filmov
tv
'Federated Learning, Knowledge Transfer, and Knowledge...' Prof. Witold Pedrycz (ICSOFT 2022)
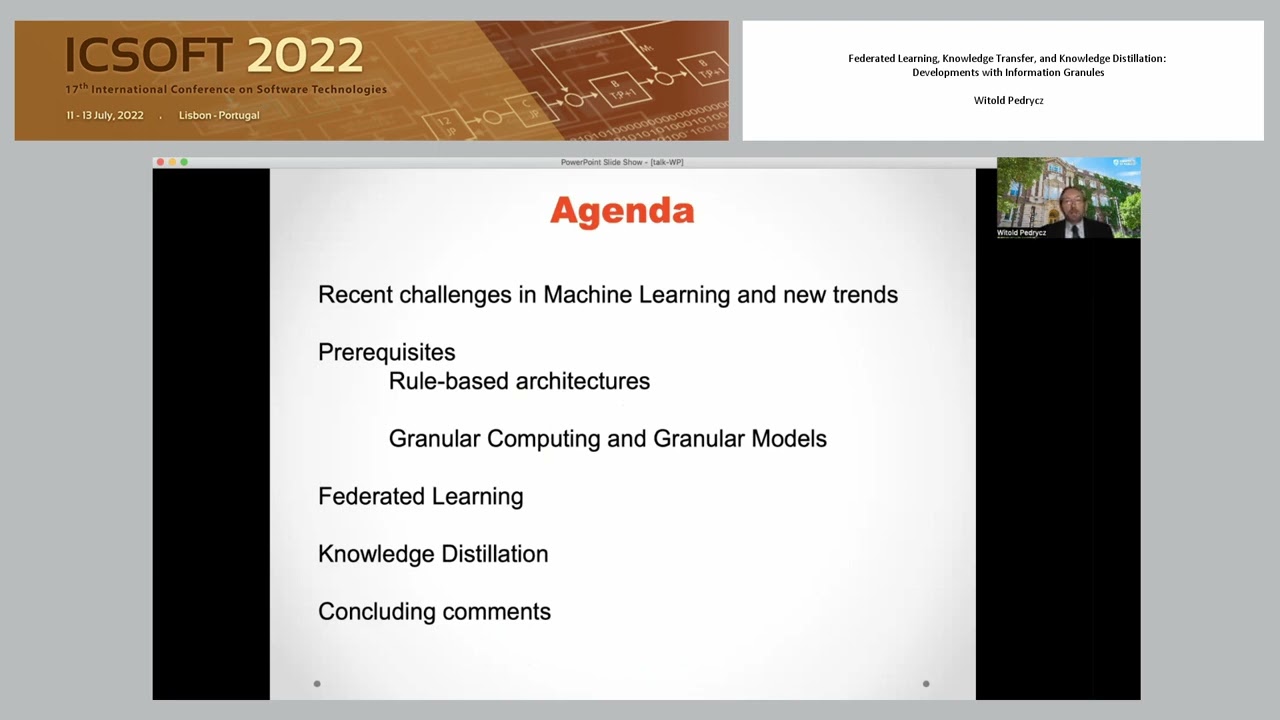
Показать описание
Keynote Title: Federated Learning, Knowledge Transfer, and Knowledge Distillation: Developments with Information Granules
Keynote Lecturer: Witold Pedrycz
Presented on: 11/07/2022, Lisbon, Portugal
Abstract: The visible trends of Machine Learning addressing the emerging needs of coping with the diversity of real-world motivated learning scenarios involve federated learning, transfer learning, and knowledge distillation. We advocate that to conveniently address these challenges encountered in these directions, it becomes beneficial to engage the fundamental framework of Granular Computing to enhance the above approaches or to establish new and augmented methodologies. We demonstrate that various ways of conceptualization of information granules in the form of fuzzy sets, sets, rough sets, among others, lead to efficient solutions. To establish a sound conceptual modeling framework, we include a brief discussion of concepts of information granules and Granular Computing. In the sequel, a concise information granules-oriented design of rule-based architectures is discussed. A way of forming the rules through unsupervised federated learning is investigated along with algorithmic developments. A granular characterization of the model formed by the server vis-a-vis data located at individual clients is presented. It is demonstrated that the quality of the rules at the client’s end is described in terms of granular parameters and subsequently the global model becomes represented as a granular model. Subsequently, the roles of granular augmentations of models in the setting of granular knowledge transfer and knowledge distillation, in particular, are discussed.
Presented at the following Conference: ICSOFT, 17th International Conference on Software Technologies
Keynote Lecturer: Witold Pedrycz
Presented on: 11/07/2022, Lisbon, Portugal
Abstract: The visible trends of Machine Learning addressing the emerging needs of coping with the diversity of real-world motivated learning scenarios involve federated learning, transfer learning, and knowledge distillation. We advocate that to conveniently address these challenges encountered in these directions, it becomes beneficial to engage the fundamental framework of Granular Computing to enhance the above approaches or to establish new and augmented methodologies. We demonstrate that various ways of conceptualization of information granules in the form of fuzzy sets, sets, rough sets, among others, lead to efficient solutions. To establish a sound conceptual modeling framework, we include a brief discussion of concepts of information granules and Granular Computing. In the sequel, a concise information granules-oriented design of rule-based architectures is discussed. A way of forming the rules through unsupervised federated learning is investigated along with algorithmic developments. A granular characterization of the model formed by the server vis-a-vis data located at individual clients is presented. It is demonstrated that the quality of the rules at the client’s end is described in terms of granular parameters and subsequently the global model becomes represented as a granular model. Subsequently, the roles of granular augmentations of models in the setting of granular knowledge transfer and knowledge distillation, in particular, are discussed.
Presented at the following Conference: ICSOFT, 17th International Conference on Software Technologies