quantum circuits or exponentially many single-qubit measurements. In this work, we prove that almost all n-qubit target states, including those with exponential circuit complexity, can be certified from only O(n^2) single-qubit measurements. This result is established by a new technique that relates certification to the mixing time of a random walk. Our protocol has applications for benchmarking quantum systems, for optimizing quantum circuits to generate a desired target state, and for learning and verifying neural networks, tensor networks, and various other representations of quantum states using only single-qubit measurements. We show that such verified representations can be used to efficiently predict highly non-local properties that would otherwise require an exponential number of measurements. We demonstrate these applications in numerical experiments with up to 120 qubits, and observe advantage over existing methods such as cross-entropy benchmarking (XEB).
Hsin-Yuan Huang (Robert) is a Senior Research Scientist at Google Quantum AI and a Visiting Scientist at MIT. In 2025, he will join California Institute of Technology as an Assistant Professor of Theoretical Physics. He completed his Ph.D. under the mentorship of John Preskill and Thomas Vidick.
His research harnesses learning theory to unveil novel insights in physics, information science, and quantum computation. His seminal contributions include classical shadow tomography for learning large-scale quantum systems, provably efficient machine learning algorithms for solving quantum many-body problems, and quantum advantages in learning from experiments. His findings have been published in Nature, Science, Nature Physics, Nature Review Physics, Nature Communications, Physical Review Letters, PRX Quantum, as well as premier conferences in theoretical computer science, such as IEEE Symposium on Foundations of Computer Science (FOCS), and ACM Symposium on Theory of Computing (STOC). Over the past five years, he has been invited to give more than 120 research talks.
His doctoral dissertation, "Learning in the Quantum Universe," was honored with the Milton and Francis Clauser Doctoral Prize. This award, bestowed annually to a single doctoral dissertation across all disciplines at Caltech, recognizes work demonstrating the highest degree of originality and potential for opening new avenues of human thought and endeavor. He was also awarded the Ben P. C. Chou Doctoral Prize in Information Science and Technology, a Google Ph.D. fellowship, the Boeing Quantum Creator Prize, the MediaTek Research Young Scholarship, and the Kortschak Scholarship. Starting in 2025, Dr. Hsin-Yuan Huang will hold the position of William H. Hurt Scholar, an endowed early career professorship at California Institute of Technology.
Certifying that an n-qubit state synthesized in the lab is close to the target state is a fundamental task in quantum information science. However, existing rigorous protocols either require deep quantum circuits or exponentially many single-qubit measurements. In this work, we prove that almost all n-qubit target states, including those with exponential circuit complexity, can be certified from only O(n^2) single-qubit measurements. This result is established by a new technique that relates certification to the mixing time of a random walk. Our protocol has applications for benchmarking quantum systems, for optimizing quantum circuits to generate a desired target state, and for learning and verifying neural networks, tensor networks, and various other representations of quantum states using only single-qubit measurements. We show that such verified representations can be used to efficiently predict highly non-local properties that would otherwise require an exponential number of measurements. We demonstrate these applications in numerical experiments with up to 120 qubits, and observe advantage over existing methods such as cross-entropy benchmarking (XEB).
Hsin-Yuan Huang (Robert) is a Senior Research Scientist at Google Quantum AI and a Visiting Scientist at MIT. In 2025, he will join California Institute of Technology as an Assistant Professor of Theoretical Physics. He completed his Ph.D. under the mentorship of John Preskill and Thomas Vidick.
His research harnesses learning theory to unveil novel insights in physics, information science, and quantum computation. His seminal contributions include classical shadow tomography for learning large-scale quantum systems, provably efficient machine learning algorithms for solving quantum many-body problems, and quantum advantages in learning from experiments. His findings have been published in Nature, Science, Nature Physics, Nature Review Physics, Nature Communications, Physical Review Letters, PRX Quantum, as well as premier conferences in theoretical computer science, such as IEEE Symposium on Foundations of Computer Science (FOCS), and ACM Symposium on Theory of Computing (STOC). Over the past five years, he has been invited to give more than 120 research talks.
His doctoral dissertation, "Learning in the Quantum Universe," was honored with the Milton and Francis Clauser Doctoral Prize. This award, bestowed annually to a single doctoral dissertation across all disciplines at Caltech, recognizes work demonstrating the highest degree of originality and potential for opening new avenues of human thought and endeavor. He was also awarded the Ben P. C. Chou Doctoral Prize in Information Science and Technology, a Google Ph.D. fellowship, the Boeing Quantum Creator Prize, the MediaTek Research Young Scholarship, and the Kortschak Scholarship. Starting in 2025, Dr. Hsin-Yuan Huang will hold the position of William H. Hurt Scholar, an endowed early career professorship at California Institute of Technology.