filmov
tv
Rohit Vuppala-OSU-A Machine Learning Approach to predict Wind Field Data
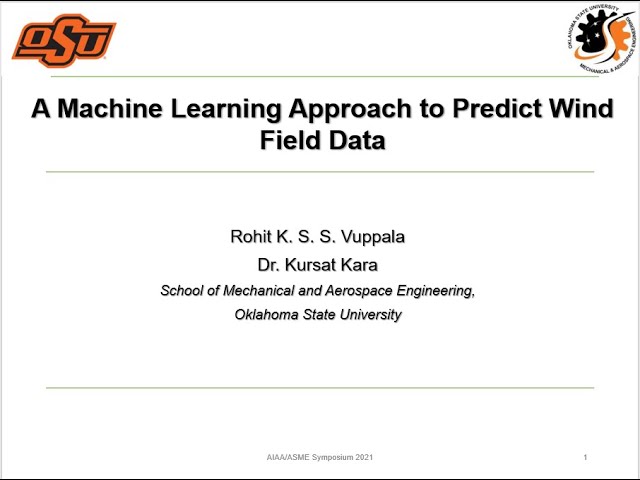
Показать описание
This video is part of the AIAA/ASME 2021 Symposium at Oklahoma State University.
Abstract:
Spatio-temporal wind field data prediction is becoming increasingly essential for many systems' safe operation, like wind energy generation and gust avoidance for small Unmanned Aerial Systems (UAS). These systems are susceptible to changes in local wind patterns. Wind field prediction and forecasting in real-time are necessary for robust and continuous operation. While state-of-the-art local and global Numerical Weather Prediction (NWP) tools are available at our disposal, the computational cost associated with them makes their use immensely prohibitive for 'real-time' or close to 'real-time' predictions. However, with the recent advancements in Machine Learning algorithms and techniques, it is possible to harness Neural Networks and associated frameworks for wind field prediction. Using Large Eddy Simulation (LES) data from numerical solvers for atmospheric flows as an offline training data set, we could train the Neural Networks model. Subsequently, it can be deployed for short-term prediction of the local flow fields from the trained network. Building upon other robust mathematical techniques like Principal Component Analysis (PCA) or Proper Orthogonal Decomposition (POD), we could further decrease the computational cost associated with training and deployment of Long Short-Term Memory (LSTM) Neural Networks for the problem reducing the overall cost. This work aims to provide a machine learning approach for wind field prediction using numerical solutions for atmospheric flows over a domain of interest.
Abstract:
Spatio-temporal wind field data prediction is becoming increasingly essential for many systems' safe operation, like wind energy generation and gust avoidance for small Unmanned Aerial Systems (UAS). These systems are susceptible to changes in local wind patterns. Wind field prediction and forecasting in real-time are necessary for robust and continuous operation. While state-of-the-art local and global Numerical Weather Prediction (NWP) tools are available at our disposal, the computational cost associated with them makes their use immensely prohibitive for 'real-time' or close to 'real-time' predictions. However, with the recent advancements in Machine Learning algorithms and techniques, it is possible to harness Neural Networks and associated frameworks for wind field prediction. Using Large Eddy Simulation (LES) data from numerical solvers for atmospheric flows as an offline training data set, we could train the Neural Networks model. Subsequently, it can be deployed for short-term prediction of the local flow fields from the trained network. Building upon other robust mathematical techniques like Principal Component Analysis (PCA) or Proper Orthogonal Decomposition (POD), we could further decrease the computational cost associated with training and deployment of Long Short-Term Memory (LSTM) Neural Networks for the problem reducing the overall cost. This work aims to provide a machine learning approach for wind field prediction using numerical solutions for atmospheric flows over a domain of interest.