filmov
tv
Ali Ghodsi, Lec 19: PAC Learning
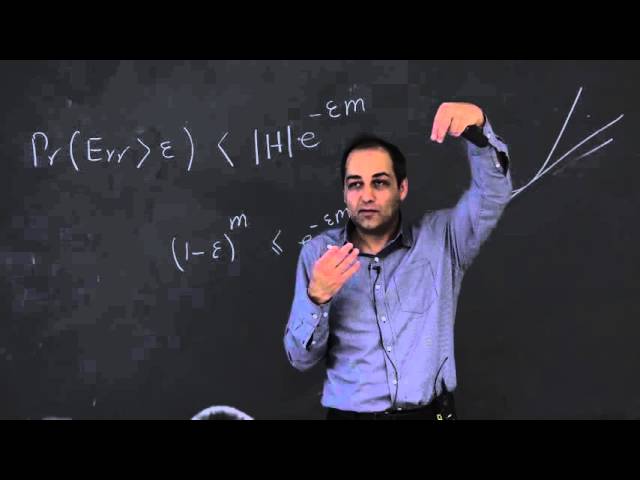
Показать описание
Description
Ali Ghodsi, Lec 19: PAC Learning
Ali Ghodsi, Lec 16 : Ali Ghodsi-Boosting
Ali Ghodsi, Lec 6: Logistic Regression, Perceptron
Ali Ghodsi, Lec [5.1]: Deep Learning, Recurrent neural network
Ali Ghodsi, Lec 15: t-SNE
Ali Ghodsi, Lec 13: Dual PCA, Supervised PCA
Ali Ghodsi, Lec 7: Backpropagation
Ali Ghodsi, Lec 1: Principal Component Analysis
Ali Ghodsi, Lec 17: Bagging, Convolutional Networks (part 1)
Ali Ghodsi, Lec 18: Convolutional neural network (Part 2)
Lecture 19 (EECS4404E) - PAC Learning
Ali Ghodsi, Lec [3,1]: Deep Learning, Word2vec
Ali Ghodsi Lec5 Model selection, Neural Networks
Ali Ghodsi, Lec [1,1]: Deep Learning, Introduction
Ali Ghodsi, Lec [7], Deep Learning , Restricted Boltzmann Machines (RBMs)
Ali Ghodsi, Lec [2,2]: Deep Learning, Regularization
AliGhodsi Lec 18, Bagging
Ali Ghodsi, Lec 9: Stein’s unbiased risk estimator (sure)
Agnostic PAC-Learning | Lê Nguyên Hoang
Ali Ghodsi, Lec [2,1]: Deep Learning, Regularization
Ali Ghodsi, Lec10: Weight decay
Lecture 25 - P.A.C Learning - Part I - 2019
PAC Learning and VC Dimension
Ali Ghodsi, Lec 15: Decision Tree, KNN
Комментарии