filmov
tv
Is Tree-based RAG Struggling? Not with Knowledge Graphs!
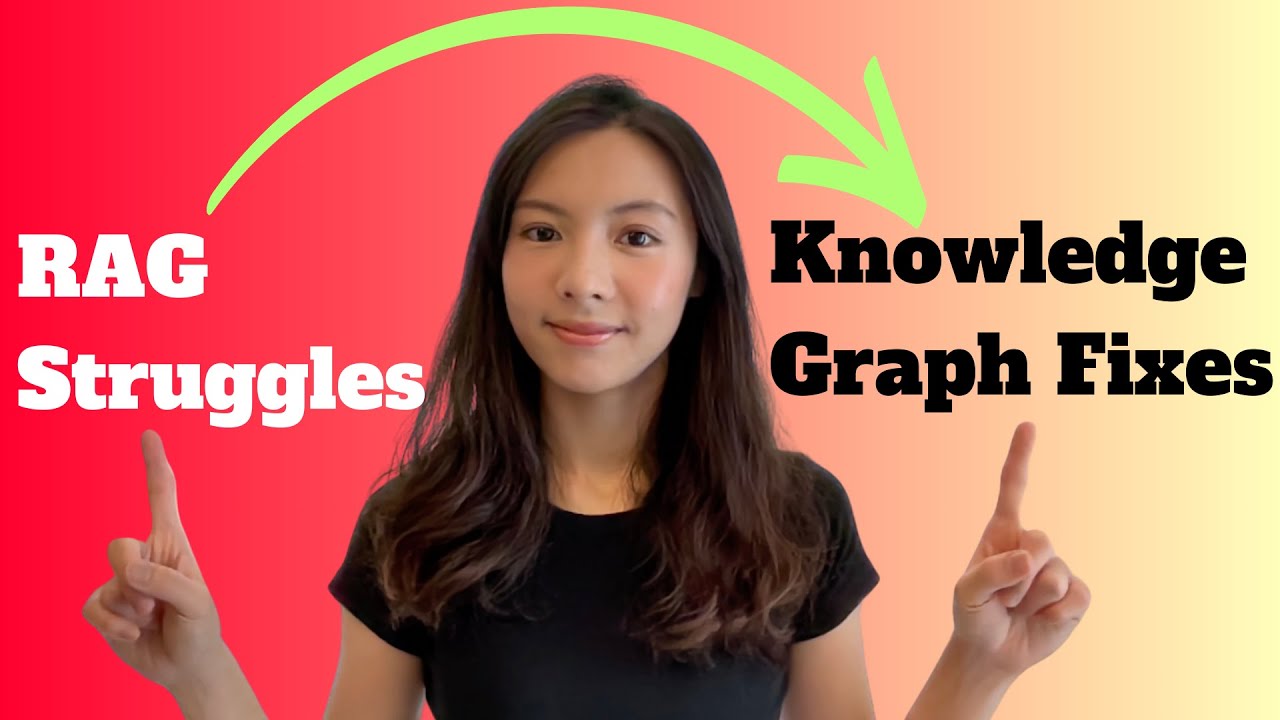
Показать описание
Long-Context models such as Google Gemini Pro 1.5 or Large World Model are probably changing the way we think about RAG (retrieval-augmented generation). Some are starting to explore the potential application of “Long-Context RAG”. One example is RAPTOR (Recursive Abstractive Processing for Tree-Organized Retrieval), by clustering and summarizing documents, this method lets language models grasp both general concepts and granular details in the individual documents. Inspired by LangChain, we tested out constructing a tree-based long context RAG. Watch the video to find out whether this approach allows us to say goodbye to the lost-in-the-middle effect commonly seen in large language models. AND, most importantly, how knowledge graphs can come to the rescue to enhance the answer quality. 😉
0:00 Coming Up
0:30 Intro
1:41 What is RAPTOR?
2:35 Knowledge base for RAG
3:13 Constructing a Knowledge Graph with Diffbot API for RAG
3:59 Construct tree-based RAG with RAPTOR
4:22 Test questions on tree-based RAG!
6:45 Reference our knowledge graph for answer enhancement
7:11 Are answers enhanced by our knowledge graph?
8:25 What are your takeaways?
Want to turn your unstructured text data into knowledge graphs?
0:00 Coming Up
0:30 Intro
1:41 What is RAPTOR?
2:35 Knowledge base for RAG
3:13 Constructing a Knowledge Graph with Diffbot API for RAG
3:59 Construct tree-based RAG with RAPTOR
4:22 Test questions on tree-based RAG!
6:45 Reference our knowledge graph for answer enhancement
7:11 Are answers enhanced by our knowledge graph?
8:25 What are your takeaways?
Want to turn your unstructured text data into knowledge graphs?
Комментарии