filmov
tv
#26 Machine Learning Specialization [Course 1, Week 2, Lesson 2]
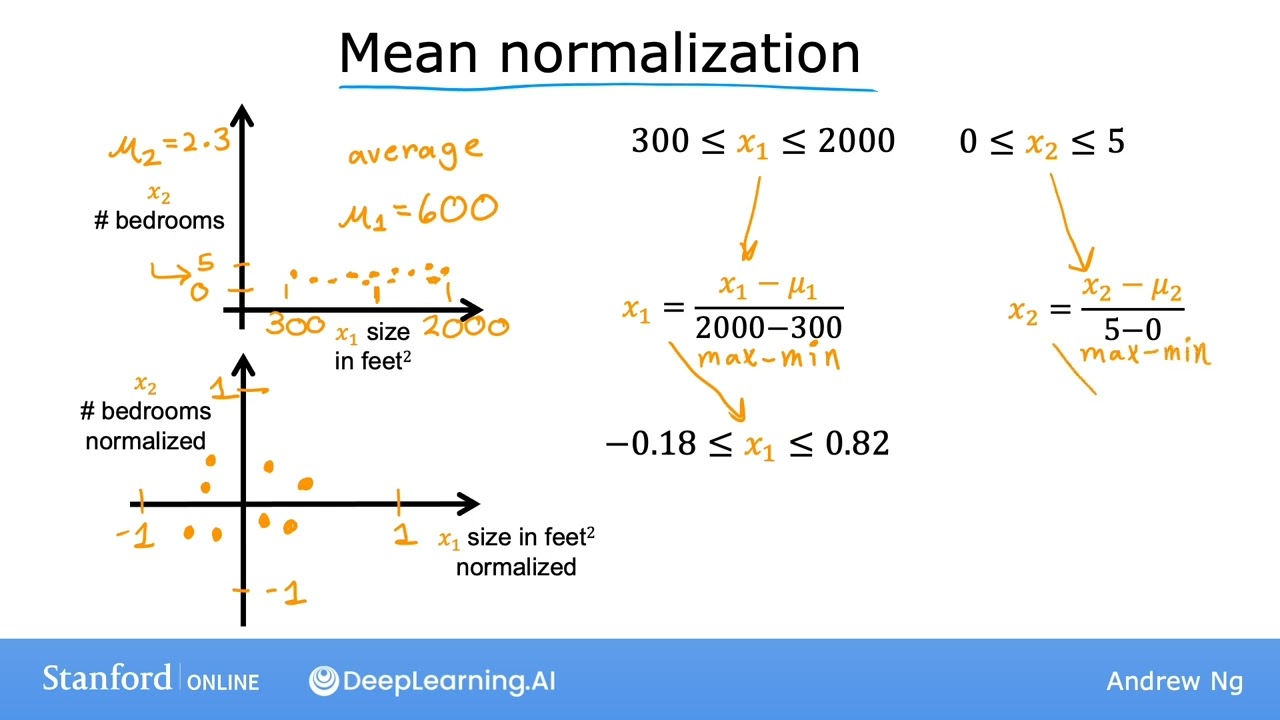
Показать описание
The Machine Learning Specialization is a foundational online program created in collaboration between DeepLearning.AI and Stanford Online. This beginner-friendly program will teach you the fundamentals of machine learning and how to use these techniques to build real-world AI applications.
This Specialization is taught by Andrew Ng, an AI visionary who has led critical research at Stanford University and groundbreaking work at Google Brain, Baidu, and Landing.AI to advance the AI field.
This video is from Course 1 (Supervised Machine Learning Regression and Classification), Week 2 (Regression with multiple input variables), Lesson 2 (Gradient descent in practice), Video 2 (Feature scaling part 2).
Follow us:
This Specialization is taught by Andrew Ng, an AI visionary who has led critical research at Stanford University and groundbreaking work at Google Brain, Baidu, and Landing.AI to advance the AI field.
This video is from Course 1 (Supervised Machine Learning Regression and Classification), Week 2 (Regression with multiple input variables), Lesson 2 (Gradient descent in practice), Video 2 (Feature scaling part 2).
Follow us:
#26 Machine Learning Specialization [Course 1, Week 2, Lesson 2]
Andrew Ng’s Machine Learning Specialization 2022 | What is it and is it worth taking?
I can't STOP reading these Machine Learning Books!
#26 Machine Learning Engineering for Production (MLOps) Specialization [Course 1, Week 3 Lesson 2]
Coursera Self-Driving Cars Specialization Final Project
Stanford Machine Learning Specialization - Review 2024 - Andrew Ng Machine Learning Specialization
Coursera x Stanford Machine Learning specialization
#26 AI for Good Specialization [Course 1, Week 2, Lesson 2]
AI vs ML vs DL vs Data Science - Difference Explained | Simplilearn
PyTorch for Deep Learning & Machine Learning – Full Course
Deep Learning Specialization: A Comprehensive Review - Is It Worth It In 2023?
Step-by-Step guide to become AI Engineer
AI VS ML VS DL VS Data Science
#25 Machine Learning Specialization [Course 1, Week 2, Lesson 2]
Introduction to Machine Learning for Beginners [Part 1] | Machine Learning for Beginners
Best Machine Learning Masters Programs For 2022-2023 (US Edition)
Will AI replace Software Engineers? Future of Tech
#21 Machine Learning Specialization [Course 1, Week 2, Lesson 1]
Machine Learning Course for Beginners
I asked ChatGPT to do EDA for me: Here's how it went
5 Beginner Friendly Steps to Learn Machine Learning
Machine Learning Specialization on Coursera in 2023
#28 Machine Learning Specialization [Course 1, Week 2, Lesson 2]
Do You Need Mathematics To Learn Machine Learning?
Комментарии