filmov
tv
Introduction to Conformal Prediction and Distribution-Free Uncertainty Quantification
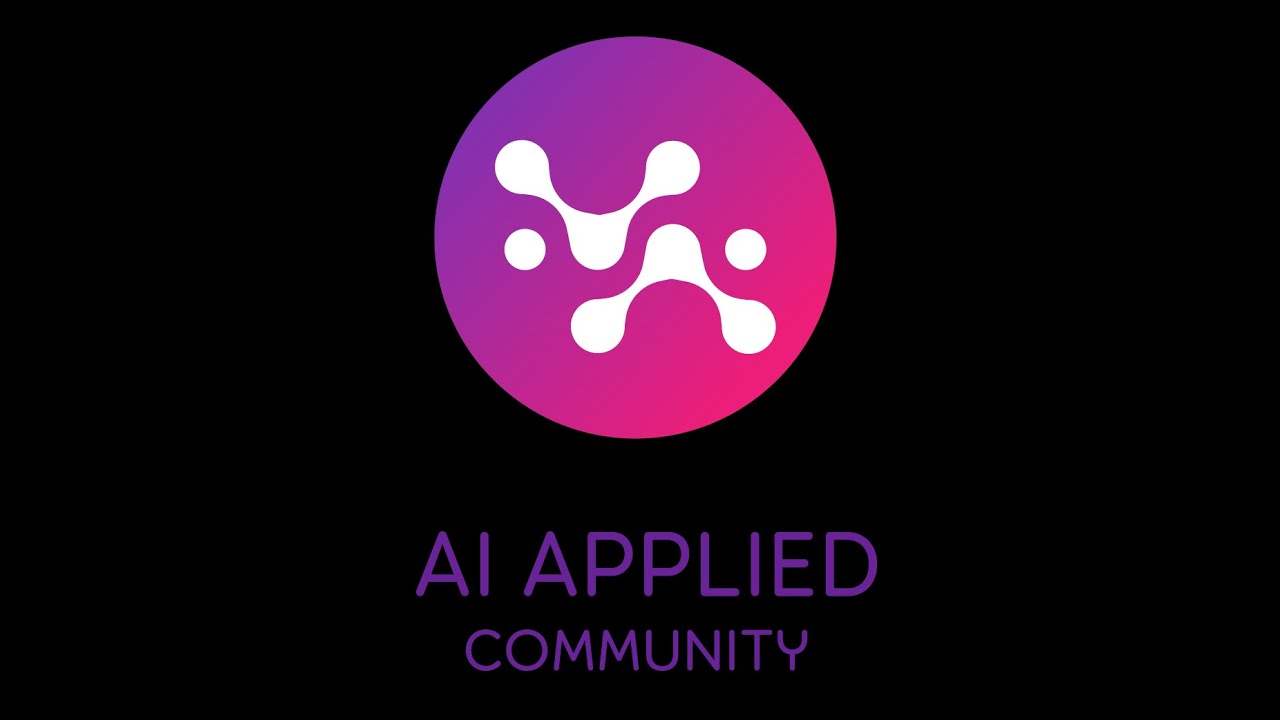
Показать описание
As we begin deploying machine learning models in consequential settings like medical diagnostics or self-driving vehicles, knowing a model's accuracy is not enough. We need a way of quantifying an algorithm's uncertainty for a particular test-time instance while rigorously guaranteeing that consequential errors don't happen too frequently (for example, that the car doesn't hit a human). I'll discuss how to generate rigorous, finite-sample confidence intervals for any prediction task, model and dataset for free.
A Tutorial on Conformal Prediction
Intro to Conformal Prediction
Uncertainty Quantification (1): Enter Conformal Predictors
Introduction to Conformal Prediction and Distribution-Free Uncertainty Quantification
Introduction to Conformal Prediction and Distribution-Free Uncertainty Quantification
An introduction to conformal prediction
Prediction Intervals with Conformal Inference: An Intuitive Explanation
Three Easy Steps to Understand Conformal Prediction (CP), Conformity Score, Python Implementation
Conformal Prediction: Enhanced Method for Understanding the Prediction Quality
Conformal Prediction
A Tutorial on Conformal Prediction Part 2: Conditional Coverage and Diagnostics
Henrik Linusson: Conformal Prediction
Uncertainty Quantification (4A): Implementing Split Conformal - Relation for Prediction Intervals
Uncertainty Quantification (2): Full Conformal Predictors
[CW Paper-Club] Introduction to Conformal Prediction & Distribution-Free Uncertainty Quantificat...
Tutorial on Conformal Prediction Part 3: Beyond Conformal Prediction
Learning Optimal Conformal Classifiers with David Stutz of DeepMind
Michael I. Jordan on Conformal Prediction
Conformal Prediction Tutorial
Is a 99% accurate ML model actually trustable? Conformal Predictions
Quantifying the Uncertainty in Model Predictions
Conformal Predictions in Machine Learning with Python
Ying Jin - Prediction-Assisted Screening and Discovery with Conformal p-values
MoroccoAI webinar - Dr. Soundouss Messoudi - 'Confidence learning using conformal prediction&a...
Комментарии