filmov
tv
Divide-and-Conquer Monte Carlo Tree Search For Goal-Directed Planning (Paper Explained)
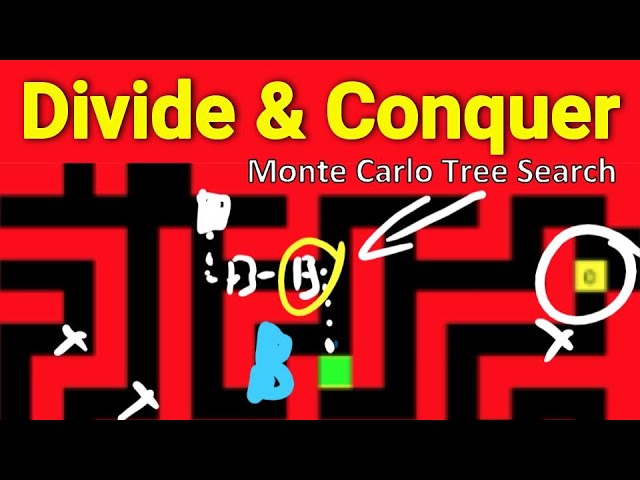
Показать описание
When AI makes a plan it usually does so step by step, forward in time. But often it is beneficial to define intermediate goals to divide a large problem into easier sub-problems. This paper proposes a generalization of MCTS that searches not for the best next actions to take, but for the best way to sub-divide the problem recursively into problems so tiny that they can each be solved in a single step.
Abstract:
Standard planners for sequential decision making (including Monte Carlo planning, tree search, dynamic programming, etc.) are constrained by an implicit sequential planning assumption: The order in which a plan is constructed is the same in which it is executed. We consider alternatives to this assumption for the class of goal-directed Reinforcement Learning (RL) problems. Instead of an environment transition model, we assume an imperfect, goal-directed policy. This low-level policy can be improved by a plan, consisting of an appropriate sequence of sub-goals that guide it from the start to the goal state. We propose a planning algorithm, Divide-and-Conquer Monte Carlo Tree Search (DC-MCTS), for approximating the optimal plan by means of proposing intermediate sub-goals which hierarchically partition the initial tasks into simpler ones that are then solved independently and recursively. The algorithm critically makes use of a learned sub-goal proposal for finding appropriate partitions trees of new tasks based on prior experience. Different strategies for learning sub-goal proposals give rise to different planning strategies that strictly generalize sequential planning. We show that this algorithmic flexibility over planning order leads to improved results in navigation tasks in grid-worlds as well as in challenging continuous control environments.
Authors: Giambattista Parascandolo, Lars Buesing, Josh Merel, Leonard Hasenclever, John Aslanides, Jessica B. Hamrick, Nicolas Heess, Alexander Neitz, Theophane Weber
Links:
Abstract:
Standard planners for sequential decision making (including Monte Carlo planning, tree search, dynamic programming, etc.) are constrained by an implicit sequential planning assumption: The order in which a plan is constructed is the same in which it is executed. We consider alternatives to this assumption for the class of goal-directed Reinforcement Learning (RL) problems. Instead of an environment transition model, we assume an imperfect, goal-directed policy. This low-level policy can be improved by a plan, consisting of an appropriate sequence of sub-goals that guide it from the start to the goal state. We propose a planning algorithm, Divide-and-Conquer Monte Carlo Tree Search (DC-MCTS), for approximating the optimal plan by means of proposing intermediate sub-goals which hierarchically partition the initial tasks into simpler ones that are then solved independently and recursively. The algorithm critically makes use of a learned sub-goal proposal for finding appropriate partitions trees of new tasks based on prior experience. Different strategies for learning sub-goal proposals give rise to different planning strategies that strictly generalize sequential planning. We show that this algorithmic flexibility over planning order leads to improved results in navigation tasks in grid-worlds as well as in challenging continuous control environments.
Authors: Giambattista Parascandolo, Lars Buesing, Josh Merel, Leonard Hasenclever, John Aslanides, Jessica B. Hamrick, Nicolas Heess, Alexander Neitz, Theophane Weber
Links:
Комментарии