filmov
tv
Nadav Cohen: Generalization in Deep Learning Through the Lens of Implicit Rank Minimization
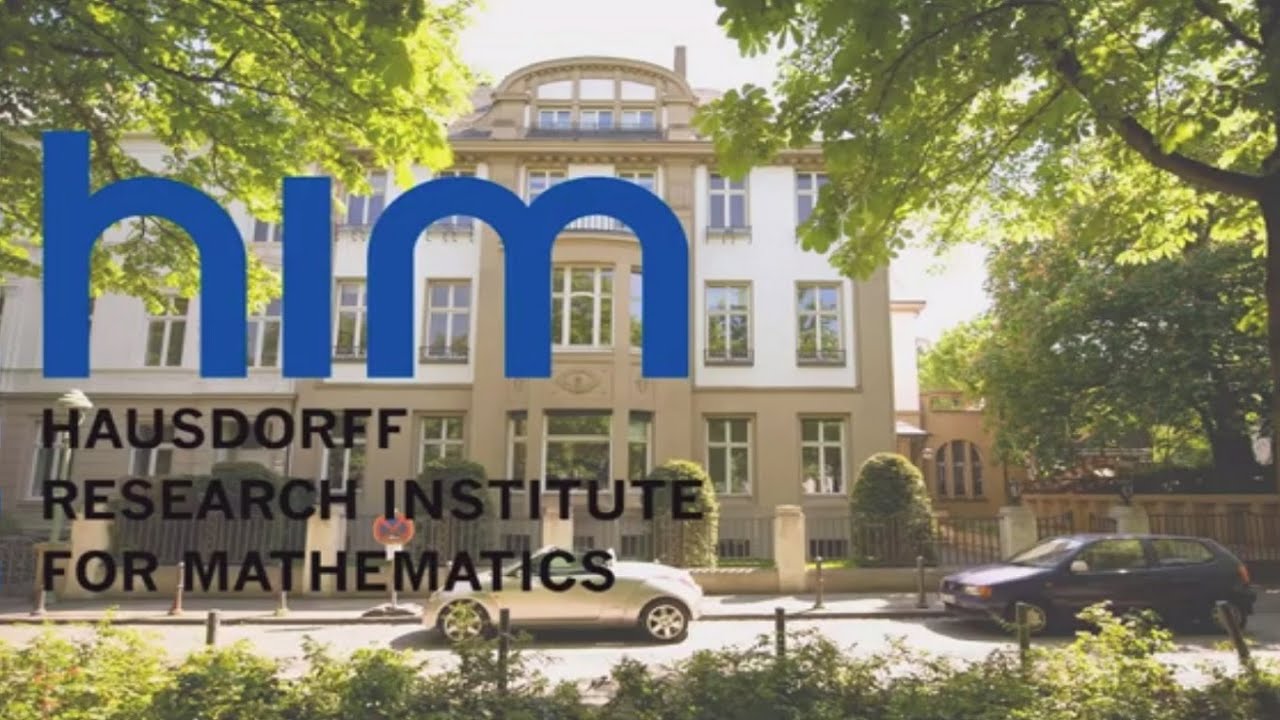
Показать описание
Abstract: The mysterious ability of neural networks to generalize is believed to stem from an implicit regularization - a tendency of gradient-based optimization to t training data with predictors of low "complexity." Despite vast efforts, a satisfying formalization of the latter belief is lacking. In this talk I will present a series of works theoretically analyzing the implicit regularization in matrix and tensor factorizations, known to be equivalent to certain linear and non-linear neural networks, respectively. Through dynamical characterizations, I will establish implicit regularization towards low (matrix and tensor) ranks, different from any type of norm minimization, in contrast to prior beliefs. I will then discuss implications of this finding for both theory and practice of modern deep learning. The results I will present highlight the potential of ranks to explain and improve generalization in deep learning.
Works covered in the talk were in collaboration with Sanjeev Arora, Wei Hu, Yuping Luo, Asaf Maman and Noam Razin.
Works covered in the talk were in collaboration with Sanjeev Arora, Wei Hu, Yuping Luo, Asaf Maman and Noam Razin.