filmov
tv
The Epistemology of Deep Learning - Yann LeCun
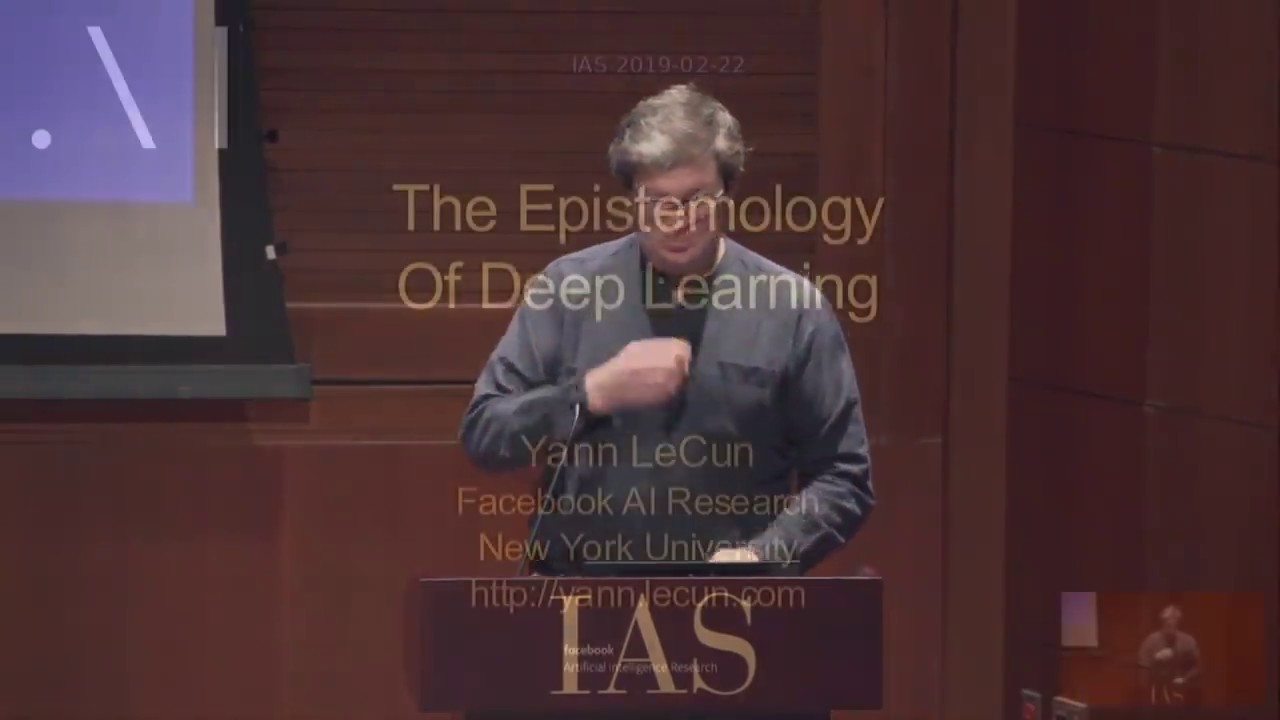
Показать описание
Deep Learning: Alchemy or Science?
Topic: The Epistemology of Deep Learning
Speaker: Yann LeCun
Affiliation: Facebook AI Research/New York University
Date: February 22, 2019
Topic: The Epistemology of Deep Learning
Speaker: Yann LeCun
Affiliation: Facebook AI Research/New York University
Date: February 22, 2019
The Epistemology of Deep Learning - Yann LeCun
Is Epistemic Uncertainty Faithfully Represented by Evidential Deep Learning Methods? - ICML 2024
Is Epistemic Uncertainy faithfully represented by Evidential Deep Learning Methods?
#07 - What Kinds of Knowledge Can AI Provide? The Epistemology of AI and Machine Vision
This is why Deep Learning is really weird.
Large Language Models and Kantian Epistemology
How do we teach Ontology and Epistemology to a machine?
Exploring Heidegger's Epistemology in the Age of Artificial Intelligence
Should attention be all we need? The ethical and epistemic implications of unification in machine...
Epistemology - A Deep-Dive
Epistemology Meets AI: Understanding Knowledge #aiphilosophy #artificialconsciousness #aiandethics
What is Epistemology? (Philosophical Definitions)
Neural Epistemology in Dynamical System Learning
Should attention be all we need? The ethical and epistemic implications of unification in machine...
'Quantitative Epistemology: How Machine Learning can help humans become better decision-makers&...
Uncertainty (Aleatoric vs Epistemic) | Machine Learning
A Deeper Look into Aleatoric and Epistemic Uncertainty Disentanglement - LXCV CVPR 2022 - Pitch
Day 4 - J. Maclure: The Epistemology and Ethics of LLMs
Konstantin Genin: Morals and Methodology
Quine's Naturalized Epistemology Explained: How Science Redefines Knowledge!
Confusion Says: Epistemology Part 1
Unlocking the Secrets of Cultural Knowledge: A Deep Dive into Epistemology w/ James Giordano PhD
An internal critique of Confucianism. #shorts #confuciusquotes #easternphilosophy #epistemology
Epistemology of Chatbots | Steven Gubka
Комментарии